Multilingual Neural Machine Translation with Deep Encoder and Multiple Shallow Decoders.
EACL(2021)
摘要
Recent work in multilingual translation advances translation quality surpassing bilingual baselines using deep transformer models with increased capacity. However, the extra latency and memory costs introduced by this approach may make it unacceptable for efficiency-constrained applications. It has recently been shown for bilingual translation that using a deep encoder and shallow decoder (DESD) can reduce inference latency while maintaining translation quality, so we study similar speed-accuracy trade-offs for multilingual translation. We find that for many-to-one translation we can indeed increase decoder speed without sacrificing quality using this approach, but for one-to-many translation, shallow decoders cause a clear quality drop. To ameliorate this drop, we propose a deep encoder with multiple shallow decoders (DEMSD) where each shallow decoder is responsible for a disjoint subset of target languages. Specifically, the DEMSD model with 2-layer decoders is able to obtain a 1.8x speedup on average compared to a standard transformer model with no drop in translation quality.
更多查看译文
关键词
deep encoder,shallow decoders,translation
AI 理解论文
溯源树
样例
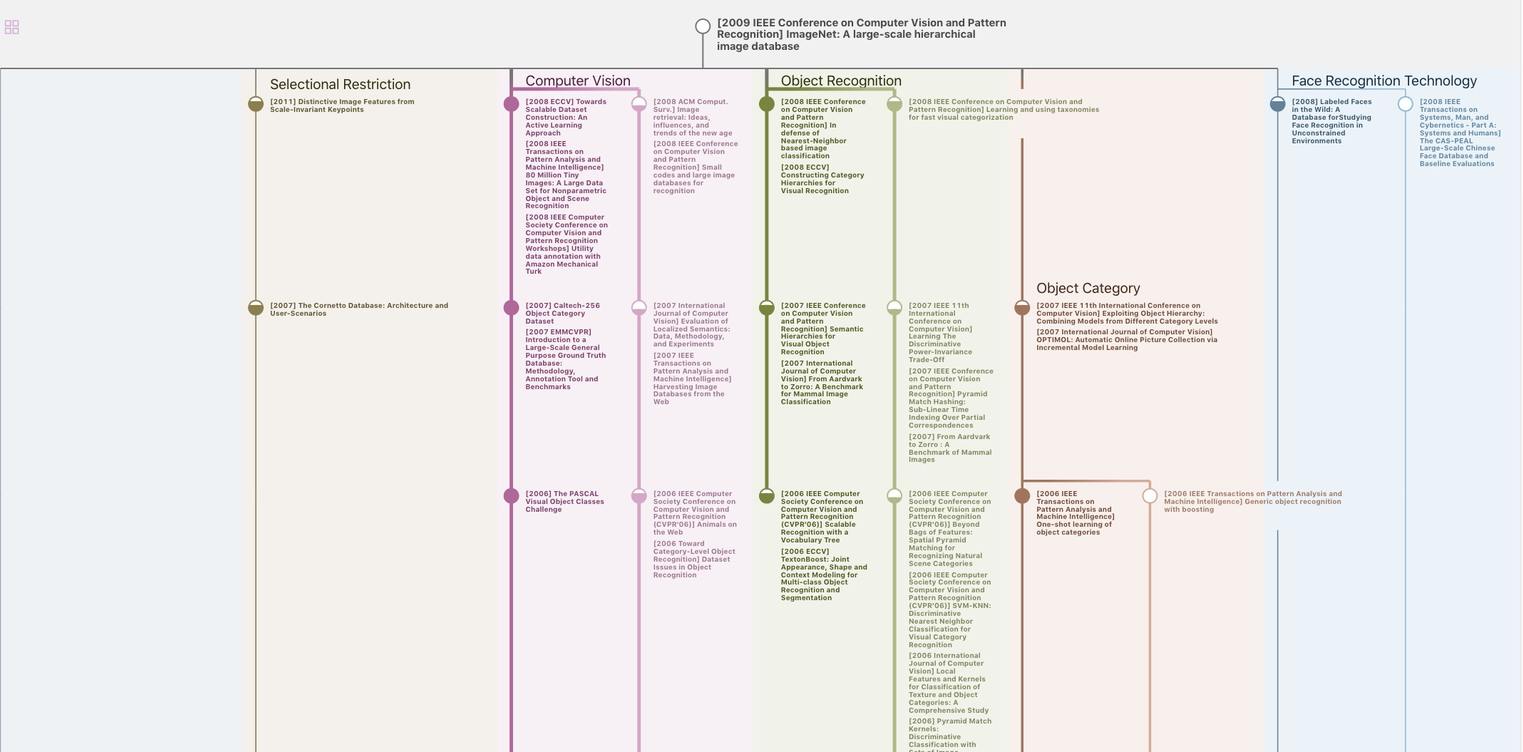
生成溯源树,研究论文发展脉络
Chat Paper
正在生成论文摘要