Double-Branch Network With Pyramidal Convolution And Iterative Attention For Hyperspectral Image Classification
REMOTE SENSING(2021)
摘要
Deep-learning methods, especially convolutional neural networks (CNN), have become the first choice for hyperspectral image (HSI) classification to date. It is a common procedure that small cubes are cropped from hyperspectral images and then fed into CNNs. However, standard CNNs find it difficult to extract discriminative spectral-spatial features. How to obtain finer spectral-spatial features to improve the classification performance is now a hot topic of research. In this regard, the attention mechanism, which has achieved excellent performance in other computer vision, holds the exciting prospect. In this paper, we propose a double-branch network consisting of a novel convolution named pyramidal convolution (PyConv) and an iterative attention mechanism. Each branch concentrates on exploiting spectral or spatial features with different PyConvs, supplemented by the attention module for refining the feature map. Experimental results demonstrate that our model can yield competitive performance compared to other state-of-the-art models.
更多查看译文
关键词
hyperspectral, deep learning, convolutional neural network, attention mechanism
AI 理解论文
溯源树
样例
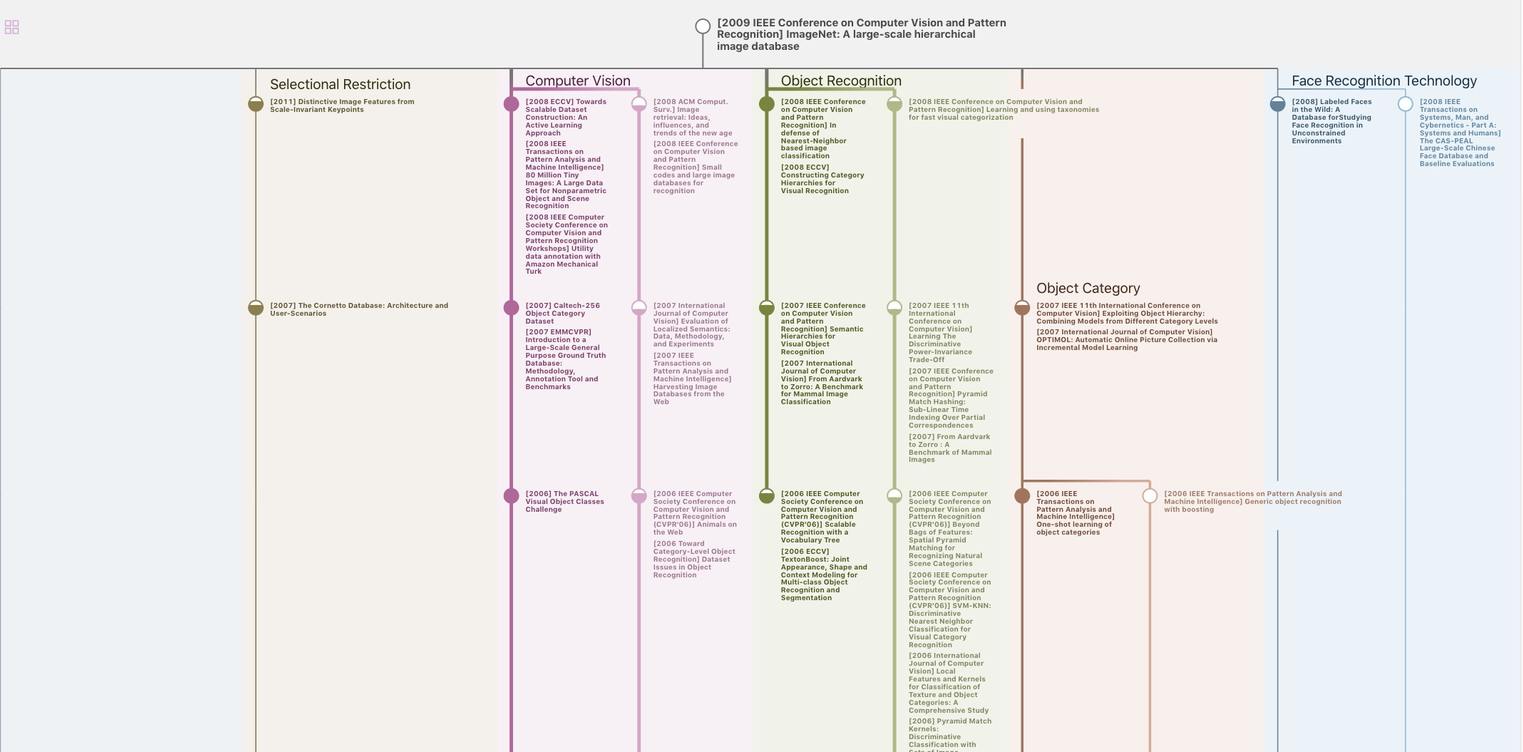
生成溯源树,研究论文发展脉络
Chat Paper
正在生成论文摘要