Linear convergence of the subspace constrained mean shift algorithm: from Euclidean to directional data.
Information and inference : a journal of the IMA(2023)
摘要
This paper studies the linear convergence of the subspace constrained mean shift (SCMS) algorithm, a well-known algorithm for identifying a density ridge defined by a kernel density estimator. By arguing that the SCMS algorithm is a special variant of a subspace constrained gradient ascent (SCGA) algorithm with an adaptive step size, we derive the linear convergence of such SCGA algorithm. While the existing research focuses mainly on density ridges in the Euclidean space, we generalize density ridges and the SCMS algorithm to directional data. In particular, we establish the stability theorem of density ridges with directional data and prove the linear convergence of our proposed directional SCMS algorithm.
更多查看译文
关键词
49Q12,62G05,62H11,directional data,optimization on a manifold,ridges,subspace constrained mean shift
AI 理解论文
溯源树
样例
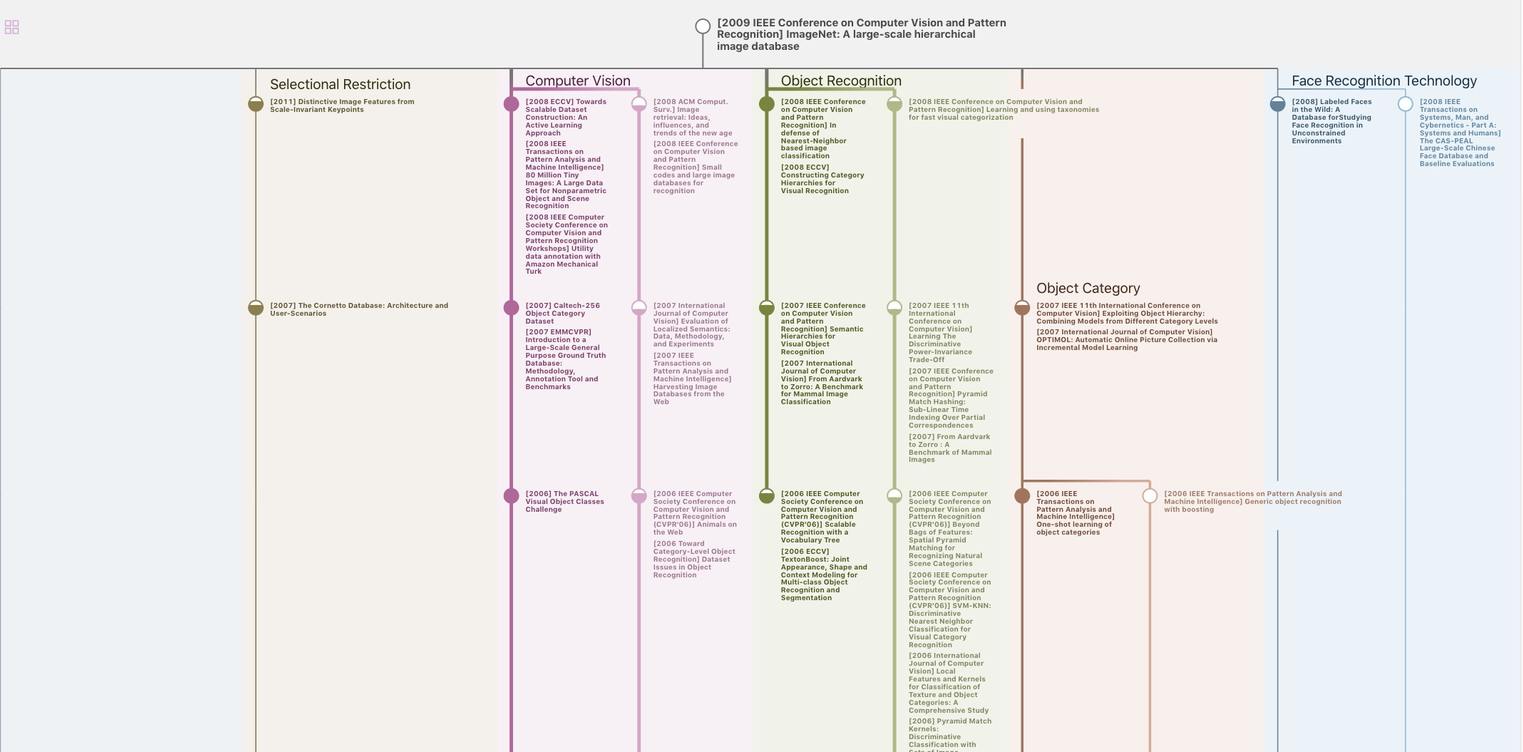
生成溯源树,研究论文发展脉络
Chat Paper
正在生成论文摘要