Cross-Domain Adaptive Clustering for Semi-Supervised Domain Adaptation
2021 IEEE/CVF CONFERENCE ON COMPUTER VISION AND PATTERN RECOGNITION, CVPR 2021(2021)
摘要
In semi-supervised domain adaptation, a few labeled samples per class in the target domain guide features of the remaining target samples to aggregate around them. However, the trained model cannot produce a highly discriminative feature representation for the target domain because the training data is dominated by labeled samples from the source domain. This could lead to disconnection between the labeled and unlabeled target samples as well as misalignment between unlabeled target samples and the source domain. In this paper, we propose a novel approach called Cross-domain Adaptive Clustering to address this problem. To achieve both inter-domain and intra-domain adaptation, we first introduce an adversarial adaptive clustering loss to group features of unlabeled target data into clusters and perform cluster-wise feature alignment across the source and target domains. We further apply pseudo labeling to unlabeled samples in the target domain and retain pseudo-labels with high confidence. Pseudo labeling expands the number of "labeled" samples in each class in the target domain, and thus produces a more robust and powerful cluster core for each class to facilitate adversarial learning. Extensive experiments on benchmark datasets, including DomainNet, Office-Home and Office, demonstrate that our proposed approach achieves the state-of-the-art performance in semi-supervised domain adaptation.
更多查看译文
关键词
semisupervised domain adaptation,highly discriminative feature representation,source domain,labeled target samples,unlabeled target samples,adversarial adaptive clustering loss,cluster-wise feature alignment,cross-domain adaptive clustering,training data,intradomain adaptation,interdomain adaptation,pseudolabeling,adversarial learning
AI 理解论文
溯源树
样例
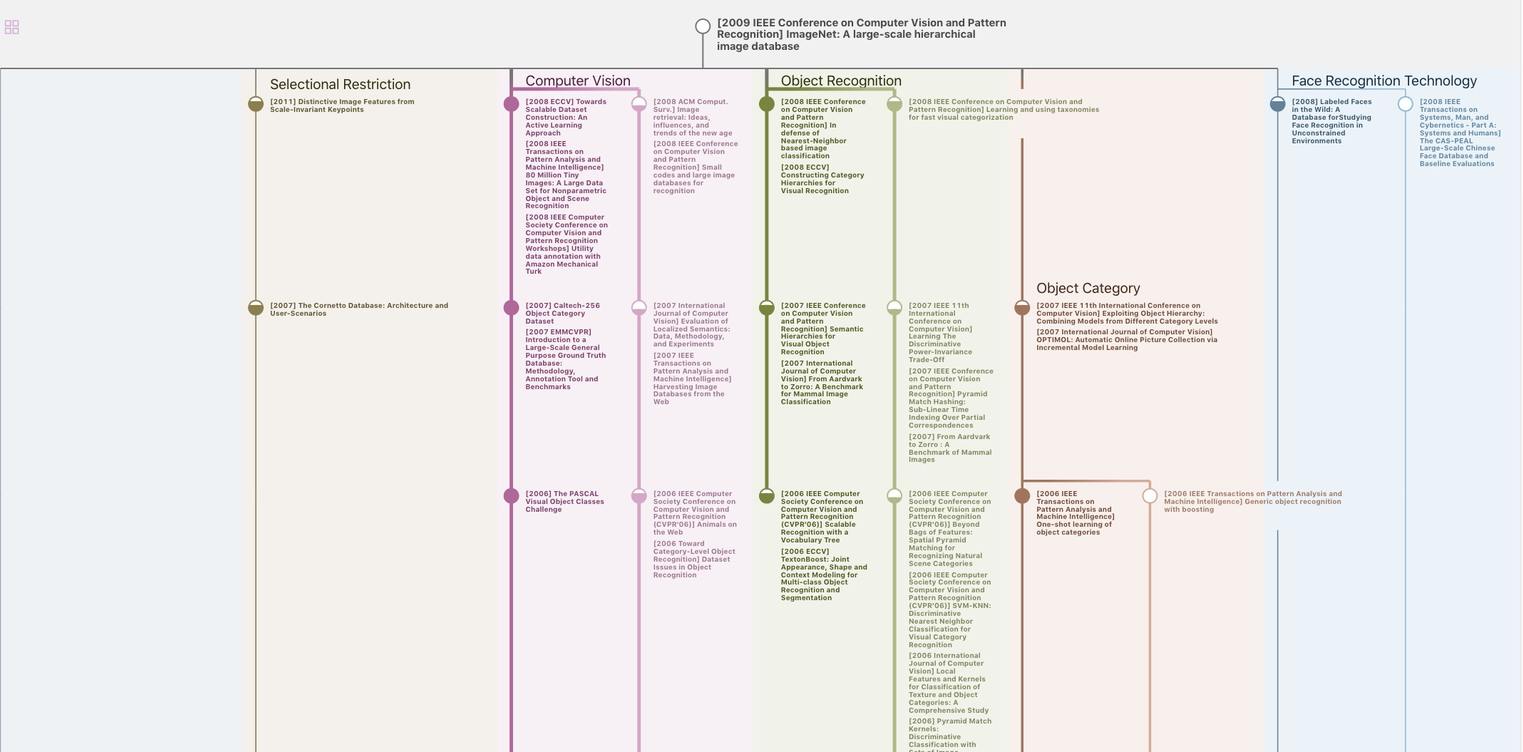
生成溯源树,研究论文发展脉络
Chat Paper
正在生成论文摘要