End-to-End One-Shot Human Parsing
IEEE TRANSACTIONS ON PATTERN ANALYSIS AND MACHINE INTELLIGENCE(2023)
摘要
Previous human parsing methods are limited to parsing humans into pre-defined classes, which is inflexible for practical fashion applications that often have new fashion item classes. In this paper, we define a novel one-shot human parsing (OSHP) task that requires parsing humans into an open set of classes defined by any test example. During training, only base classes are exposed, which only overlap with part of the test-time classes. To address three main challenges in OSHP, i.e., small sizes, testing bias, and similar parts, we devise an End-to-end One-shot human Parsing Network (EOPNet). Firstly, an end-to-end human parsing framework is proposed to parse the query image into both coarse-grained and fine-grained human classes, which embeds rich semantic information that is shared across different granularities to identify the small-sized human classes. Then, we gradually smooth the training-time static prototypes to get robust class representations. Moreover, we employ a dynamic objective to encourage the network enhancing features' representational capability in the early training phase while improving features' transferability in the late training phase. Therefore, our method can quickly adapt to the novel classes and mitigate the testing bias issue. In addition, we add a contrastive loss at the prototype level to enforce inter-class distances, thereby discriminating the similar parts. For comprehensive evaluations on the newtask, we tailor three existing popularhumanparsing benchmarks to the OSHP task. Experiments demonstrate that EOP-Net outperforms representative one-shot segmentation models by large margins and serves as a strong baseline for further research.
更多查看译文
关键词
Benchmark,contrastive learning,end-to-end model,human parsing,one-shot semantic segmentation
AI 理解论文
溯源树
样例
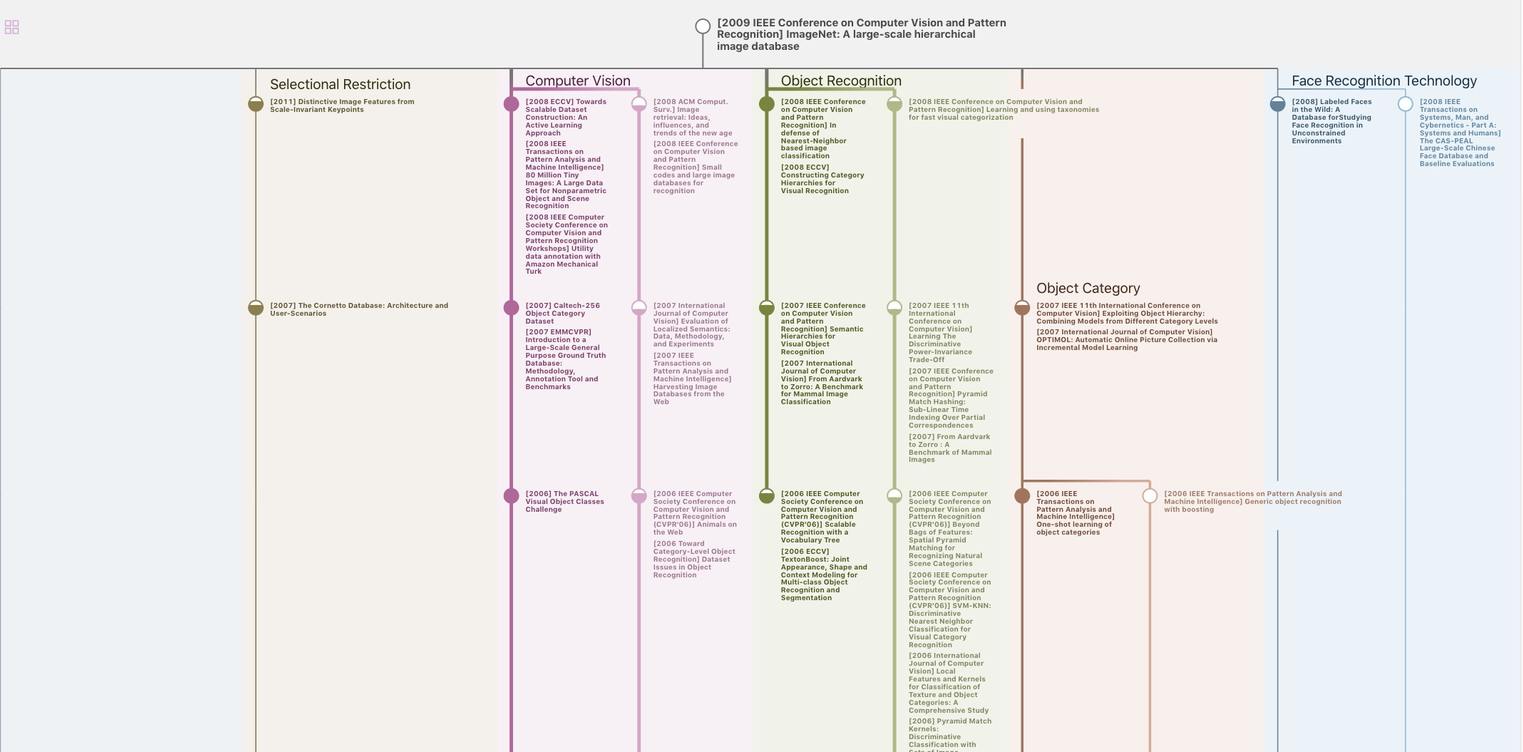
生成溯源树,研究论文发展脉络
Chat Paper
正在生成论文摘要