Labels, Information, and Computation: Efficient Learning Using Sufficient Labels
JOURNAL OF MACHINE LEARNING RESEARCH(2023)
摘要
In supervised learning, obtaining a large set of fully-lab eled training data is expensive. We show that we do not always need full label information on every single training example to train a competent classifier. Specifically, inspired by the principle of sufficiency in statistics, we present a statistic (a summary) of the fully-lab eled training set that captures almost all the relevant information for classification but at the same time is easier to obtain directly. We call this statistic "sufficiently-lab eled data" and prove its sufficiency and efficiency for finding the optimal hidden representations, on which competent classifier heads can be trained using as few as a single randomly-chosen fully-lab eled example per class. Sufficiently-lab eled data can be obtained from annotators directly without collecting the fully-lab eled data first. And we prove that it is easier to directly obtain sufficiently-lab eled data than obtaining fully-lab eled data. Furthermore, sufficiently-lab eled data is naturally more secure since it stores relative, instead of absolute, information. Extensive experimental results are provided to support our theory.
更多查看译文
关键词
Classification,Deep Learning,Data Efficiency,Data Security,Privacy-Preserving Learning
AI 理解论文
溯源树
样例
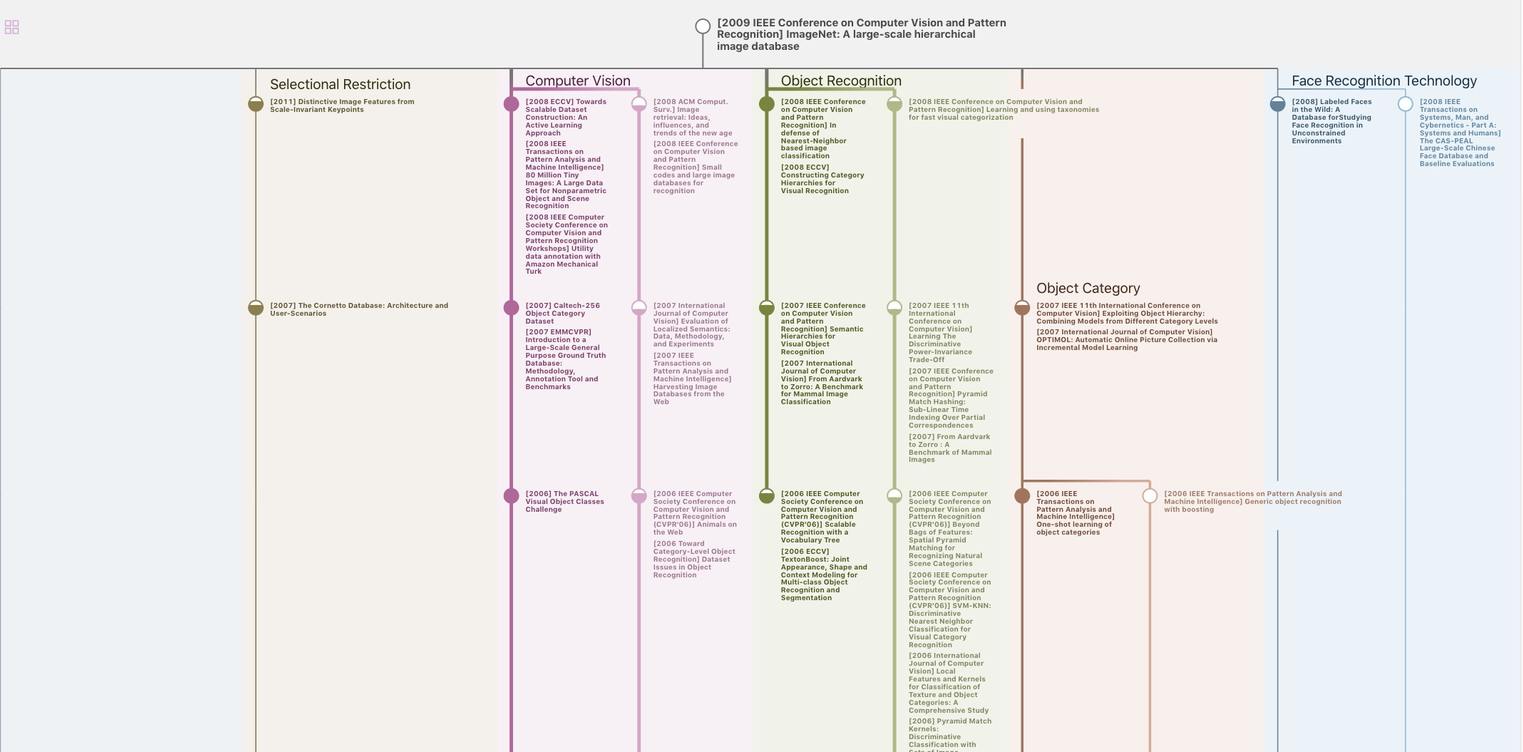
生成溯源树,研究论文发展脉络
Chat Paper
正在生成论文摘要