Low-Rank Riemannian Optimization for Graph-Based Clustering Applications
IEEE Transactions on Pattern Analysis and Machine Intelligence(2022)
摘要
With the abundance of data, machine learning applications engaged increased attention in the last decade. An attractive approach to robustify the statistical analysis is to preprocess the data through clustering. This paper develops a low-complexity Riemannian optimization framework for solving optimization problems on the set of positive semidefinite stochastic matrices. The low-complexity feature of the proposed algorithms stems from the factorization of the optimization variable
$\mathbf {X}=\mathbf {Y}\mathbf {Y}^{\mathrm{T}}$
and deriving conditions on the number of columns of
$\mathbf {Y}$
under which the factorization yields a satisfactory solution. The paper further investigates the embedded and quotient geometries of the resulting Riemannian manifolds. In particular, the paper explicitly derives the tangent space, Riemannian gradients and Hessians, and a retraction operator allowing the design of efficient first and second-order optimization methods for the graph-based clustering applications of interest. The numerical results reveal that the resulting algorithms present a clear complexity advantage as compared with state-of-the-art euclidean and Riemannian approaches for graph clustering applications.
更多查看译文
关键词
Riemannian manifolds,low-rank factorization,graph-based clustering,convex and non-convex optimization
AI 理解论文
溯源树
样例
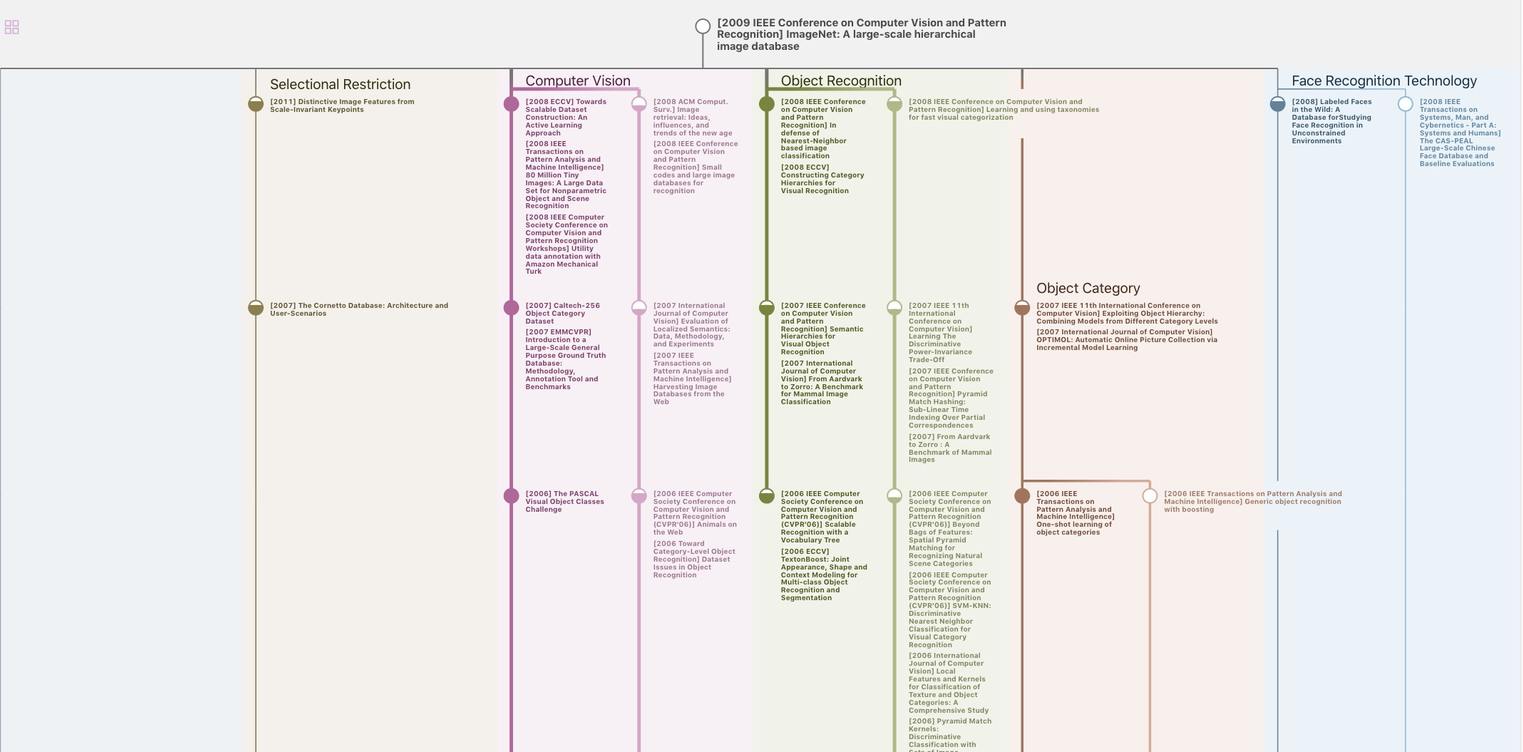
生成溯源树,研究论文发展脉络
Chat Paper
正在生成论文摘要