Weakly-Supervised Video Object Localization With Attentive Spatio-Temporal Correlation
PATTERN RECOGNITION LETTERS(2021)
摘要
Weakly-supervised video object localization is a challenging yet important task. The system should spatially localize the object of interest in videos, where only the descriptive sentences and their corresponding video segments are given in the training stage. Recent efforts propose to apply image-based Multiple Instance Learning (MIL) theory in this video task, and propagate the supervision from the video into frames by applying different frame-weighting strategies. Despite their promising progress, the spatiotemporal correlation between different object regions in videos has been largely ignored. To fill the research gap, in this work we introduce a simple but effective feature expression and aggregation framework, which utilizes the self-attention mechanism to capture the latent spatio-temporal correlation between multimodal object features and design a multimodal interaction module to model the similarity between the semantic query in sentences and the object regions in videos. We conduct extensive experimental evaluation on the YouCookII and ActivityNet-Entities datasets, which demonstrates significant improvements over multiple competitive baselines. (C) 2021 Published by Elsevier B.V.
更多查看译文
关键词
Video object localization, Spatio-temporal correlation, Weakly-supervised
AI 理解论文
溯源树
样例
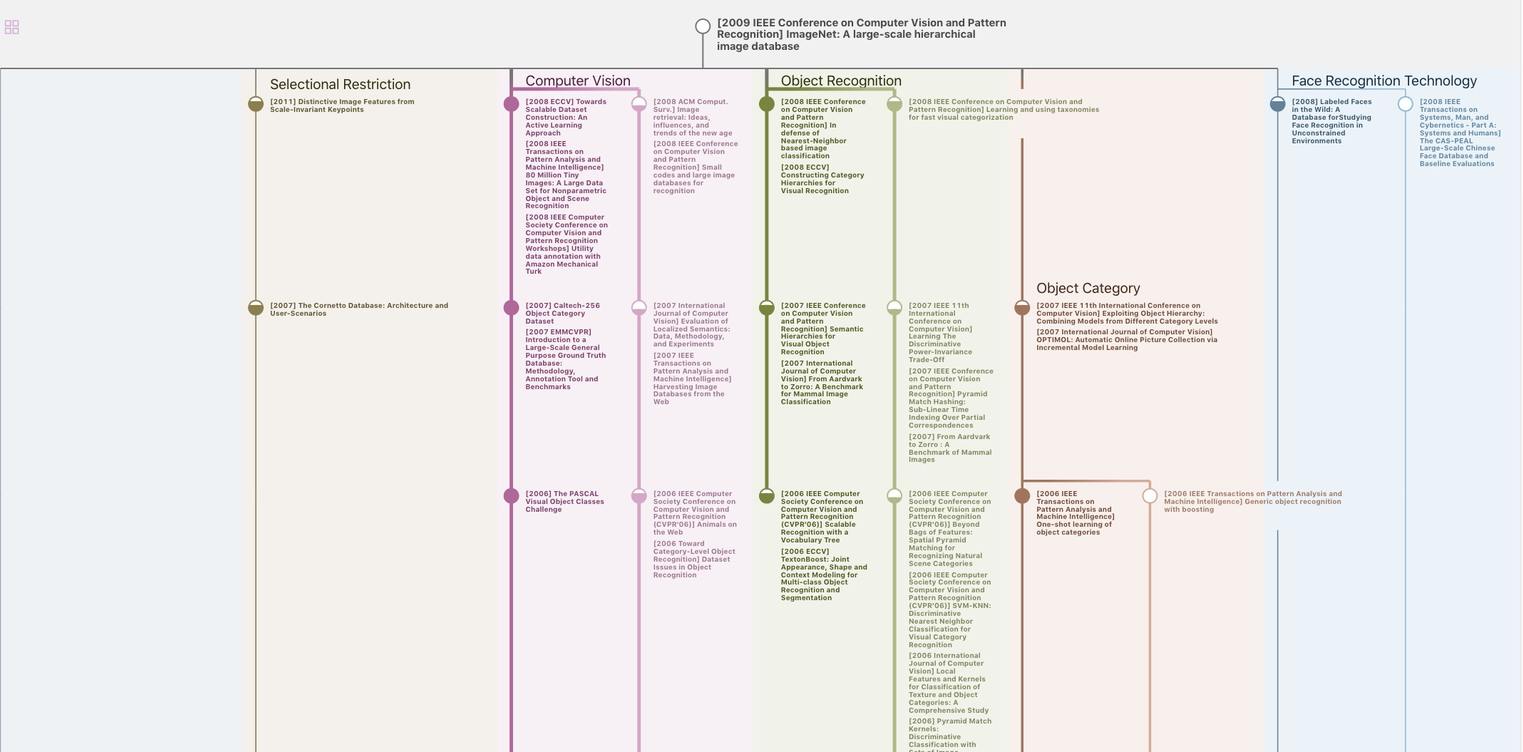
生成溯源树,研究论文发展脉络
Chat Paper
正在生成论文摘要