A Unifying Framework for Sparsity-Constrained Optimization
arxiv(2023)
摘要
In this paper, we consider the optimization problem of minimizing a continuously differentiable function subject to both convex constraints and sparsity constraints. By exploiting a mixed-integer reformulation from the literature, we define a necessary optimality condition based on a tailored neighborhood that allows to take into account potential changes of the support set. We then propose an algorithmic framework to tackle the considered class of problems and prove its convergence to points satisfying the newly introduced concept of stationarity. We further show that, by suitably choosing the neighborhood, other well-known optimality conditions from the literature can be recovered at the limit points of the sequence produced by the algorithm. Finally, we analyze the computational impact of the neighborhood size within our framework and in the comparison with some state-of-the-art algorithms, namely, the Penalty Decomposition method and the Greedy Sparse-Simplex method. The algorithms have been tested using a benchmark related to sparse logistic regression problems.
更多查看译文
关键词
Sparsity-constrained problems,Optimality conditions,Stationarity,Numerical methods,Asymptotic convergence,Sparse logistic regression
AI 理解论文
溯源树
样例
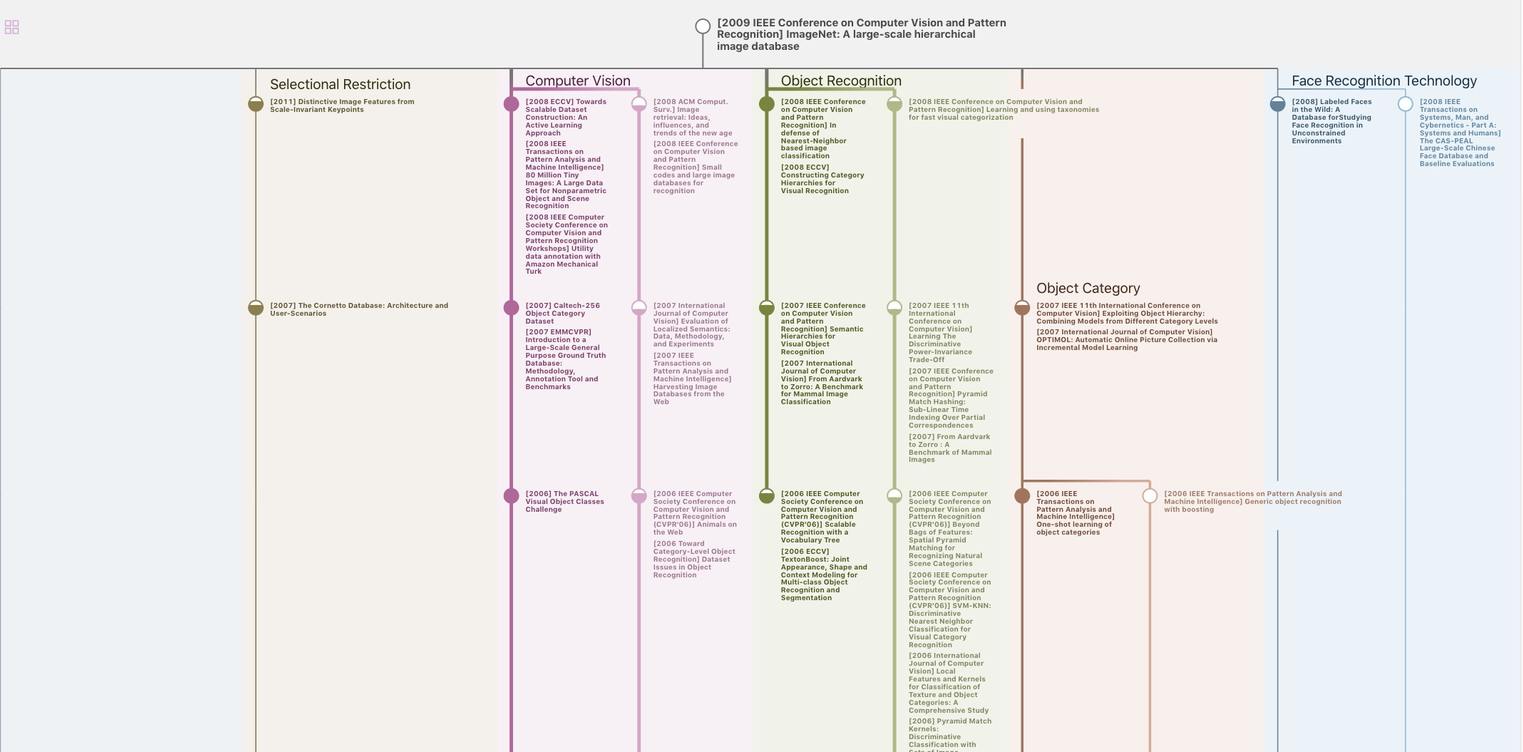
生成溯源树,研究论文发展脉络
Chat Paper
正在生成论文摘要