Prediction Of Incident Cancers In The Lifelines Population-Based Cohort
CANCERS(2021)
摘要
Simple SummaryThe accurate prediction of incident cancers could be relevant to understanding and reducing cancer incidence. The aim of this study was to develop machine learning (ML) models that could predict an incident diagnosis of cancer. Data were available for 116,188 cancer-free participants and 4232 incident cancer cases. The main outcome was an incident cancer (excluding skin cancer) during follow-up assessment in a population-based cohort. The performance of three ML algorithms was evaluated using supervised binary classification to identify incident cancers among participants. An overall area under the receiver operator curve (AUC) < 0.75 was obtained; the highest AUC was for prostate cancer AUC > 0.80. Linear and non-linear ML algorithms including socioeconomic, lifestyle, and clinical variables produced a moderate predictive performance of incident cancers in the Lifelines cohort.Cancer incidence is rising, and accurate prediction of incident cancers could be relevant to understanding and reducing cancer incidence. The aim of this study was to develop machine learning (ML) models that could predict an incident diagnosis of cancer. Participants without any history of cancer within the Lifelines population-based cohort were followed for a median of 7 years. Data were available for 116,188 cancer-free participants and 4232 incident cancer cases. At baseline, socioeconomic, lifestyle, and clinical variables were assessed. The main outcome was an incident cancer during follow-up (excluding skin cancer), based on linkage with the national pathology registry. The performance of three ML algorithms was evaluated using supervised binary classification to identify incident cancers among participants. Elastic net regularization and Gini index were used for variables selection. An overall area under the receiver operator curve (AUC) <0.75 was obtained, the highest AUC value was for prostate cancer (random forest AUC = 0.82 (95% CI 0.77-0.87), logistic regression AUC = 0.81 (95% CI 0.76-0.86), and support vector machines AUC = 0.83 (95% CI 0.78-0.88), respectively); age was the most important predictor in these models. Linear and non-linear ML algorithms including socioeconomic, lifestyle, and clinical variables produced a moderate predictive performance of incident cancers in the Lifelines cohort.
更多查看译文
关键词
classification, prediction, neoplasms, supervised machine learning, health behavior, lifestyle
AI 理解论文
溯源树
样例
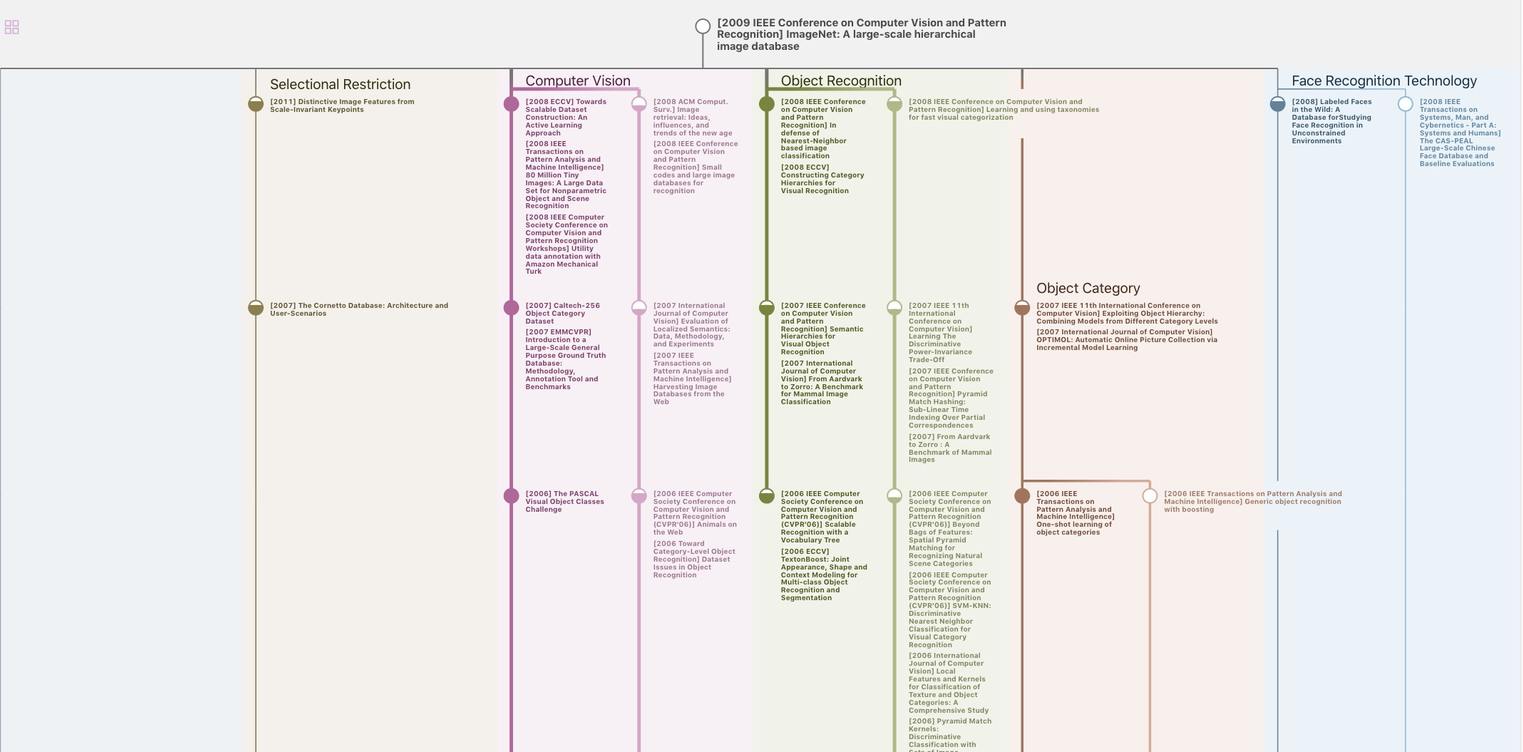
生成溯源树,研究论文发展脉络
Chat Paper
正在生成论文摘要