Ocean-Surface Heterogeneity Mapping (Ohma) To Identify Regions Of Change
REMOTE SENSING(2021)
摘要
Mapping heterogeneity of the ocean's surface waters is important for understanding biogeographical distributions, ocean surface habitat mapping, and ocean surface stability. This article describes the Ocean-surface Heterogeneity MApping (OHMA) algorithm-an objective, replicable approach that uses hypertemporal, satellite-derived datasets to map the spatio-temporal heterogeneity of ocean surface waters. The OHMA produces a suite of complementary datasets-a surface spatio-temporal heterogeneity dataset, and an optimised spatio-temporal classification of the ocean surface. It was demonstrated here using a hypertemporal Sea Surface Temperature image dataset of the North Atlantic. Validation with Underway-derived temperature data showed higher heterogeneity areas were associated with stronger surface temperature gradients, or an increased presence of locally extreme temperature values. Using four exploratory case studies, spatio-temporal heterogeneity values were related to a range of region-specific surface and sub-surface characteristics including fronts, currents and bathymetry. The values conveyed the interactions between these parameters as a single metric. Such over-arching heterogeneity information is virtually impossible to map from in-situ instruments, or less temporally dense satellite datasets. This study demonstrated the OHMA approach is a useful and robust tool to explore, examine, and describe the ocean's surface. It advances our capability to map biologically relevant measures of ocean surface heterogeneity. It can support ongoing efforts in Ocean Surface Partitioning, and attempts to understand marine species distributions. The study highlighted the need to establish dedicated spatio-temporal ocean validation sites, specifically measured using surface transits, to support advances in hypertemporal ocean data use, and exploitation. A number of future research avenues are also highlighted.
更多查看译文
关键词
hypertemporal satellite imagery, SST, North Atlantic, heterogeneity, surface waters, ocean surface partitioning, water masses, spatio-temporal, ISODATA, Generalised Linear Models
AI 理解论文
溯源树
样例
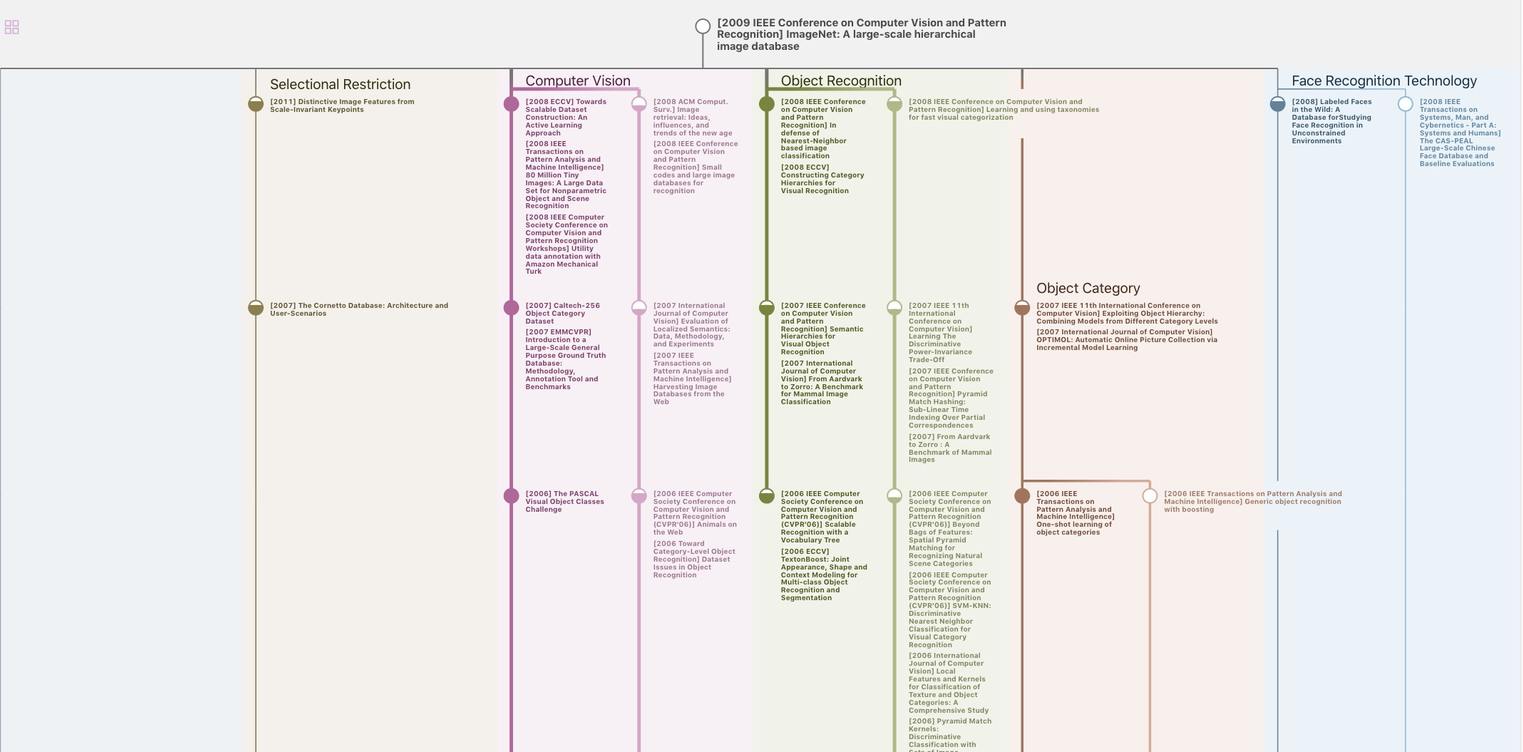
生成溯源树,研究论文发展脉络
Chat Paper
正在生成论文摘要