An Affordance Keypoint Detection Network for Robot Manipulation
IEEE ROBOTICS AND AUTOMATION LETTERS(2021)
摘要
This letter investigates the addition of keypoint detections to a deep network affordance segmentation pipeline. The intent is to better interpret the functionality of object parts from a manipulation perspective. While affordance segmentation does provide label information about the potential use of object parts, it lacks predictions on the physical geometry that would support such use. The keypoints remedy the situation by providing structured predictions regarding position, direction, and extent. To support joint training of affordances and keypoints, a new dataset is created based on the UMD dataset. Called the UMD+GT affordance dataset, it emphasizes household objects and affordances. The dataset has a uniform representation for five keypoints that encodes information about where and how to manipulate the associated affordance. Visual processing benchmarking shows that the trained network, called AffKp, achieves the state-of-the-art performance on affordance segmentation and satisfactory result on keypoint detection. Manipulation experiments show more stable detection of the operating position for AffKp versus segmentation-only methods and the ability to infer object part pose and operating direction for task execution.
更多查看译文
关键词
Deep learning in grasping and manipulation, perception for grasping and manipulation, RGB-D perception
AI 理解论文
溯源树
样例
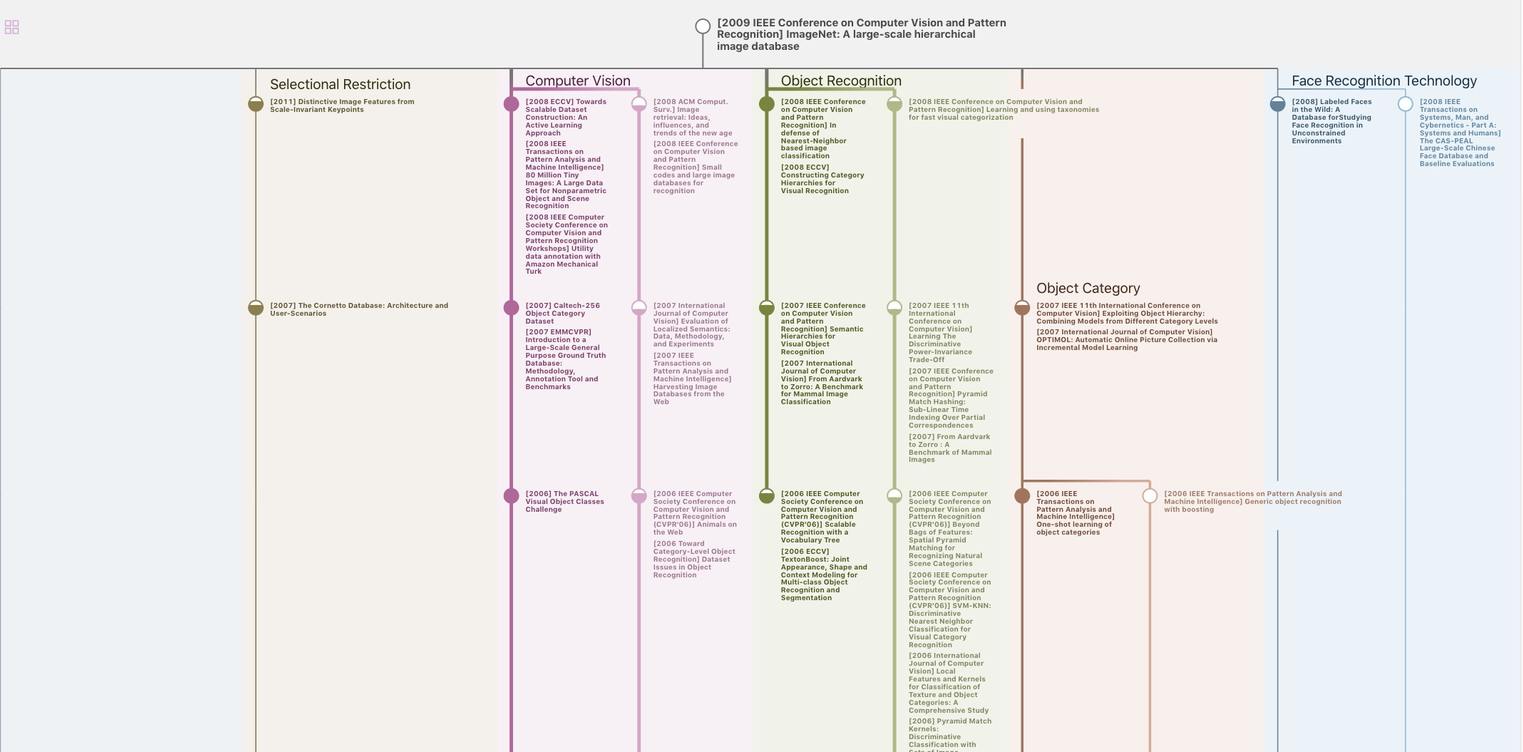
生成溯源树,研究论文发展脉络
Chat Paper
正在生成论文摘要