Dual Transformer for Point Cloud Analysis
arxiv(2023)
摘要
Feature representation learning is a key component in 3D point cloud analysis. However, the powerful convolutional neural networks (CNNs) cannot be applied due to the irregular structure of point clouds. Therefore, following the tremendous success of transformer in natural language processing and image understanding tasks, in this paper, we present a novel point cloud representation learning architecture, named Dual Transformer Network (DTNet), which mainly consists of Dual Point Cloud Transformer (DPCT) module. Specifically, by aggregating the well-designed point-wise and channel-wise self-attention models simultaneously, DPCT module can capture much richer contextual dependencies semantically from the perspective of position and channel. With the DPCT model as a fundamental component, we construct the DTNet for performing 3D point cloud analysis in an end-to-end manner. Extensive quantitative and qualitative experiments on publicly available benchmarks demonstrate the effectiveness of our transformer framework for the tasks of 3D point cloud classification, segmentation and visual object affordance understanding, achieving highly competitive performance in comparison with the state-of-the-art approaches.
更多查看译文
关键词
Classification,point cloud,segmentation,self attention,transformer,visual affordance understanding
AI 理解论文
溯源树
样例
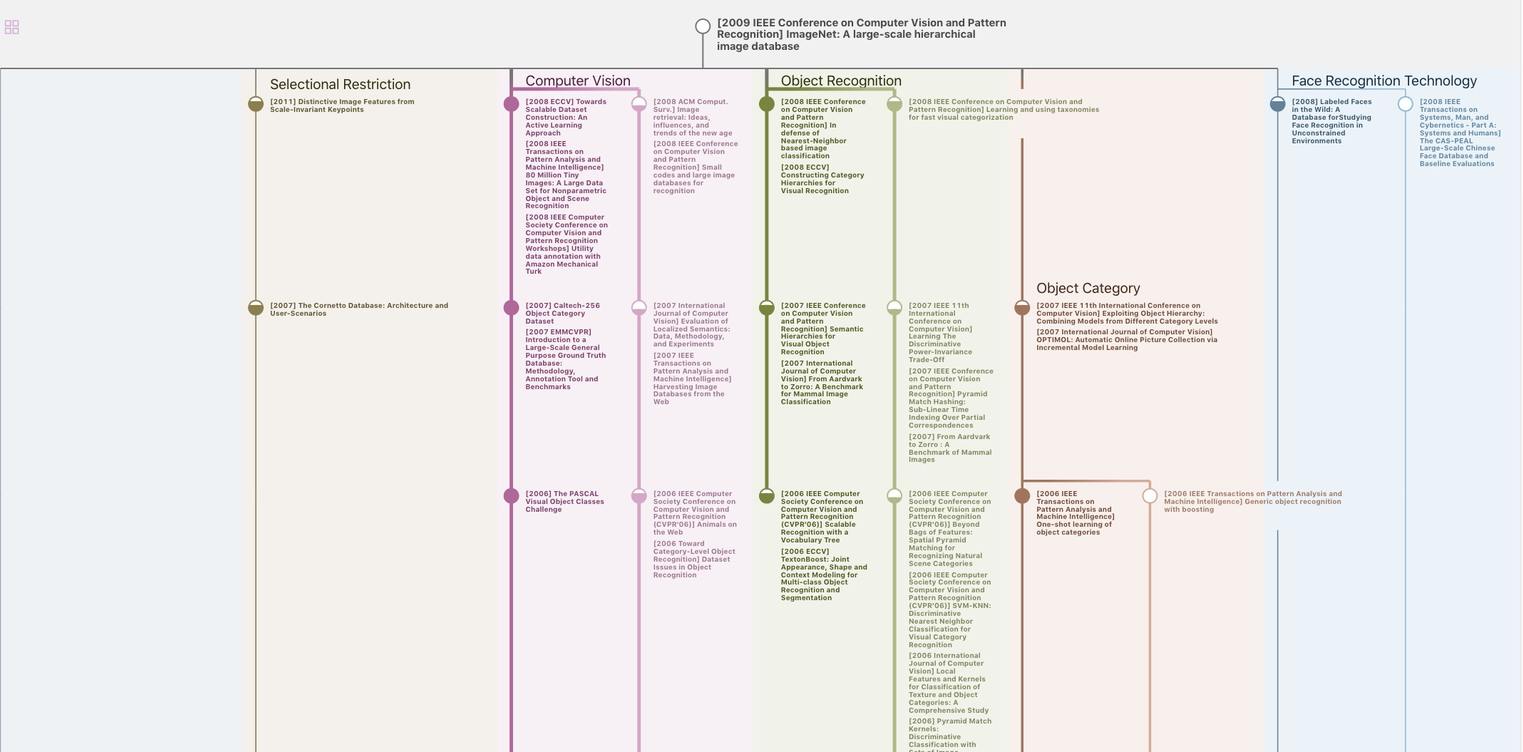
生成溯源树,研究论文发展脉络
Chat Paper
正在生成论文摘要