A Fully Spiking Hybrid Neural Network for Energy-Efficient Object Detection
IEEE TRANSACTIONS ON IMAGE PROCESSING(2021)
摘要
This paper proposes a Fully Spiking Hybrid Neural Network (FSHNN) for energy-efficient and robust object detection in resource-constrained platforms. The network architecture is based on a Spiking Convolutional Neural Network using leaky-integrate-fire neuron models. The model combines unsupervised Spike Time-Dependent Plasticity (STDP) learning with back-propagation (STBP) learning methods and also uses Monte Carlo Dropout to get an estimate of the uncertainty error. FSHNN provides better accuracy compared to DNN based object detectors while being more energy-efficient. It also outperforms these object detectors, when subjected to noisy input data and less labeled training data with a lower uncertainty error.
更多查看译文
关键词
Biological neural networks, Object detection, Training, Neurons, Detectors, Standards, Feature extraction, Spiking neural networks, leaky integrate and fire, uncertainty estimation, generalization, object detection
AI 理解论文
溯源树
样例
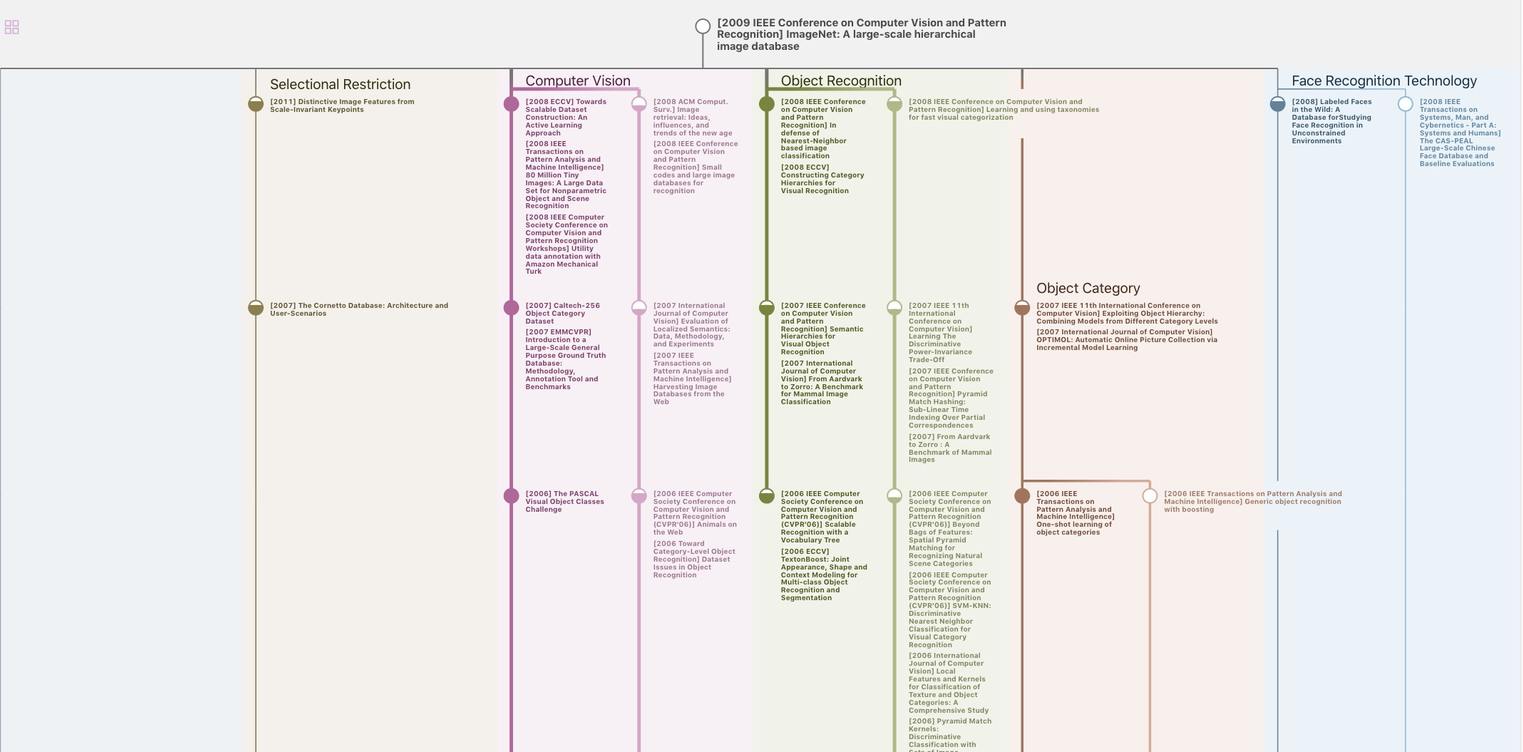
生成溯源树,研究论文发展脉络
Chat Paper
正在生成论文摘要