If your data distribution shifts, use self-learning
arxiv(2022)
摘要
We demonstrate that self-learning techniques like entropy minimization and pseudo-labeling are simple and effective at improving performance of a deployed computer vision model under systematic domain shifts. We conduct a wide range of large-scale experiments and show consistent improvements irrespective of the model architecture, the pre-training technique or the type of distribution shift. At the same time, self-learning is simple to use in practice because it does not require knowledge or access to the original training data or scheme, is robust to hyperparameter choices, is straight-forward to implement and requires only a few adaptation epochs. This makes self-learning techniques highly attractive for any practitioner who applies machine learning algorithms in the real world. We present state-of-the-art adaptation results on CIFAR10-C (8.5% error), ImageNet-C (22.0% mCE), ImageNet-R (17.4% error) and ImageNet-A (14.8% error), theoretically study the dynamics of self-supervised adaptation methods and propose a new classification dataset (ImageNet-D) which is challenging even with adaptation.
更多查看译文
关键词
data distribution shifts,self-learning
AI 理解论文
溯源树
样例
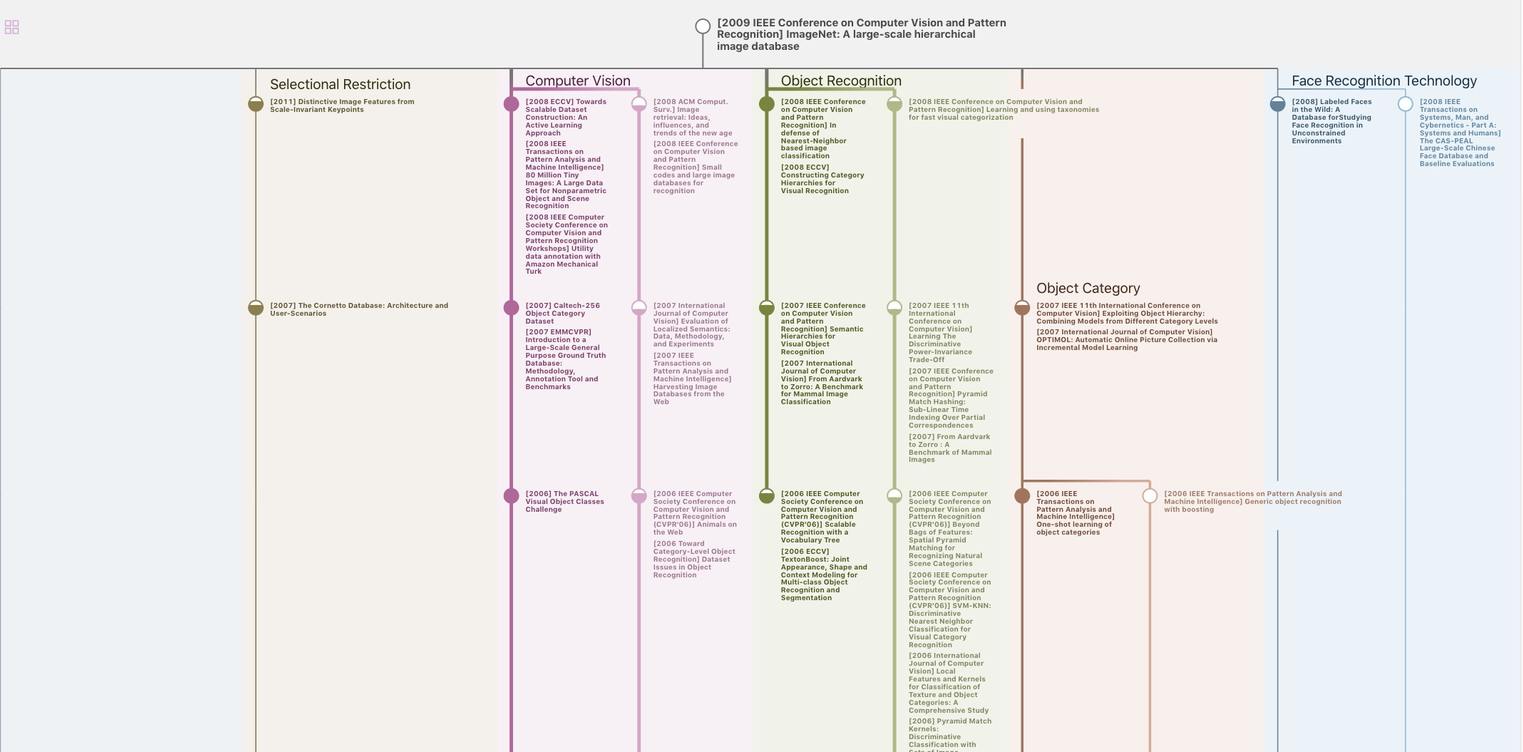
生成溯源树,研究论文发展脉络
Chat Paper
正在生成论文摘要