Explicit Facial Expression Transfer Via Fine-Grained Representations
IEEE TRANSACTIONS ON IMAGE PROCESSING(2021)
摘要
Facial expression transfer between two unpaired images is a challenging problem, as fine-grained expression is typically tangled with other facial attributes. Most existing methods treat expression transfer as an application of expression manipulation, and use predicted global expression, landmarks or action units (AUs) as a guidance. However, the prediction may be inaccurate, which limits the performance of transferring fine-grained expression. Instead of using an intermediate estimated guidance, we propose to explicitly transfer facial expression by directly mapping two unpaired input images to two synthesized images with swapped expressions. Specifically, considering AUs semantically describe fine-grained expression details, we propose a novel multi-class adversarial training method to disentangle input images into two types of fine-grained representations: AU-related feature and AU-free feature. Then, we can synthesize new images with preserved identities and swapped expressions by combining AU-free features with swapped AU-related features. Moreover, to obtain reliable expression transfer results of the unpaired input, we introduce a swap consistency loss to make the synthesized images and self-reconstructed images indistinguishable. Extensive experiments show that our approach outperforms the state-of-the-art expression manipulation methods for transferring fine-grained expressions while preserving other attributes including identity and pose.
更多查看译文
关键词
Training, Gold, Feature extraction, Facial features, Gallium nitride, Shape, Generative adversarial networks, Explicit facial expression transfer, fine-grained representation, multi-class adversarial training, swap consistency loss
AI 理解论文
溯源树
样例
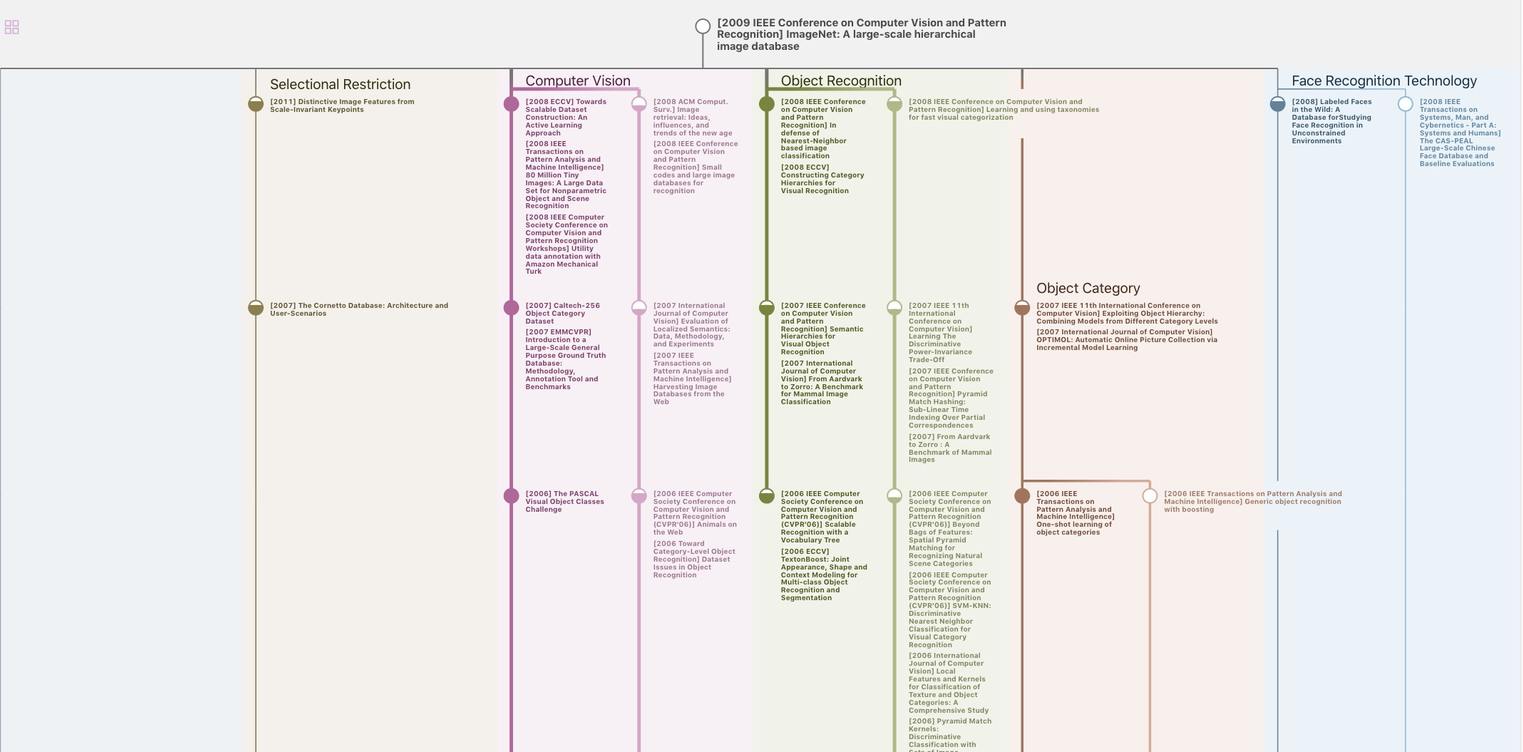
生成溯源树,研究论文发展脉络
Chat Paper
正在生成论文摘要