Bidirectional Mapping Of Brain Mri And Pet With 3d Reversible Gan For The Diagnosis Of Alzheimer'S Disease
FRONTIERS IN NEUROSCIENCE(2021)
摘要
Combining multi-modality data for brain disease diagnosis such as Alzheimer's disease (AD) commonly leads to improved performance than those using a single modality. However, it is still challenging to train a multi-modality model since it is difficult in clinical practice to obtain complete data that includes all modality data. Generally speaking, it is difficult to obtain both magnetic resonance images (MRI) and positron emission tomography (PET) images of a single patient. PET is expensive and requires the injection of radioactive substances into the patient's body, while MR images are cheaper, safer, and more widely used in practice. Discarding samples without PET data is a common method in previous studies, but the reduction in the number of samples will result in a decrease in model performance. To take advantage of multi-modal complementary information, we first adopt the Reversible Generative Adversarial Network (RevGAN) model to reconstruct the missing data. After that, a 3D convolutional neural network (CNN) classification model with multi-modality input was proposed to perform AD diagnosis. We have evaluated our method on the Alzheimer's Disease Neuroimaging Initiative (ADNI) database, and compared the performance of the proposed method with those using state-of-the-art methods. The experimental results show that the structural and functional information of brain tissue can be mapped well and that the image synthesized by our method is close to the real image. In addition, the use of synthetic data is beneficial for the diagnosis and prediction of Alzheimer's disease, demonstrating the effectiveness of the proposed framework.
更多查看译文
关键词
Alzheimer’, s disease, multi-modality, image synthesis, 3D CNN, reversible GAN
AI 理解论文
溯源树
样例
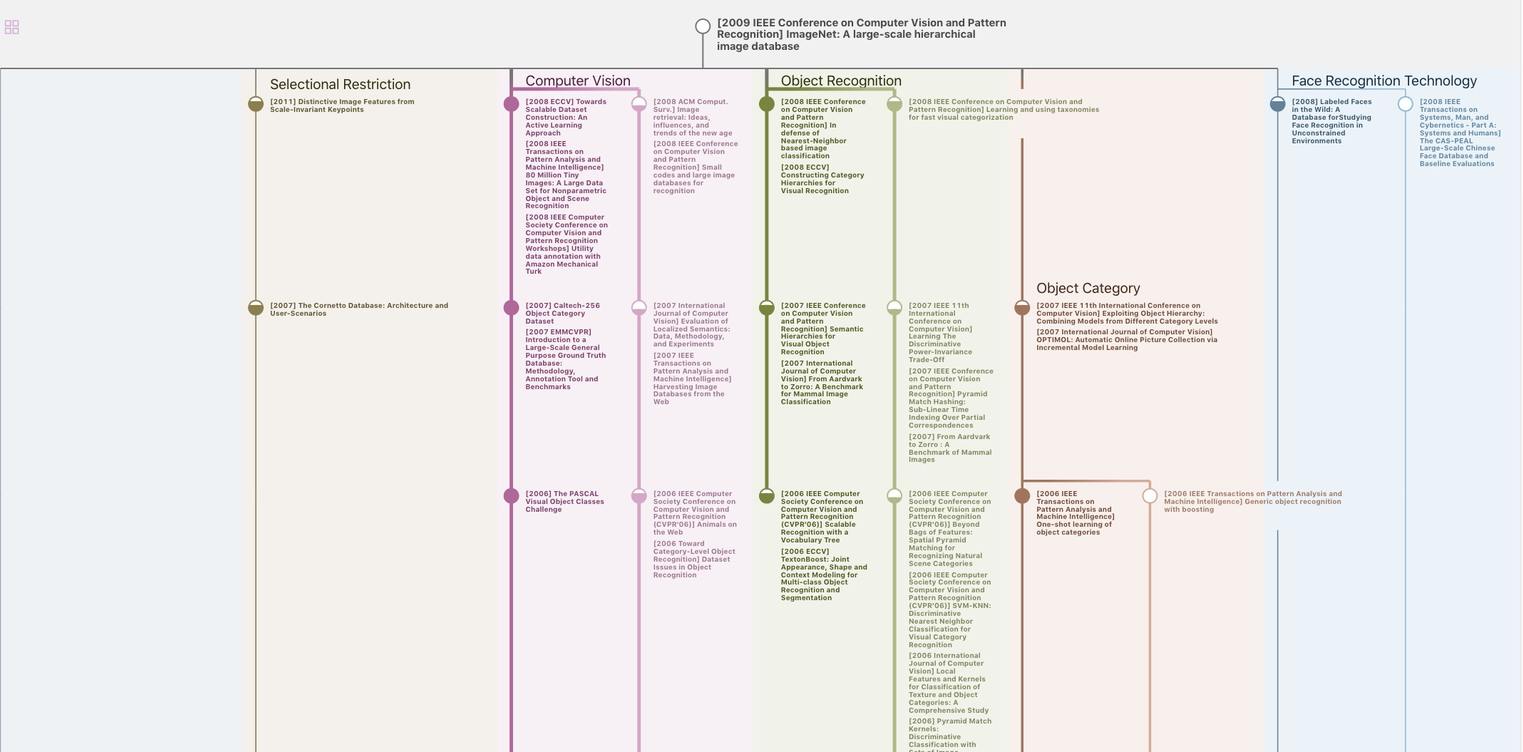
生成溯源树,研究论文发展脉络
Chat Paper
正在生成论文摘要