Achieving Small Test Error in Mildly Overparameterized Neural Networks
arxiv(2021)
摘要
Recent theoretical works on over-parameterized neural nets have focused on two aspects: optimization and generalization. Many existing works that study optimization and generalization together are based on neural tangent kernel and require a very large width. In this work, we are interested in the following question: for a binary classification problem with two-layer mildly over-parameterized ReLU network, can we find a point with small test error in polynomial time? We first show that the landscape of loss functions with explicit regularization has the following property: all local minima and certain other points which are only stationary in certain directions achieve small test error. We then prove that for convolutional neural nets, there is an algorithm which finds one of these points in polynomial time (in the input dimension and the number of data points). In addition, we prove that for a fully connected neural net, with an additional assumption on the data distribution, there is a polynomial time algorithm.
更多查看译文
关键词
neural networks,small test error,mildly
AI 理解论文
溯源树
样例
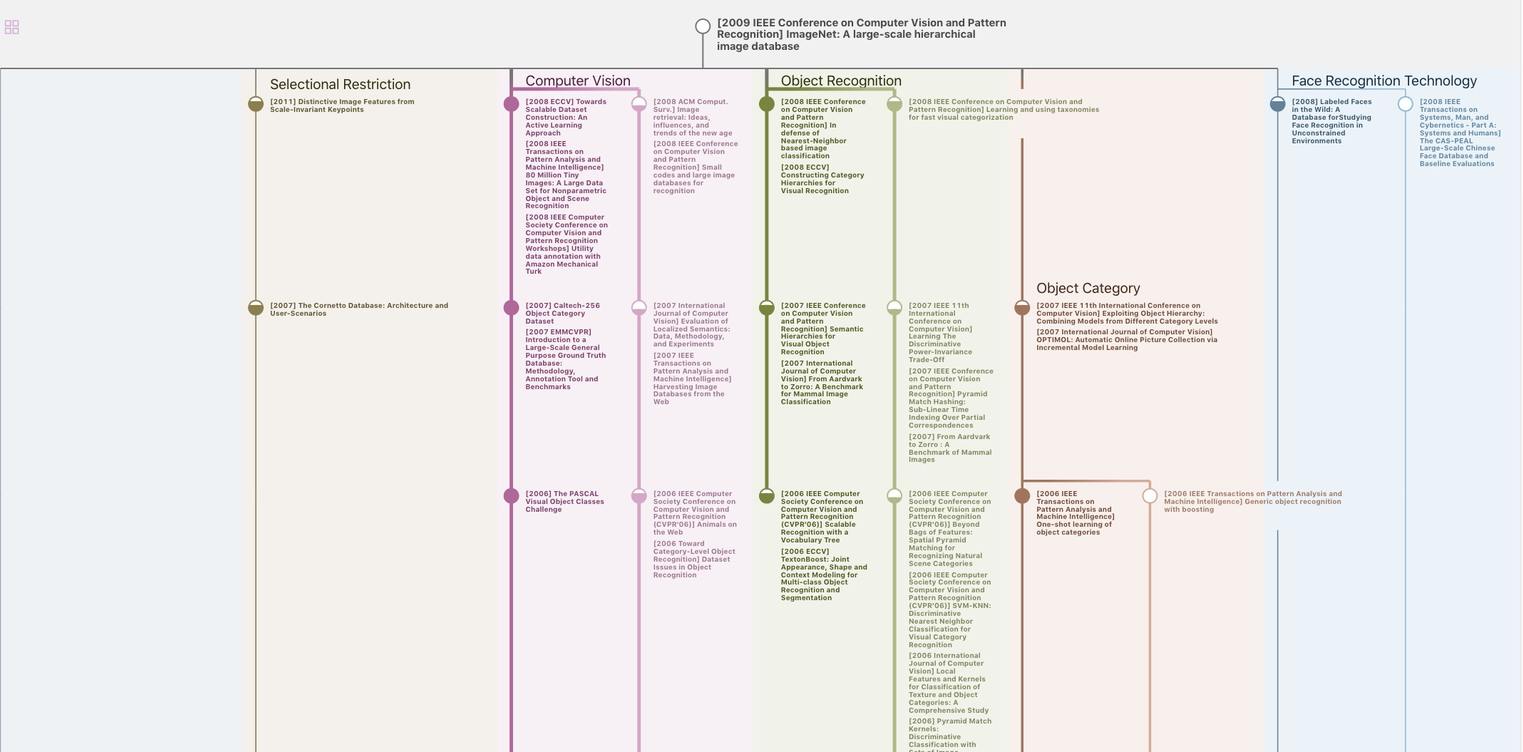
生成溯源树,研究论文发展脉络
Chat Paper
正在生成论文摘要