Robust Kernel-Based Distribution Regression
INVERSE PROBLEMS(2021)
摘要
Regularization schemes for regression have been widely studied in learning theory and inverse problems. In this paper, we study regularized distribution regression (DR) which involves two stages of sampling, and aims at regressing from probability measures to real-valued responses by regularization over a reproducing kernel Hilbert space. Many important tasks in statistical learning and inverse problems can be treated in this framework. Examples include multi-instance learning and point estimation for problems without analytical solutions. Recently, theoretical analysis on DR has been carried out via kernel ridge regression and several interesting learning behaviors have been observed. However, the topic has not been explored and understood beyond the least squares based DR. By introducing a robust loss function l ( sigma ) for two-stage sampling problems, we present a novel robust distribution regression (RDR) scheme. With a windowing function V and a scaling parameter sigma which can be appropriately chosen, l ( sigma ) can include a wide range of commonly used loss functions that enrich the theme of DR. Moreover, the loss l ( sigma ) is not necessarily convex, which enlarges the regression class (least squares) in the literature of DR. Learning rates in different regularity ranges of the regression function are comprehensively studied and derived via integral operator techniques. The scaling parameter sigma is shown to be crucial in providing robustness and satisfactory learning rates of RDR.
更多查看译文
关键词
learning theory, distribution regression, robust regression, integral operator, learning rate
AI 理解论文
溯源树
样例
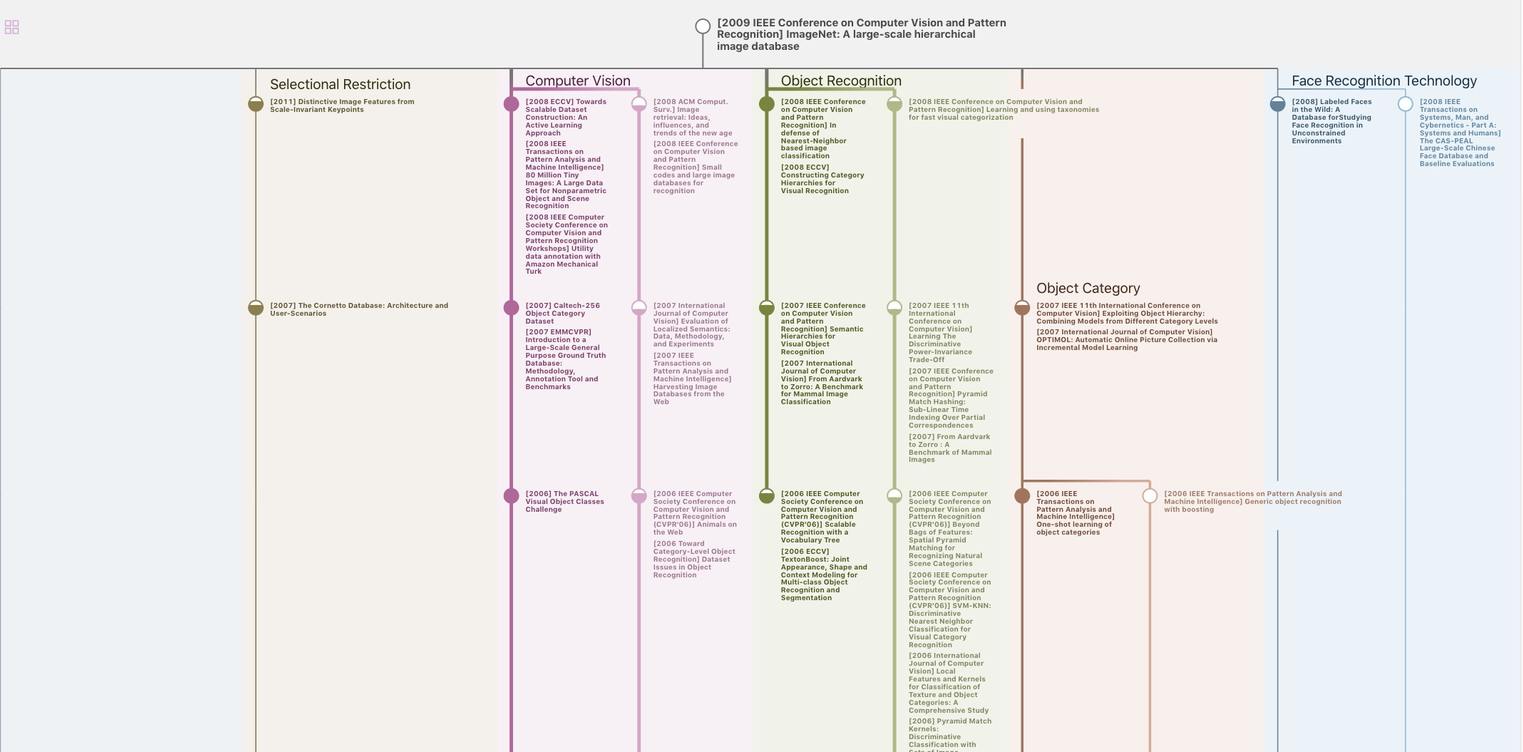
生成溯源树,研究论文发展脉络
Chat Paper
正在生成论文摘要