Asymmetric Compressive Learning Guarantees With Applications to Quantized Sketches
IEEE Transactions on Signal Processing(2022)
摘要
The compressive learning framework reduces the computational cost of training on large-scale datasets. In a sketching phase, the data is first compressed to a lightweight sketch vector, obtained by mapping the data samples through a well-chosen feature map, and averaging those contributions. In a learning phase, the desired model parameters are then extracted from this sketch by solving an optimization problem, which also involves a feature map. When the feature map is identical during the sketching and learning phases, formal statistical guarantees (excess risk bounds) have been proven. However, the desirable properties of the feature map are different during sketching and learning (
e.g.
, quantized outputs, and differentiability, respectively). We thus study the relaxation where this map is allowed to be different for each phase. First, we prove that the existing guarantees carry over to this asymmetric scheme, up to a controlled error term, provided some Limited Projected Distortion (LPD) property holds. We then instantiate this framework to the setting of quantized sketches, by proving that the LPD indeed holds for binary sketch contributions. Finally, we further validate the approach with numerical simulations, including a large-scale application in audio event classification.
更多查看译文
关键词
Asymmetric embeddings,compressive statistical learning,Gaussian mixture modeling,quantization
AI 理解论文
溯源树
样例
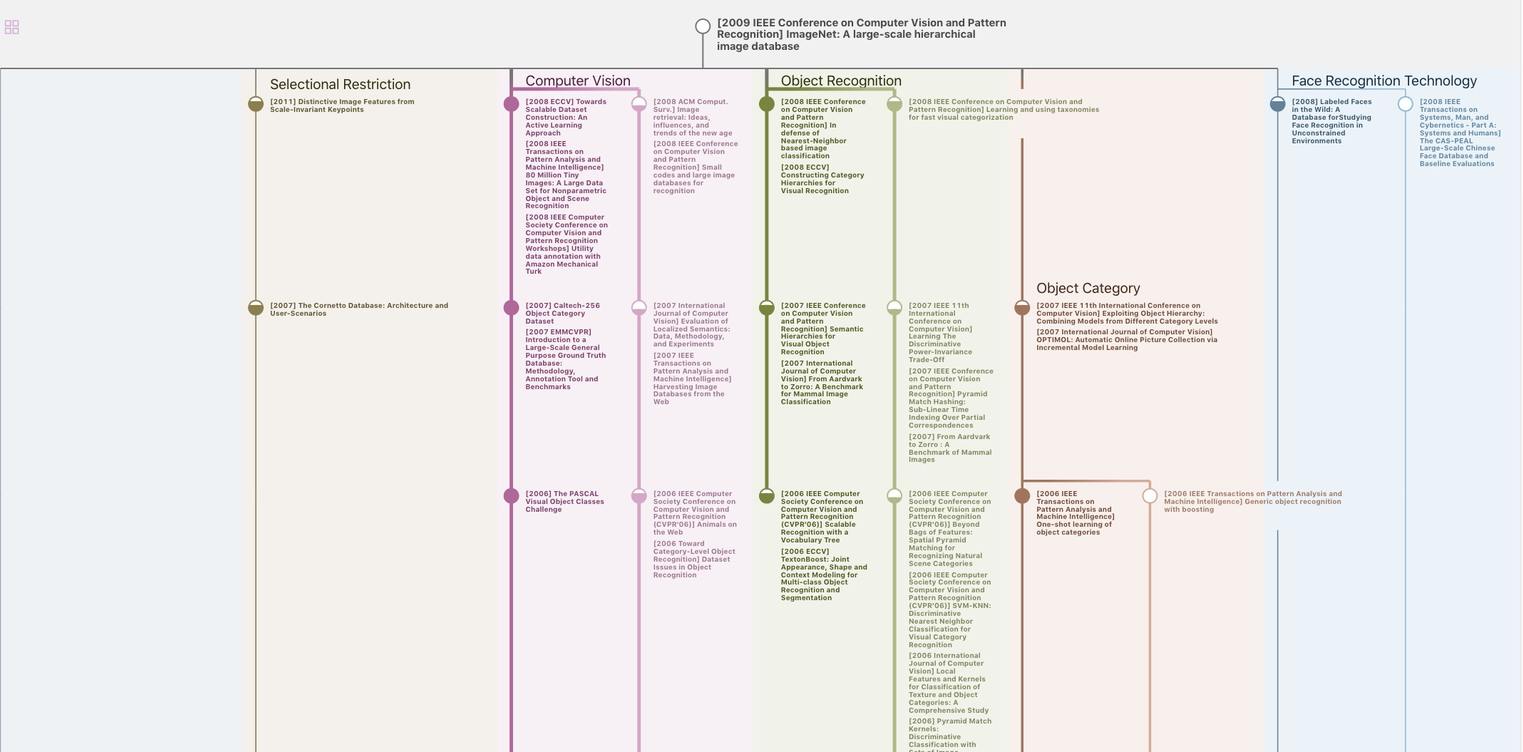
生成溯源树,研究论文发展脉络
Chat Paper
正在生成论文摘要