Performance Testing Using a Smart Reinforcement Learning-Driven Test Agent
2021 IEEE CONGRESS ON EVOLUTIONARY COMPUTATION (CEC 2021)(2021)
摘要
Performance testing with the aim of generating an efficient and effective workload to identify performance issues is challenging. Many of the automated approaches mainly rely on analyzing system models, source code, or extracting the usage pattern of the system during the execution. However, such information and artifacts are not always available. Moreover, all the transactions within a generated workload do not impact the performance of the system the same way, a finely tuned workload could accomplish the test objective in an efficient way. Model-free reinforcement learning is widely used for finding the optimal behavior to accomplish an objective in many decision-making problems without relying on a model of the system. This paper proposes that if the optimal policy (way) for generating test workload to meet a test objective can be learned by a test agent, then efficient test automation would be possible without relying on system models or source code. We present a self-adaptive reinforcement learning-driven load testing agent, RELOAD, that learns the optimal policy for test workload generation and generates an effective workload efficiently to meet the test objective. Once the agent learns the optimal policy, it can reuse the learned policy in subsequent testing activities. Our experiments show that the proposed intelligent load test agent can accomplish the test objective with lower test cost compared to common load testing procedures, and results in higher test efficiency.
更多查看译文
关键词
performance testing, load testing, workload generation, reinforcement learning, autonomous testing
AI 理解论文
溯源树
样例
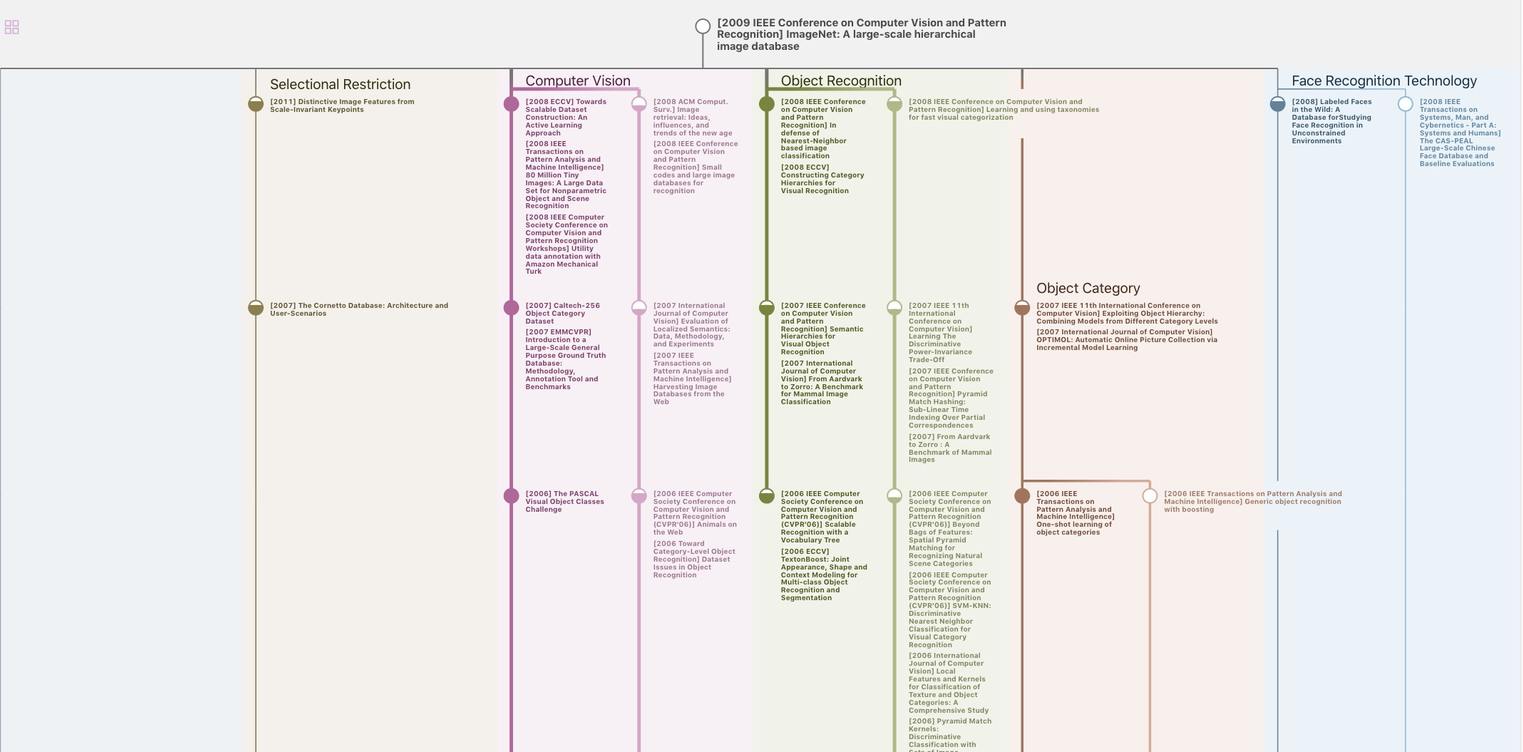
生成溯源树,研究论文发展脉络
Chat Paper
正在生成论文摘要