Recent Advances on Non-Line-of-Sight Imaging: Conventional Physical Models, Deep Learning, and New Scenes
ArXiv(2021)
摘要
As an emerging technology that has attracted huge attention, non-line-of-sight (NLOS) imaging can reconstruct hidden objects by analyzing the diffuse reflection on a relay surface, with broad application prospects in the fields of autonomous driving, medical imaging, and defense. Despite the challenges of low signal-to-noise ratio (SNR) and high ill-posedness, NLOS imaging has been developed rapidly in recent years. Most current NLOS imaging technologies use conventional physical models, constructing imaging models through active or passive illumination and using reconstruction algorithms to restore hidden scenes. Moreover, deep learning algorithms for NLOS imaging have also received much attention recently. This paper presents a comprehensive overview of both conventional and deep learning-based NLOS imaging techniques. Besides, we also survey new proposed NLOS scenes, and discuss the challenges and prospects of existing technologies. Such a survey can help readers have an overview of different types of NLOS imaging, thus expediting the development of seeing around corners.
更多查看译文
关键词
Non-line-of-sight (NLOS), deep learning, active NLOS imaging, passive NLOS imaging
AI 理解论文
溯源树
样例
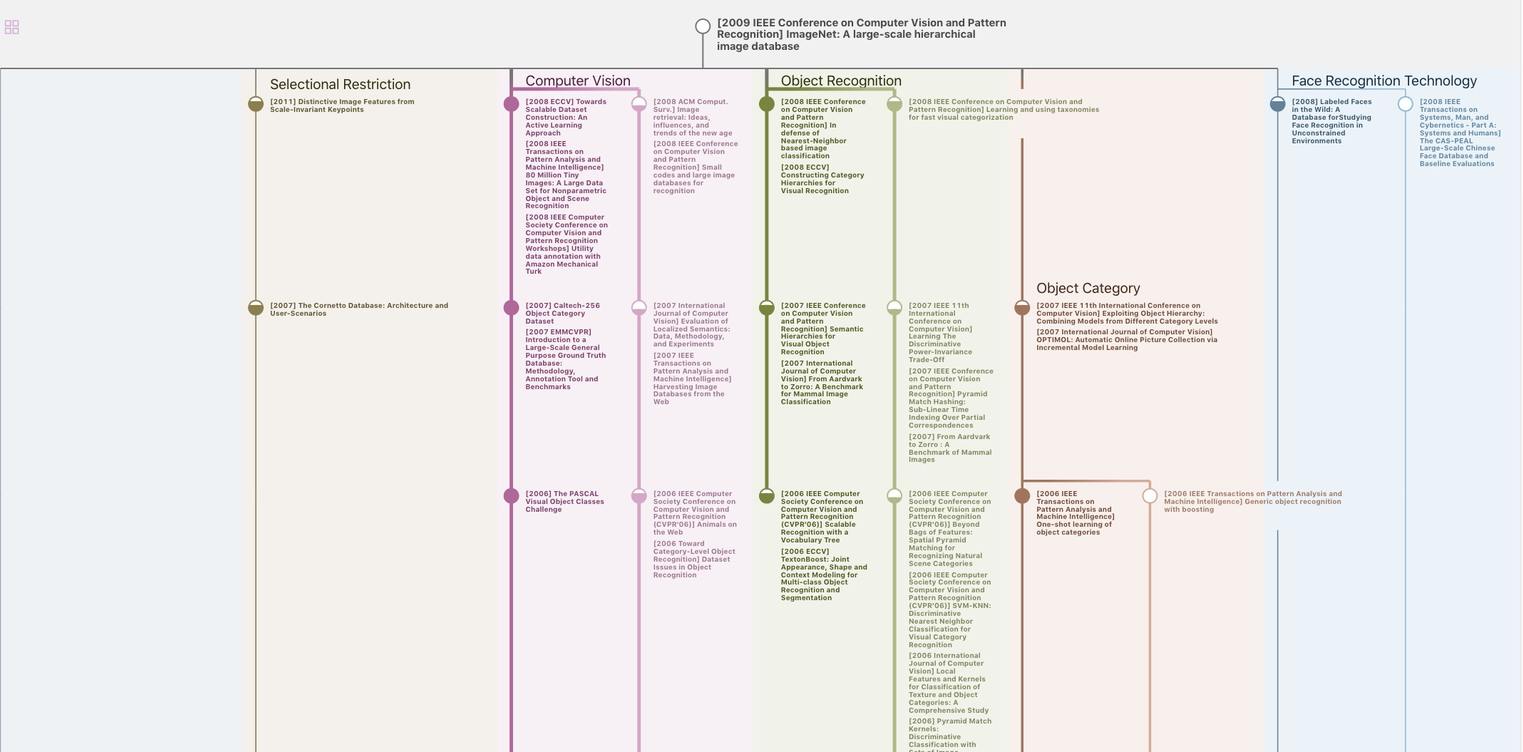
生成溯源树,研究论文发展脉络
Chat Paper
正在生成论文摘要