MeerCRAB: MeerLICHT classification of real and bogus transients using deep learning
EXPERIMENTAL ASTRONOMY(2021)
摘要
Astronomers require efficient automated detection and classification pipelines when conducting large-scale surveys of the (optical) sky for variable and transient sources. Such pipelines are fundamentally important, as they permit rapid follow-up and analysis of those detections most likely to be of scientific value. We therefore present a deep learning pipeline based on the convolutional neural network architecture called MeerCRAB. It is designed to filter out the so called “bogus” detections from true astrophysical sources in the transient detection pipeline of the MeerLICHT telescope. Optical candidates are described using a variety of 2D images and numerical features extracted from those images. The relationship between the input images and the target classes is unclear, since the ground truth is poorly defined and often the subject of debate. This makes it difficult to determine which source of information should be used to train a classification algorithm. We therefore used two methods for labelling our data (i) thresholding and (ii) latent class model approaches. We deployed variants of MeerCRAB that employed different network architectures trained using different combinations of input images and training set choices, based on classification labels provided by volunteers. The deepest network worked best with an accuracy of 99.5% and Matthews correlation coefficient (MCC) value of 0.989. The best model was integrated to the MeerLICHT transient vetting pipeline, enabling the accurate and efficient classification of detected transients that allows researchers to select the most promising candidates for their research goals.
更多查看译文
关键词
Methods, Data analysis, Methods, Deep learning, Techniques, Image processing, Surveys, Stars, General, Transients, Real, Bogus
AI 理解论文
溯源树
样例
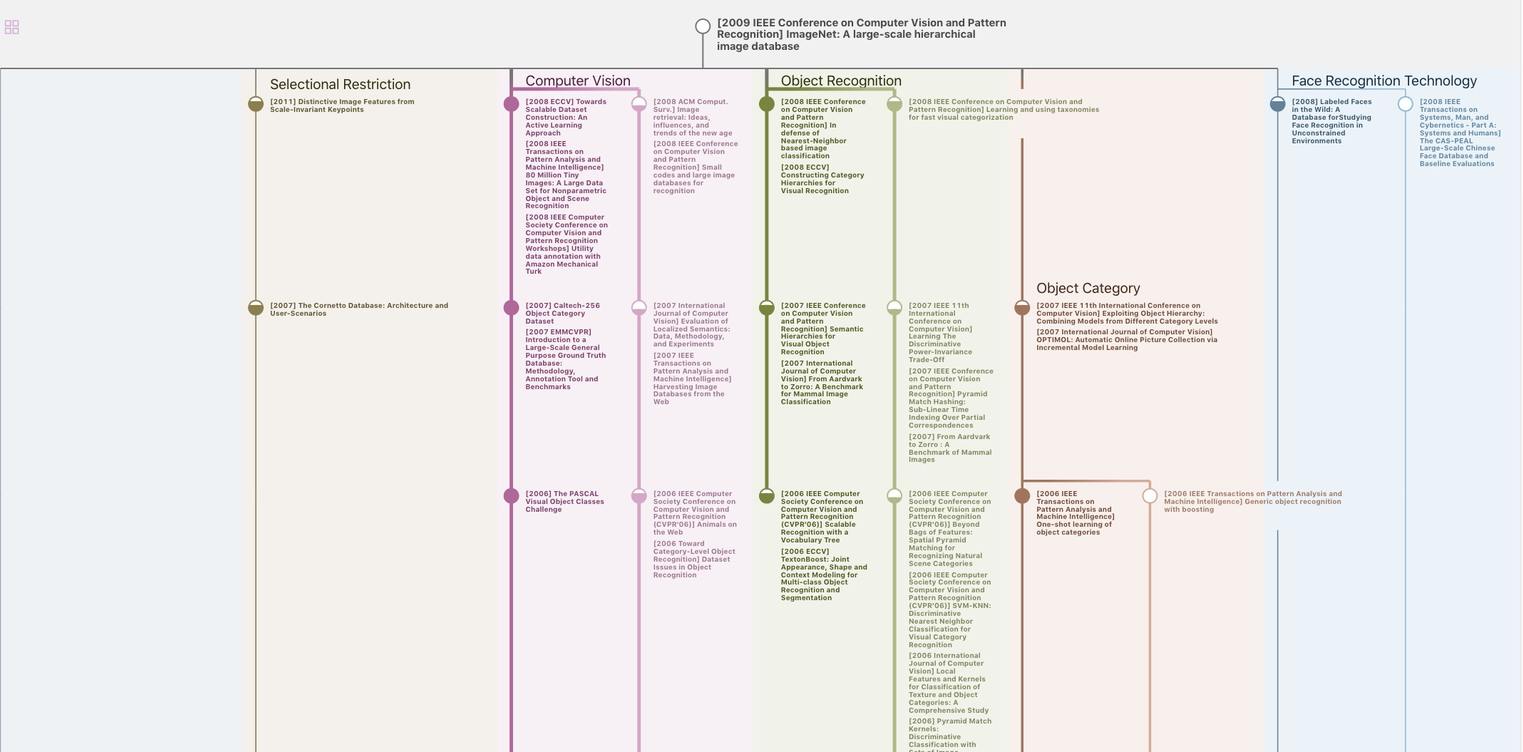
生成溯源树,研究论文发展脉络
Chat Paper
正在生成论文摘要