Deep Video Matting via Spatio-Temporal Alignment and Aggregation
2021 IEEE/CVF CONFERENCE ON COMPUTER VISION AND PATTERN RECOGNITION, CVPR 2021(2021)
摘要
Despite the significant progress made by deep learning in natural image matting, there has been so far no representative work on deep learning for video matting due to the inherent technical challenges in reasoning temporal domain and lack of large-scale video matting datasets. In this paper, we propose a deep learning-based video matting framework which employs a novel and effective spatio-temporal feature aggregation module (ST-FAM). As optical flow estimation can be very unreliable within matting regions, ST-FAM is designed to effectively align and aggregate information across different spatial scales and temporal frames within the network decoder. To eliminate frame-by-frame trimap annotations, a lightweight interactive trimap propagation network is also introduced. The other contribution consists of a large-scale video matting dataset with groundtruth alpha mattes for quantitative evaluation and real-world high-resolution videos with trimaps for qualitative evaluation. Quantitative and qualitative experimental results show that our framework significantly outperforms conventional video matting and deep image matting methods applied to video in presence of multi-frame temporal information. Our dataset is available at https://github.com/nowsyn/DVM.
更多查看译文
关键词
real-world high-resolution videos,conventional video matting,deep image matting methods,multiframe temporal information,deep video matting,spatio-temporal alignment,natural image matting,inherent technical challenges,reasoning temporal domain,large-scale video matting dataset,deep learning-based video matting framework,effective spatio-temporal feature aggregation module,matting regions,effectively align information,aggregate information,temporal frames,frame-by-frame trimap annotations,lightweight interactive trimap propagation network,quantitative evaluation
AI 理解论文
溯源树
样例
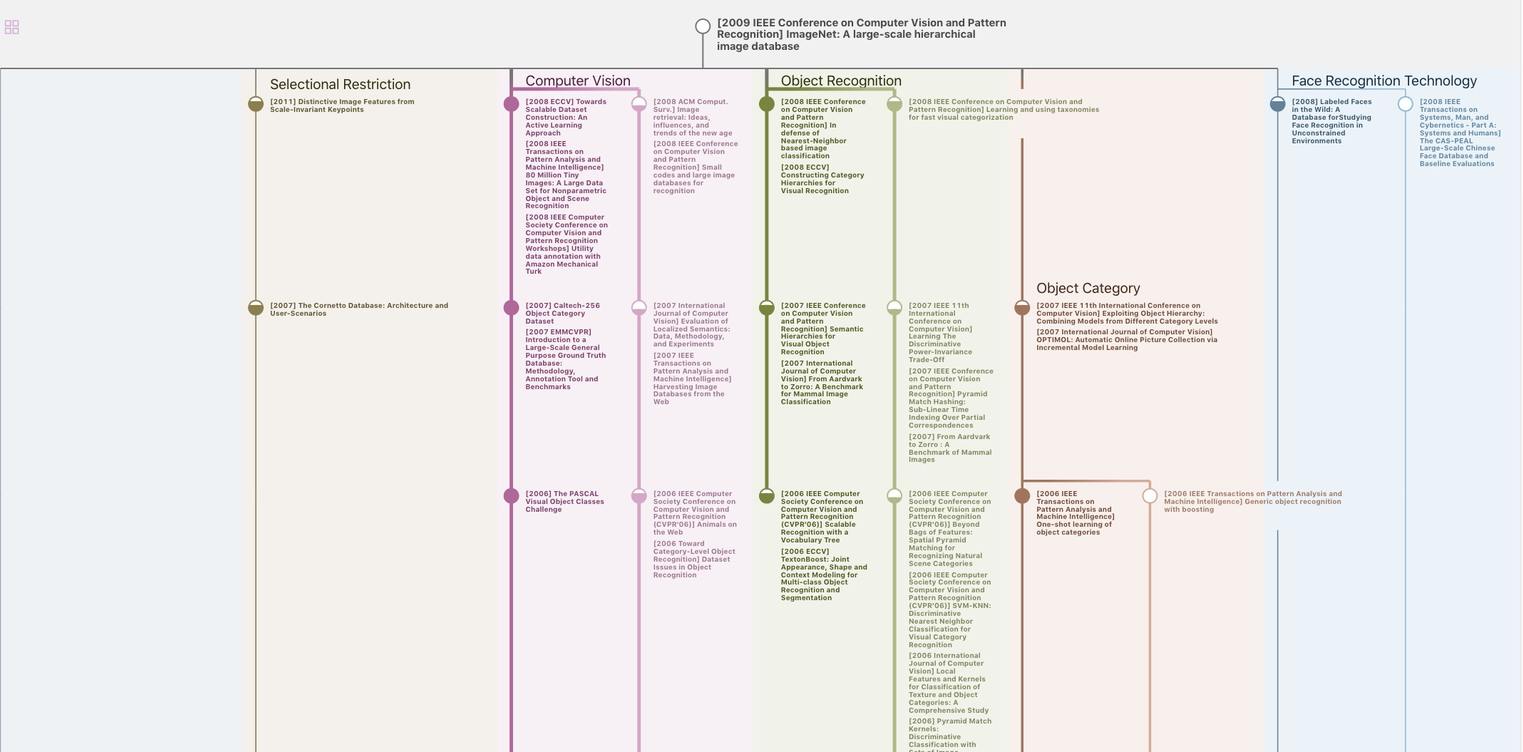
生成溯源树,研究论文发展脉络
Chat Paper
正在生成论文摘要