Multimodal Joint Head Orientation Estimation in Interacting Groups via Proxemics and Interaction Dynamics.
Proc. ACM Interact. Mob. Wearable Ubiquitous Technol.(2021)
摘要
Human head orientation estimation has been of interest because head orientation serves as a cue to directed social attention. Most existing approaches rely on visual and high-fidelity sensor inputs and deep learning strategies that do not consider the social context of unstructured and crowded mingling scenarios. We show that alternative inputs, like speaking status, body location, orientation, and acceleration contribute towards head orientation estimation. These are especially useful in crowded and in-the-wild settings where visual features are either uninformative due to occlusions or prohibitive to acquire due to physical space limitations and concerns of ecological validity. We argue that head orientation estimation in such social settings needs to account for the physically evolving interaction space formed by all the individuals in the group. To this end, we propose an LSTM-based head orientation estimation method that combines the hidden representations of the group members. Our framework jointly predicts head orientations of all group members and is applicable to groups of different sizes. We explain the contribution of different modalities to model performance in head orientation estimation. The proposed model outperforms baseline methods that do not explicitly consider the group context, and generalizes to an unseen dataset from a different social event.
更多查看译文
关键词
head orientation estimation,interaction dynamics,scene understanding
AI 理解论文
溯源树
样例
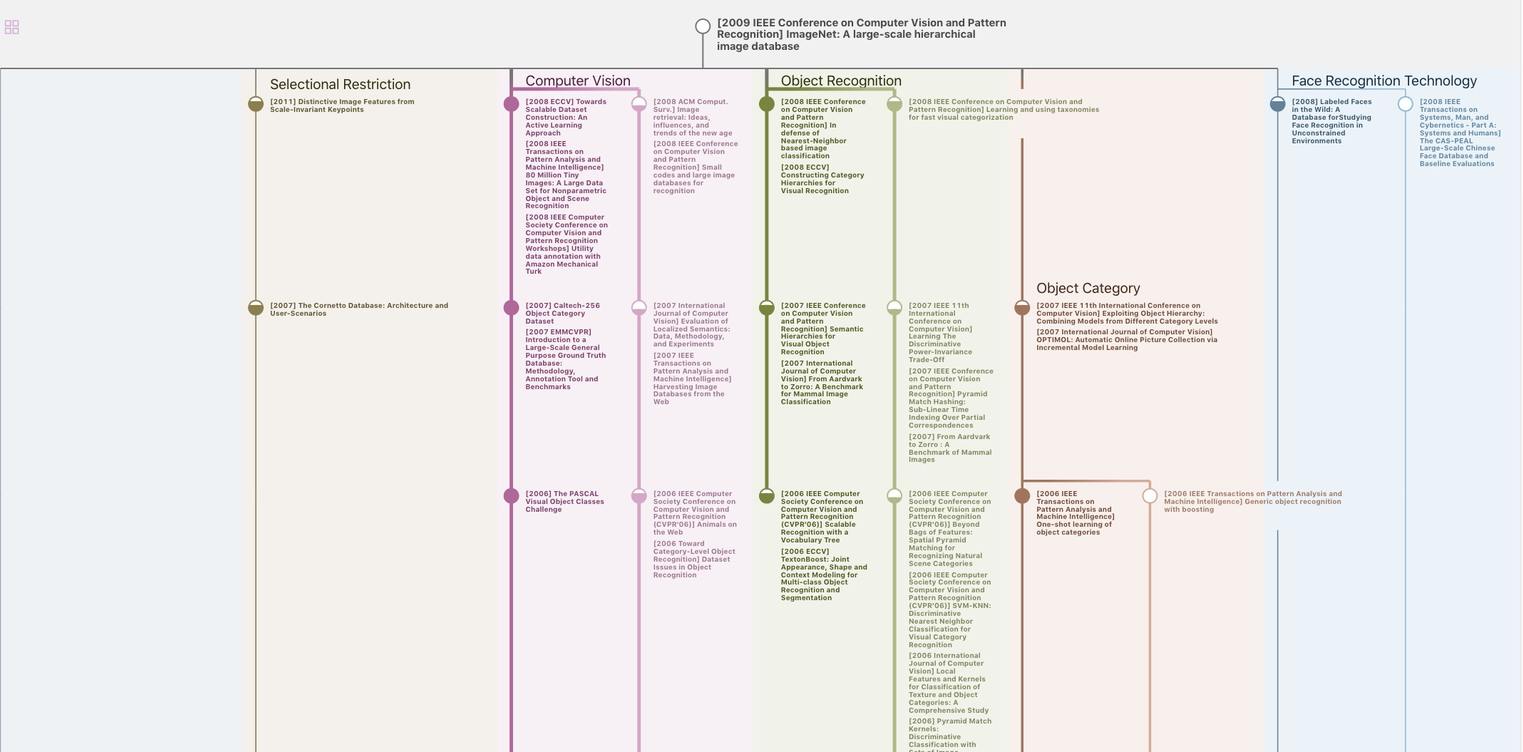
生成溯源树,研究论文发展脉络
Chat Paper
正在生成论文摘要