Distributional Gaussian Process Layers for Outlier Detection in Image Segmentation
IPMI(2021)
摘要
We propose a parameter efficient Bayesian layer for hierarchical convolutional Gaussian Processes that incorporates Gaussian Processes operating in Wasserstein-2 space to reliably propagate uncertainty. This directly replaces convolving Gaussian Processes with a distance-preserving affine operator on distributions. Our experiments on brain tissue-segmentation show that the resulting architecture approaches the performance of well-established deterministic segmentation algorithms (U-Net), which has never been achieved with previous hierarchical Gaussian Processes. Moreover, by applying the same segmentation model to out-of-distribution data (i.e., images with pathology such as brain tumors), we show that our uncertainty estimates result in out-of-distribution detection that outperforms the capabilities of previous Bayesian networks and reconstruction-based approaches that learn normative distributions.
更多查看译文
关键词
outlier detection,segmentation
AI 理解论文
溯源树
样例
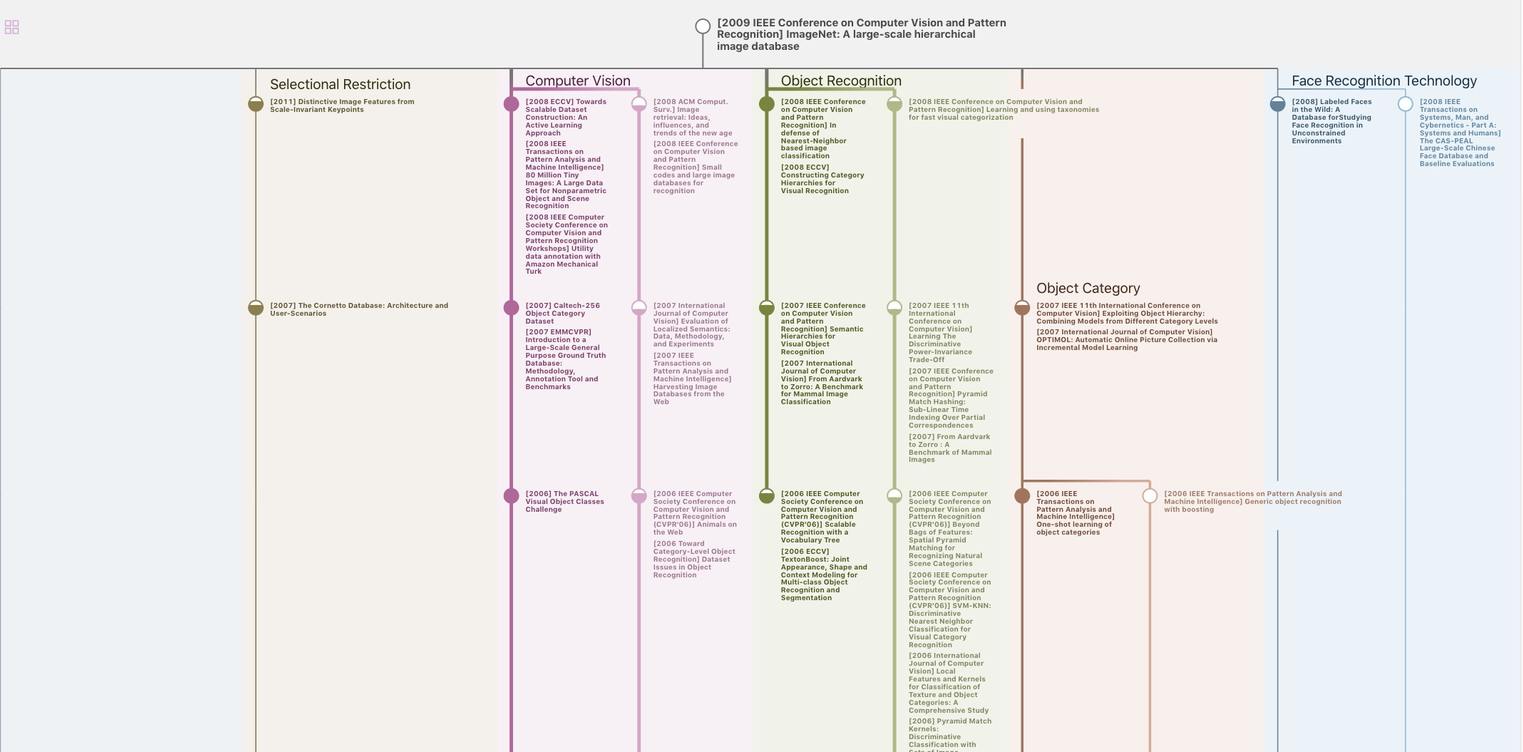
生成溯源树,研究论文发展脉络
Chat Paper
正在生成论文摘要