smCompactor: a workload-aware fine-grained resource management framework for GPGPUs
Symposium on Applied Computing(2021)
摘要
ABSTRACTRecently, graphic processing unit (GPU) multitasking has become important in many platforms since an efficient GPU multitasking mechanism can enable more GPU-enabled tasks running on limited physical GPUs. However, current GPU multitasking technologies, such as NVIDIA Multi-Process Service (MPS) and Hyper-Q may not fully utilize GPU resources since they do not consider the efficient use of intra-GPU resources. In this paper, we present smCompactor, which is a fine-grained GPU multitasking framework to fully exploit intra-GPU resources for different workloads. smCompactor dispatches any particular thread blocks (TBs) of different GPU kernels to appropriate stream multiprocessors (SMs) based on our profiled results of workloads. With smCompactor, GPU resource utilization can be improved as we can run more workloads on a single GPU while their performance is maintained. The evaluation results show that smCompactor improves resource utilization in terms of the number of active SMs by up to 33% and it reduces the kernel execution time by up to 26% compared with NVIDIA MPS.
更多查看译文
AI 理解论文
溯源树
样例
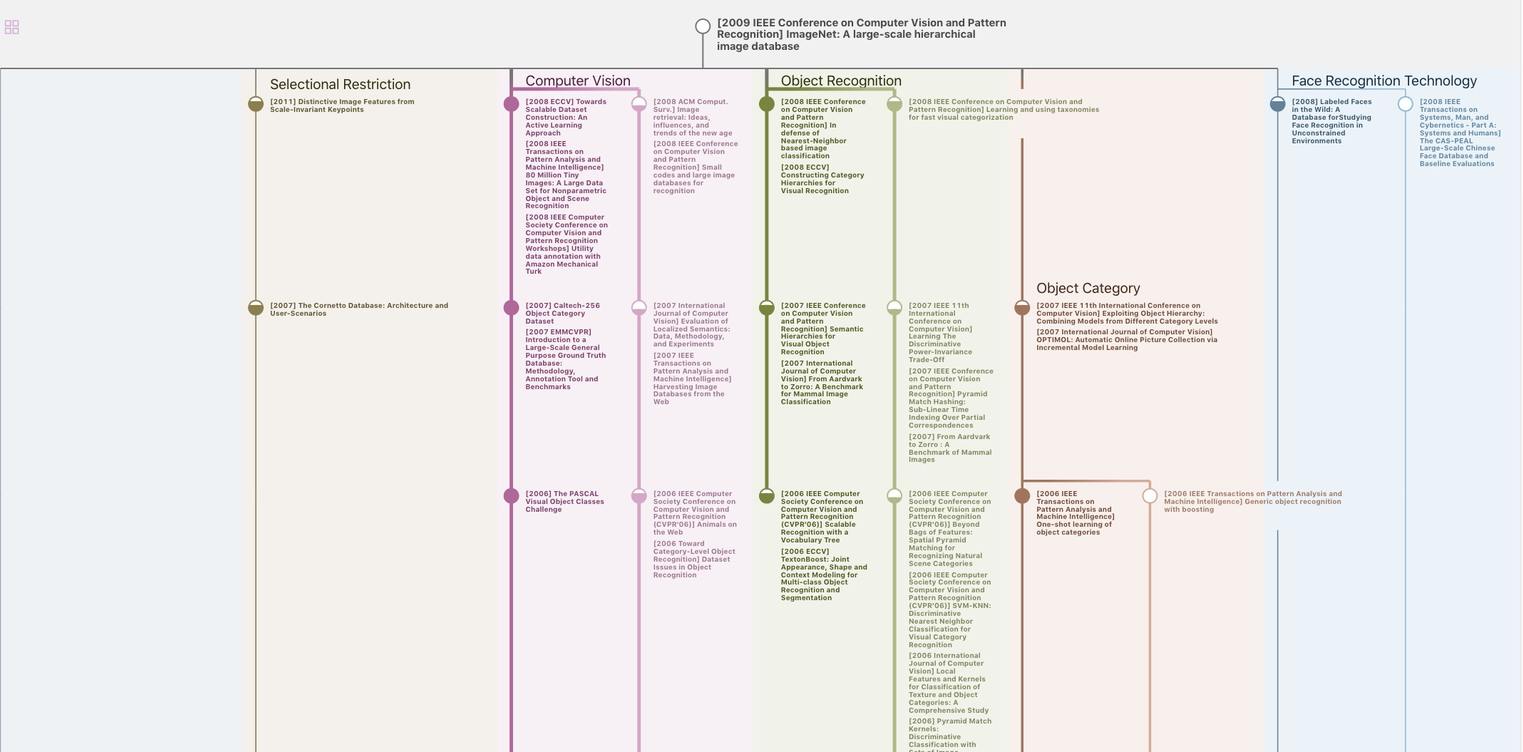
生成溯源树,研究论文发展脉络
Chat Paper
正在生成论文摘要