Comparison of RDSA and SPSA algorithms with different perturbations
2021 55TH ANNUAL CONFERENCE ON INFORMATION SCIENCES AND SYSTEMS (CISS)(2021)
摘要
Stochastic approximation (SA) algorithms can be used in system optimization problems when only noisy measurements of the system are available. This paper studies two types of SA algorithms in a multivariate Kiefer-Wolfowitz setting: random-direction SA (RDSA) and simultaneous-perturbation SA (SPSA), and then presents a complete proof for the convergence and asymptotic normality of the RDSA algorithm. Finally, this paper looks at several valid distributed perturbations in RDSA and SPSA and compares the two algorithms using mean-square errors in both theoretical and numerical ways.
更多查看译文
关键词
mean-square errors,simultaneous-perturbation SA,distributed perturbations,RDSA algorithm,asymptotic normality,convergence,complete proof,random-direction SA,multivariate Kiefer-Wolfowitz setting,SA algorithms,noisy measurements,system optimization problems,stochastic approximation algorithms,SPSA
AI 理解论文
溯源树
样例
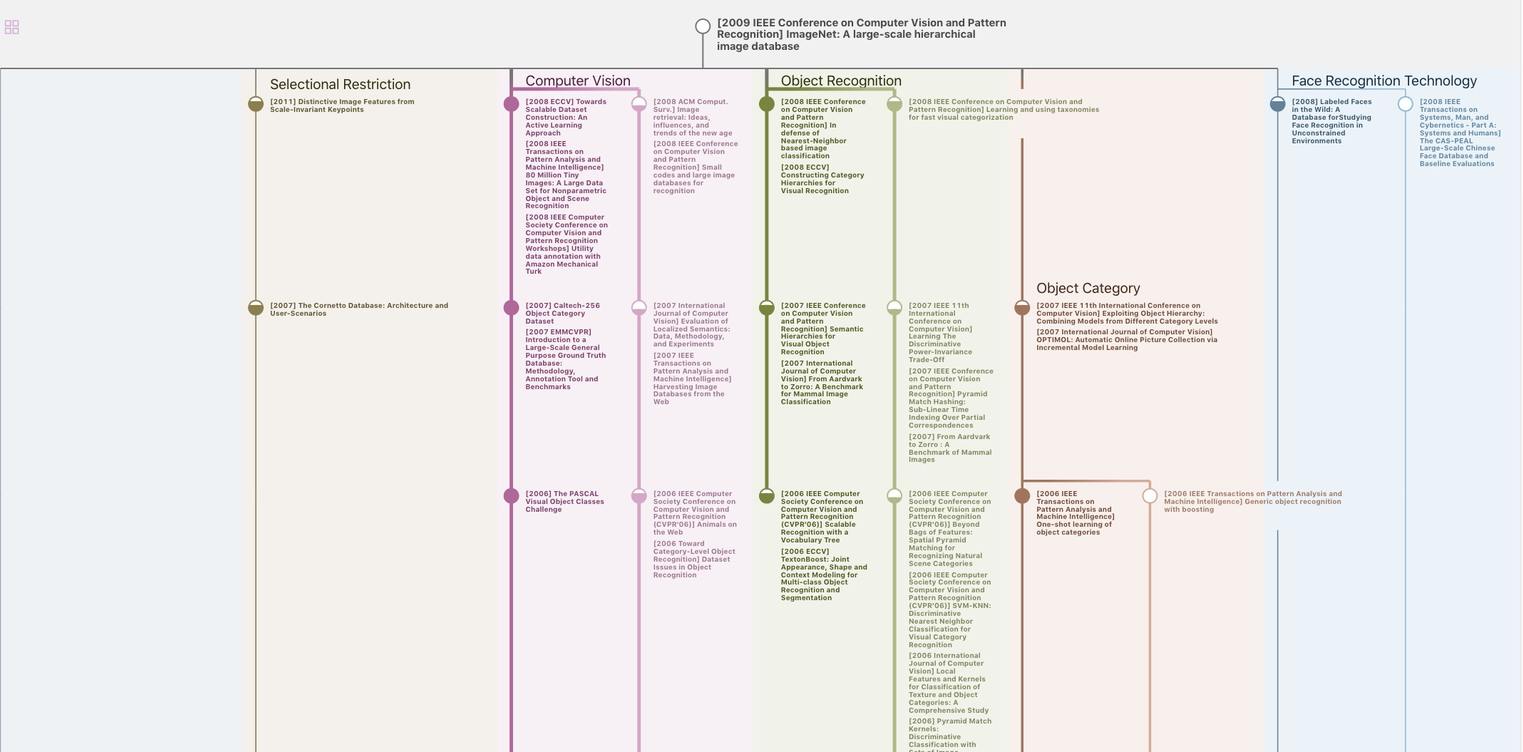
生成溯源树,研究论文发展脉络
Chat Paper
正在生成论文摘要