Boosting Co-teaching with Compression Regularization for Label Noise
2021 IEEE/CVF CONFERENCE ON COMPUTER VISION AND PATTERN RECOGITION WORKSHOPS (CVPRW 2021)(2021)
摘要
In this paper, we study the problem of learning image classification models in the presence of label noise. We revisit a simple compression regularization named Nested Dropout [22]. We find that Nested Dropout [22], though originally proposed to perform fast information retrieval and adaptive data compression, can properly regularize a neural network to combat label noise. Moreover, owing to its simplicity, it can be easily combined with Co-teaching [5] to further boost the performance.Our final model remains simple yet effective: it achieves comparable or even better performance than the state-of-the-art approaches on two real-world datasets with label noise which are Clothing1M [28] and ANIMAL-10N [24]. On Clothing1M [28], our approach obtains 74.9% accuracy which is slightly better than that of DivideMix [12]. On ANIMAL-10N [24], we achieve 84.1% accuracy while the best public result by PLC [30] is 83.4%. We hope that our simple approach can be served as a strong baseline for learning with label noise. Our implementation is available at https://github.com/yingyichen-cyy/Nested-Co-teaching.
更多查看译文
关键词
compression regularization,label,noise,co-teaching
AI 理解论文
溯源树
样例
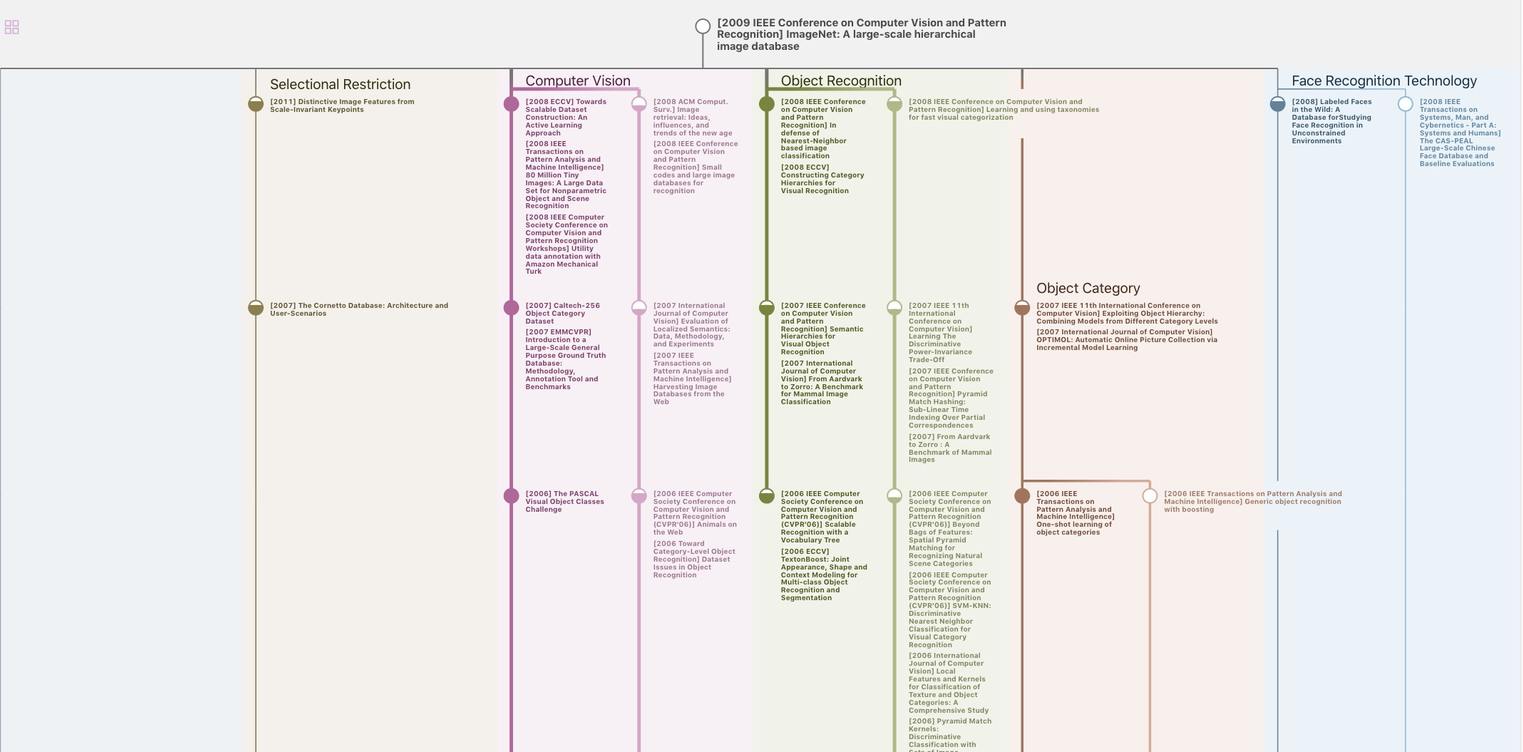
生成溯源树,研究论文发展脉络
Chat Paper
正在生成论文摘要