Synthetic Data for Model Selection
arxiv(2021)
摘要
Recent improvements in synthetic data generation make it possible to produce images that are highly photorealistic and indistinguishable from real ones. Furthermore, synthetic generation pipelines have the potential to generate an unlimited number of images. The combination of high photorealism and scale turn the synthetic data into a promising candidate for potentially improving various machine learning (ML) pipelines. Thus far, a large body of research in this field has focused on using synthetic images for training, by augmenting and enlarging training data. In contrast to using synthetic data for training, in this work we explore whether synthetic data can be beneficial for model selection. Considering the task of image classification, we demonstrate that when data is scarce, synthetic data can be used to replace the held out validation set, thus allowing to train on a larger dataset.
更多查看译文
关键词
model selection,synthetic data
AI 理解论文
溯源树
样例
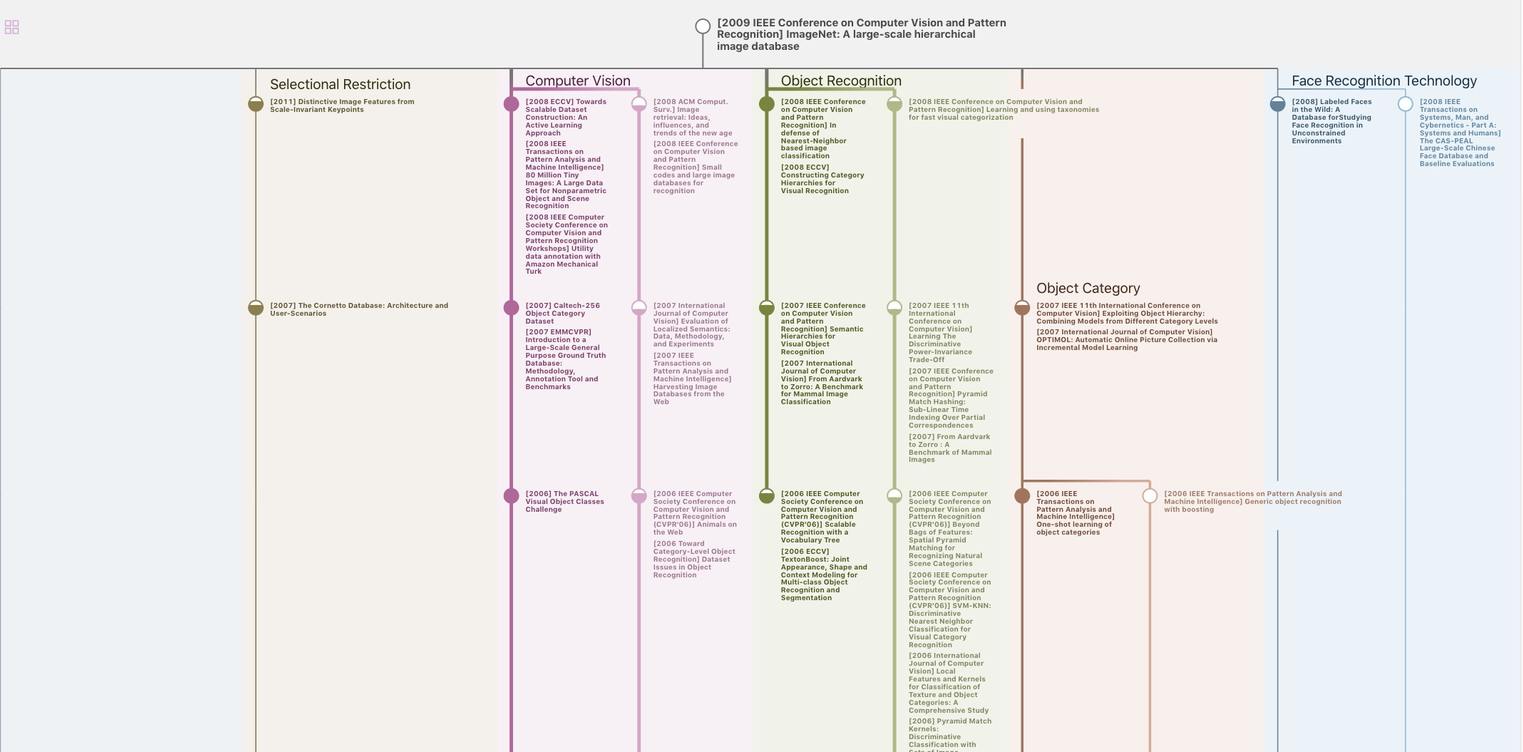
生成溯源树,研究论文发展脉络
Chat Paper
正在生成论文摘要