Breadcrumbs: Adversarial Class-Balanced Sampling for Long-Tailed Recognition
Computer Vision – ECCV 2022(2022)
摘要
The problem of long-tailed recognition, where the number of examples per class is highly unbalanced, is considered. While training with class-balanced sampling has been shown effective for this problem, it is known to over-fit to few-shot classes. It is hypothesized that this is due to the repeated sampling of examples and can be addressed by feature space augmentation. A new feature augmentation strategy, EMANATE, based on back-tracking of features across epochs during training, is proposed. It is shown that, unlike class-balanced sampling, this is an adversarial augmentation strategy. A new sampling procedure, Breadcrumb, is then introduced to implement adversarial class-balanced sampling without extra computation. Experiments on three popular long-tailed recognition datasets show that Breadcrumb training produces classifiers that outperform existing solutions to the problem. Code:
https://github.com/BoLiu-SVCL/Breadcrumbs
.
更多查看译文
关键词
sampling,recognition,class-balanced,long-tailed
AI 理解论文
溯源树
样例
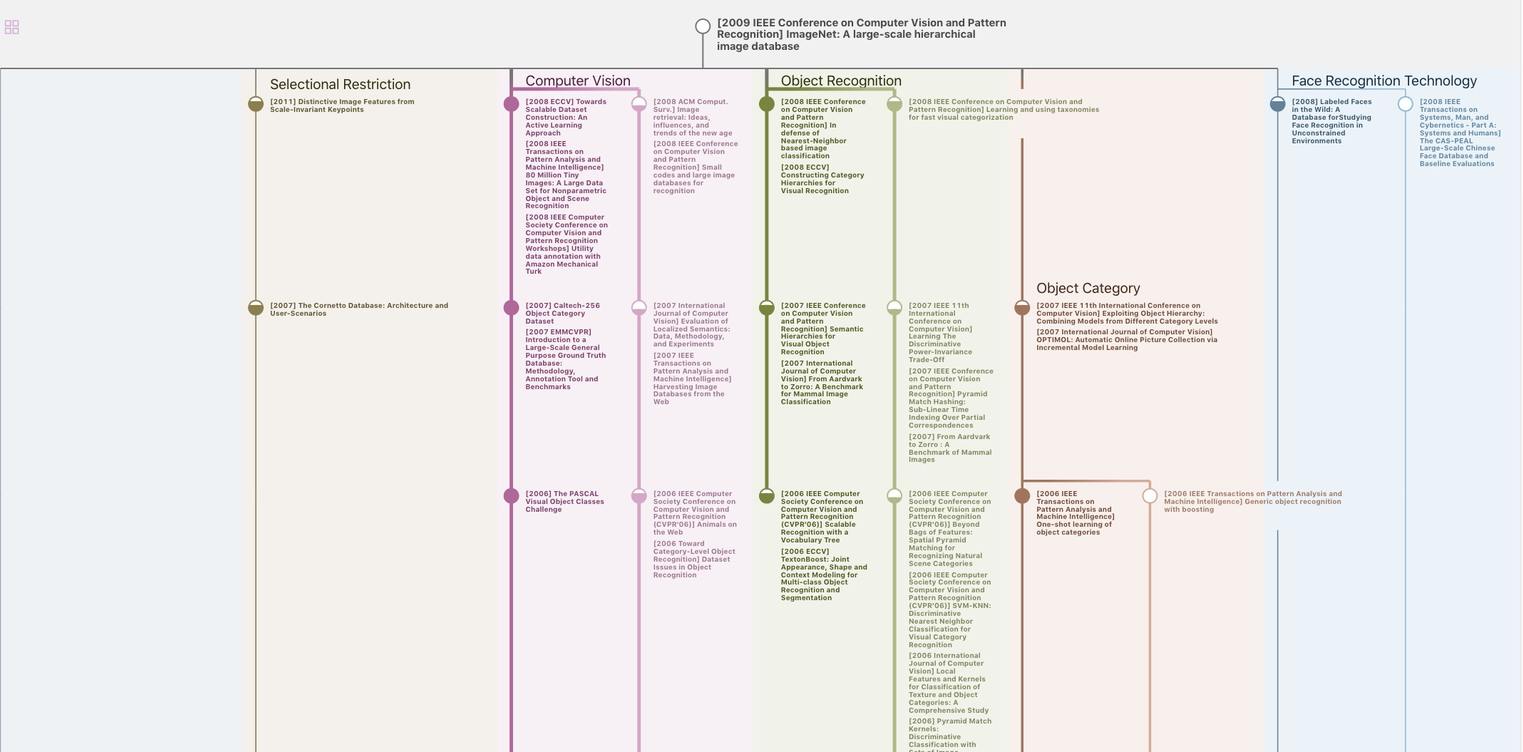
生成溯源树,研究论文发展脉络
Chat Paper
正在生成论文摘要