Unsupervised Neural Machine Translation for Similar and Distant Language Pairs: An Empirical Study
ACM Transactions on Asian and Low-Resource Language Information Processing(2021)
摘要
AbstractUnsupervised neural machine translation (UNMT) has achieved remarkable results for several language pairs, such as French–English and German–English. Most previous studies have focused on modeling UNMT systems; few studies have investigated the effect of UNMT on specific languages. In this article, we first empirically investigate UNMT for four diverse language pairs (French/German/Chinese/Japanese–English). We confirm that the performance of UNMT in translation tasks for similar language pairs (French/German–English) is dramatically better than for distant language pairs (Chinese/Japanese–English). We empirically show that the lack of shared words and different word orderings are the main reasons that lead UNMT to underperform in Chinese/Japanese–English. Based on these findings, we propose several methods, including artificial shared words and pre-ordering, to improve the performance of UNMT for distant language pairs. Moreover, we propose a simple general method to improve translation performance for all these four language pairs. The existing UNMT model can generate a translation of a reasonable quality after a few training epochs owing to a denoising mechanism and shared latent representations. However, learning shared latent representations restricts the performance of translation in both directions, particularly for distant language pairs, while denoising dramatically delays convergence by continuously modifying the training data. To avoid these problems, we propose a simple, yet effective and efficient, approach that (like UNMT) relies solely on monolingual corpora: pseudo-data-based unsupervised neural machine translation. Experimental results for these four language pairs show that our proposed methods significantly outperform UNMT baselines.
更多查看译文
关键词
Unsupervised neural machine translation, pseudo-data-based unsupervised neural machine translation, similar and distant language pairs
AI 理解论文
溯源树
样例
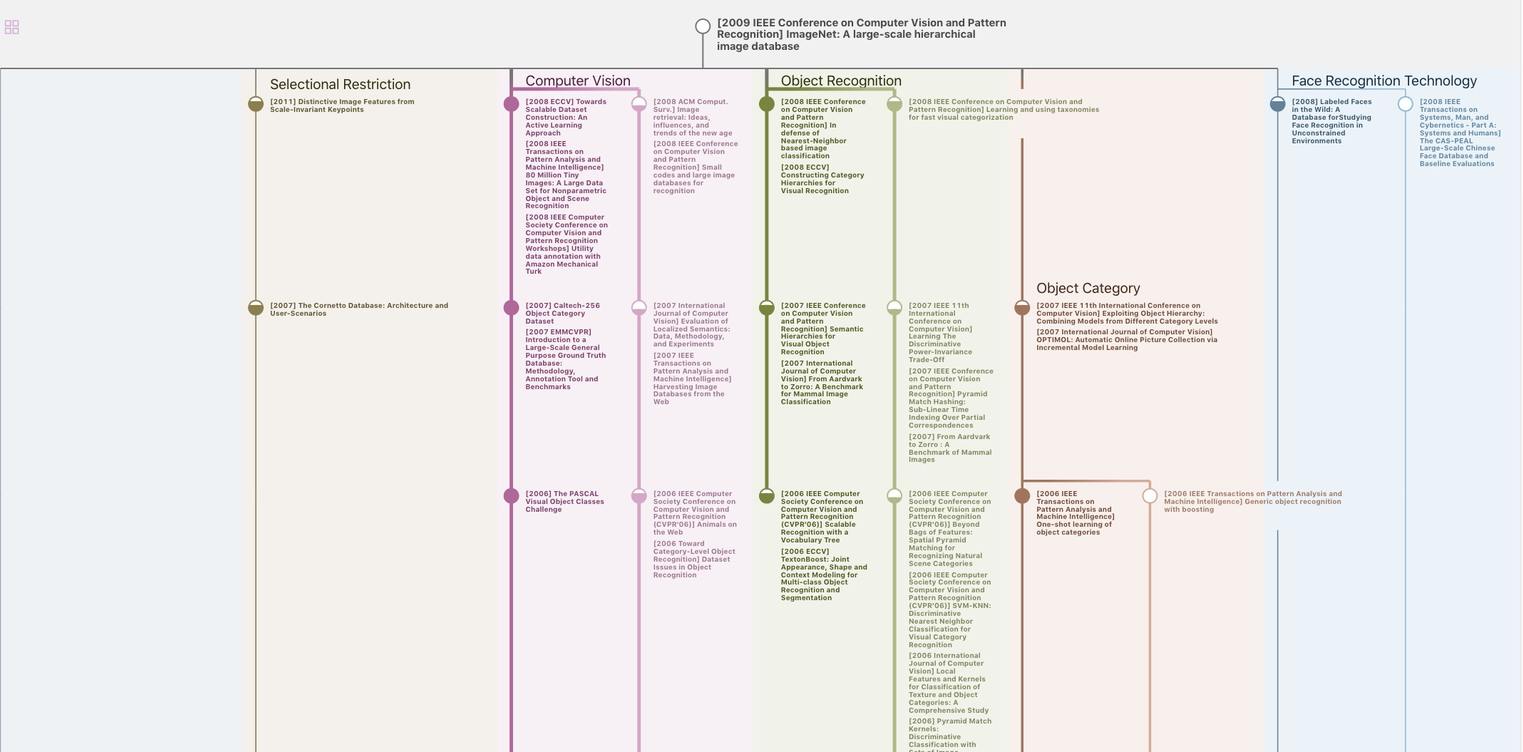
生成溯源树,研究论文发展脉络
Chat Paper
正在生成论文摘要