Human-in-the-loop Pose Estimation via Shared Autonomy
IUI(2021)
摘要
ABSTRACT Reliable, efficient shared autonomy requires balancing human operation and robot automation on complex tasks, such as dexterous manipulation. Adding to the difficulty of shared autonomy is a robot’s limited ability to perceive the 6 degree-of-freedom pose of objects, which is essential to perform manipulations those objects afforded. Inspired by Monte Carlo Localization, we propose a generative human-in-the-loop approach to estimating object pose. We characterize the performance of our mixed-initiative 3D registration approach using 2D pointing devices via a user study. Seeking an analog for Fitts’s Law for 3D registration, we introduce a new evaluation framework that takes the entire registration process into account instead of only the outcome. When combined with estimates of registration confidence, we posit that mixed-initiative registration will reduce the human workload while maintaining or even improving final pose estimation accuracy.
更多查看译文
关键词
pose estimation, human-in-the-loop, shared autonomy, affordances, Monte Carlo localization
AI 理解论文
溯源树
样例
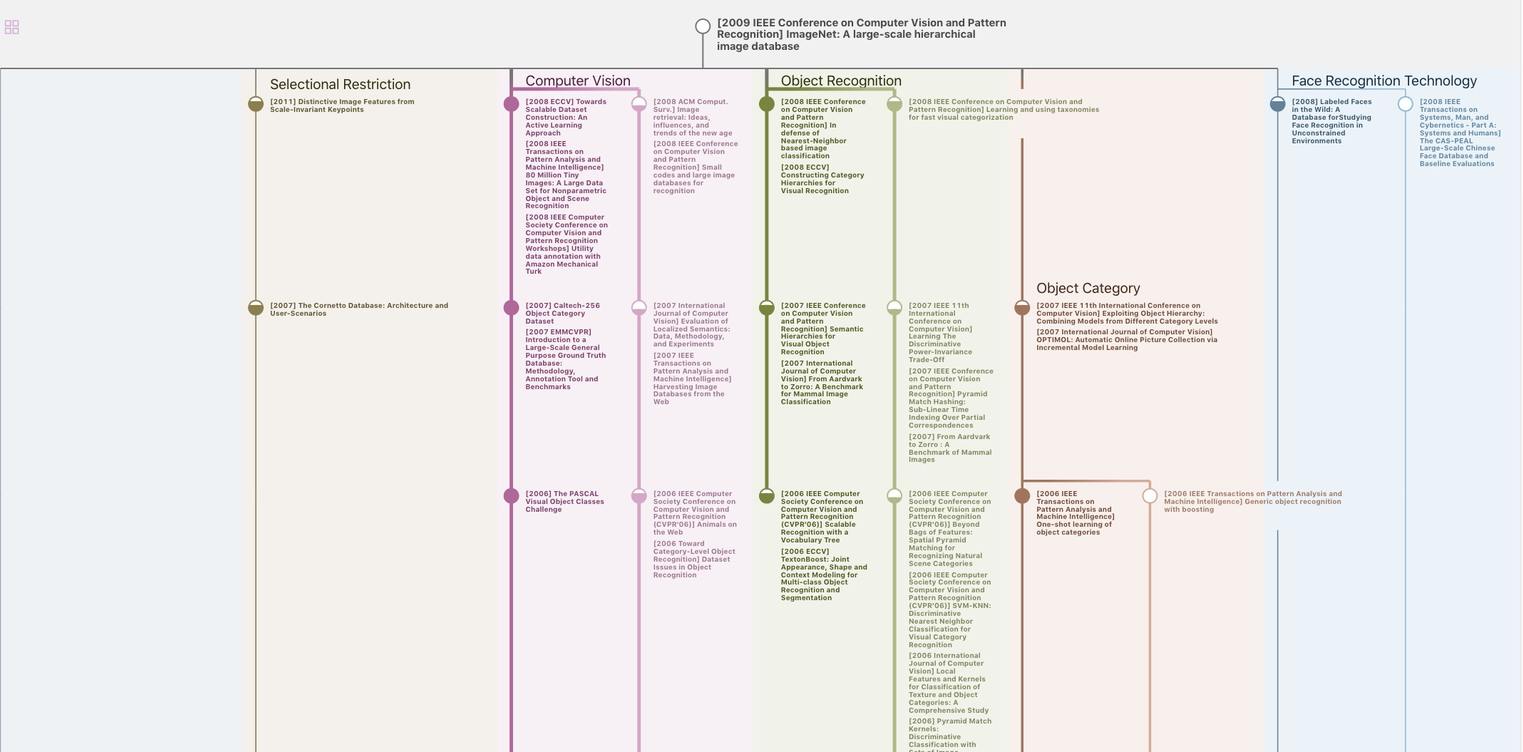
生成溯源树,研究论文发展脉络
Chat Paper
正在生成论文摘要