Boosting Multilabel Semantic Segmentation for Somata and Vessels in Mouse Brain.
Frontiers in neuroscience(2021)
摘要
Deep convolutional neural networks (DCNNs) are widely utilized for the semantic segmentation of dense nerve tissues from light and electron microscopy (EM) image data; the goal of this technique is to achieve efficient and accurate three-dimensional reconstruction of the vasculature and neural networks in the brain. The success of these tasks heavily depends on the amount, and especially the quality, of the human-annotated labels fed into DCNNs. However, it is often difficult to acquire the gold standard of human-annotated labels for dense nerve tissues; human annotations inevitably contain discrepancies or even errors, which substantially impact the performance of DCNNs. Thus, a novel boosting framework consisting of a DCNN for multilabel semantic segmentation with a customized Dice-logarithmic loss function, a fusion module combining the annotated labels and the corresponding predictions from the DCNN, and a boosting algorithm to sequentially update the sample weights during network training iterations was proposed to systematically improve the quality of the annotated labels; this framework eventually resulted in improved segmentation task performance. The microoptical sectioning tomography (MOST) dataset was then employed to assess the effectiveness of the proposed framework. The result indicated that the framework, even trained with a dataset including some poor-quality human-annotated labels, achieved state-of-the-art performance in the segmentation of somata and vessels in the mouse brain. Thus, the proposed technique of artificial intelligence could advance neuroscience research.
更多查看译文
AI 理解论文
溯源树
样例
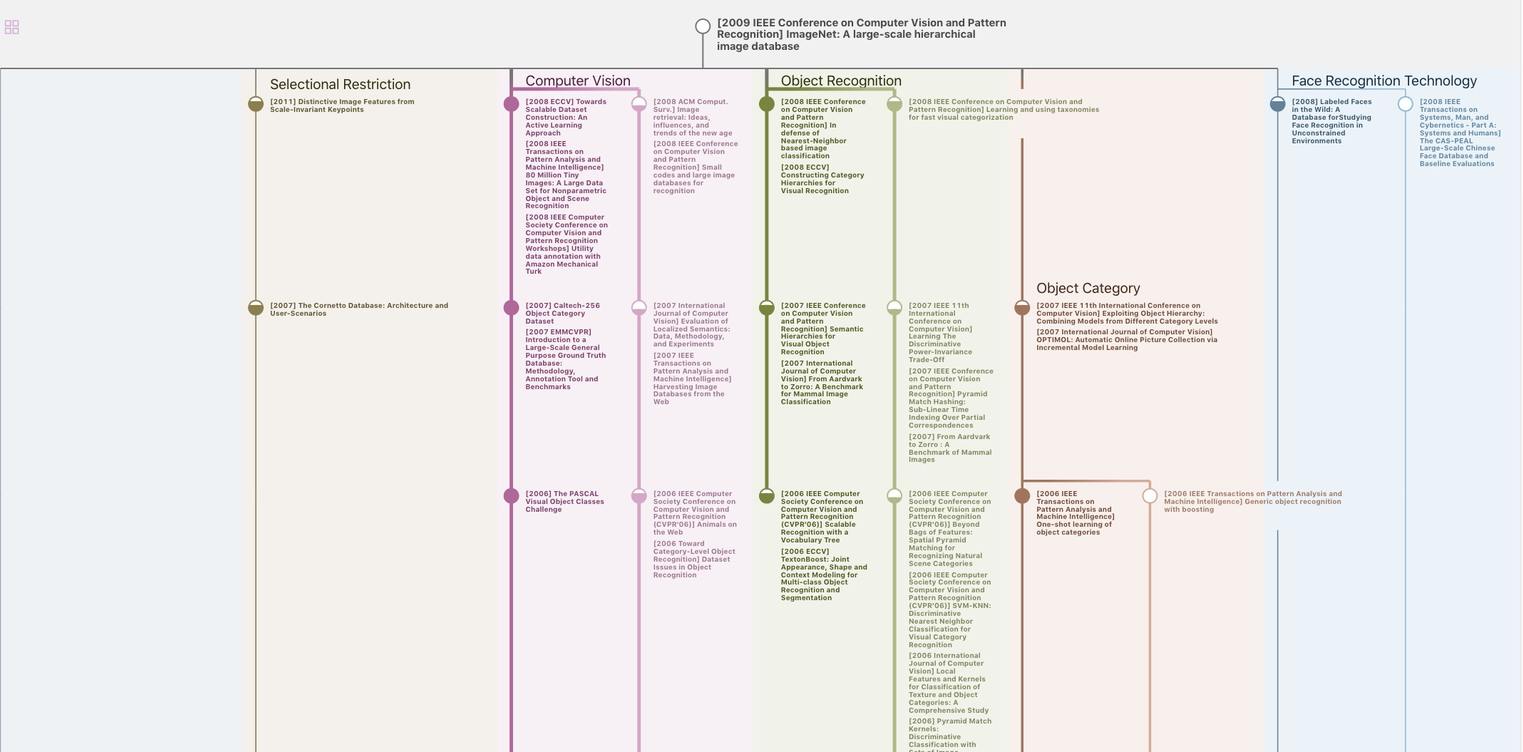
生成溯源树,研究论文发展脉络
Chat Paper
正在生成论文摘要