Learning Passage Impacts for Inverted Indexes
SIGIR '21 - PROCEEDINGS OF THE 44TH INTERNATIONAL ACM SIGIR CONFERENCE ON RESEARCH AND DEVELOPMENT IN INFORMATION RETRIEVAL(2021)
摘要
Neural information retrieval systems typically use a cascading pipeline, in which a first-stage model retrieves a candidate set of documents and one or more subsequent stages re-rank this set using contextualized language models such as BERT. In this paper, we propose DeepImpact, a new document term-weighting scheme suitable for efficient retrieval using a standard inverted index. Compared to existing methods, DeepImpact improves impact-score modeling and tackles the vocabulary-mismatch problem. In particular, DeepImpact leverages DocT5Query to enrich the document collection and, using a contextualized language model, directly estimates the semantic importance of tokens in a document, producing a single-value representation for each token in each document. Our experiments show that DeepImpact significantly outperforms prior first-stage retrieval approaches by up to 17% on effectiveness metrics w.r.t. DocT5Query, and, when deployed in a re-ranking scenario, can reach the same effectiveness of state-of-the-art approaches with up to 5.1x speedup in efficiency.
更多查看译文
关键词
Query processing,Inverted index,Neural IR,Term Weighting
AI 理解论文
溯源树
样例
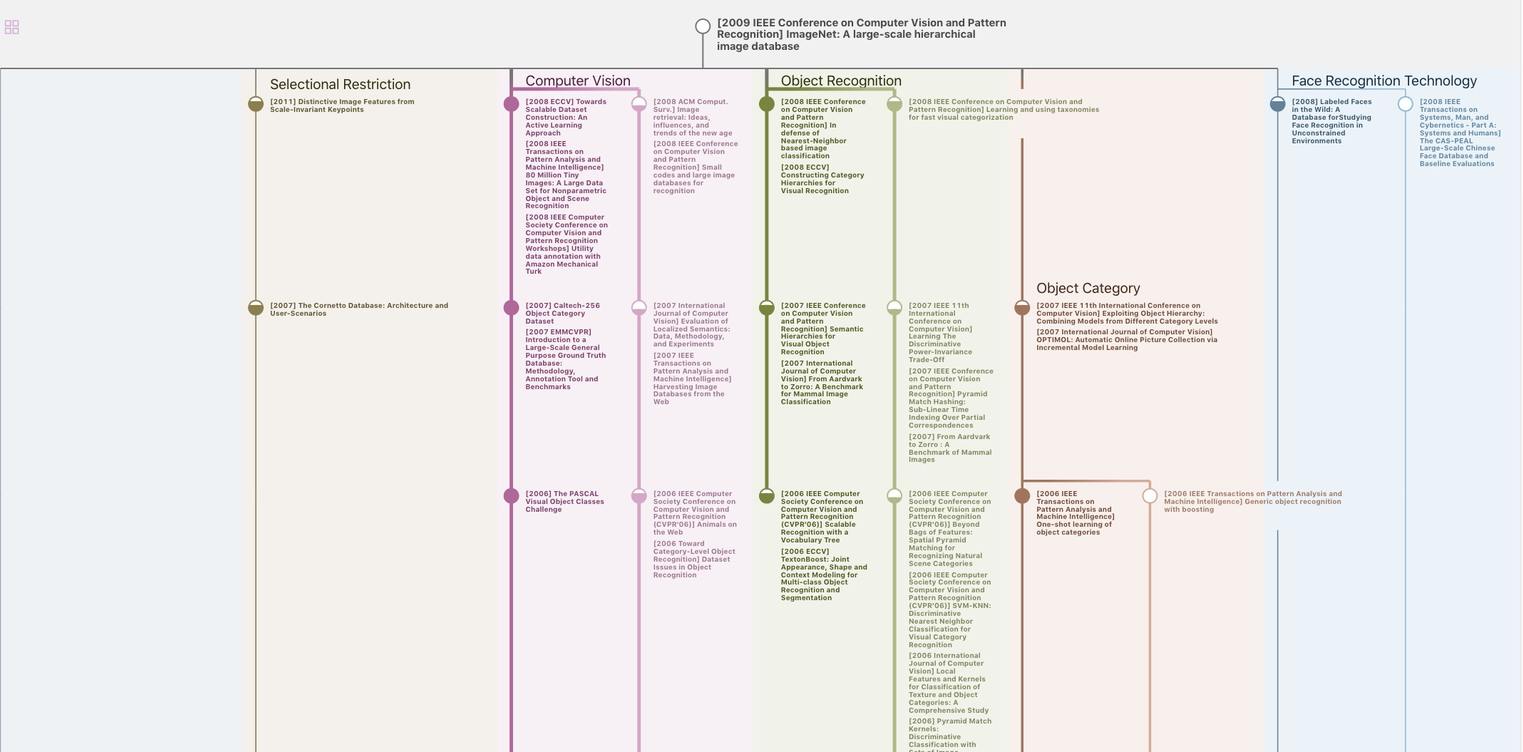
生成溯源树,研究论文发展脉络
Chat Paper
正在生成论文摘要