Generative Context Pair Selection for Multi-hop Question Answering.
EMNLP(2021)
摘要
Compositional reasoning tasks like multi-hop question answering, require making latent decisions to get the final answer, given a question. However, crowdsourced datasets often capture only a slice of the underlying task distribution, which can induce unanticipated biases in models performing compositional reasoning. Furthermore, discriminatively trained models exploit such biases to get a better held-out performance, without learning the right way to reason, as they do not necessitate paying attention to the question representation (conditioning variable) in its entirety, to estimate the answer likelihood. In this work, we propose a generative context selection model for multi-hop question answering that reasons about how the given question could have been generated given a context pair. While being comparable to the state-of-the-art answering performance, our proposed generative passage selection model has a better performance (4.9% higher than baseline) on adversarial held-out set which tests robustness of model's multi-hop reasoning capabilities.
更多查看译文
关键词
generative context pair selection,multi-hop
AI 理解论文
溯源树
样例
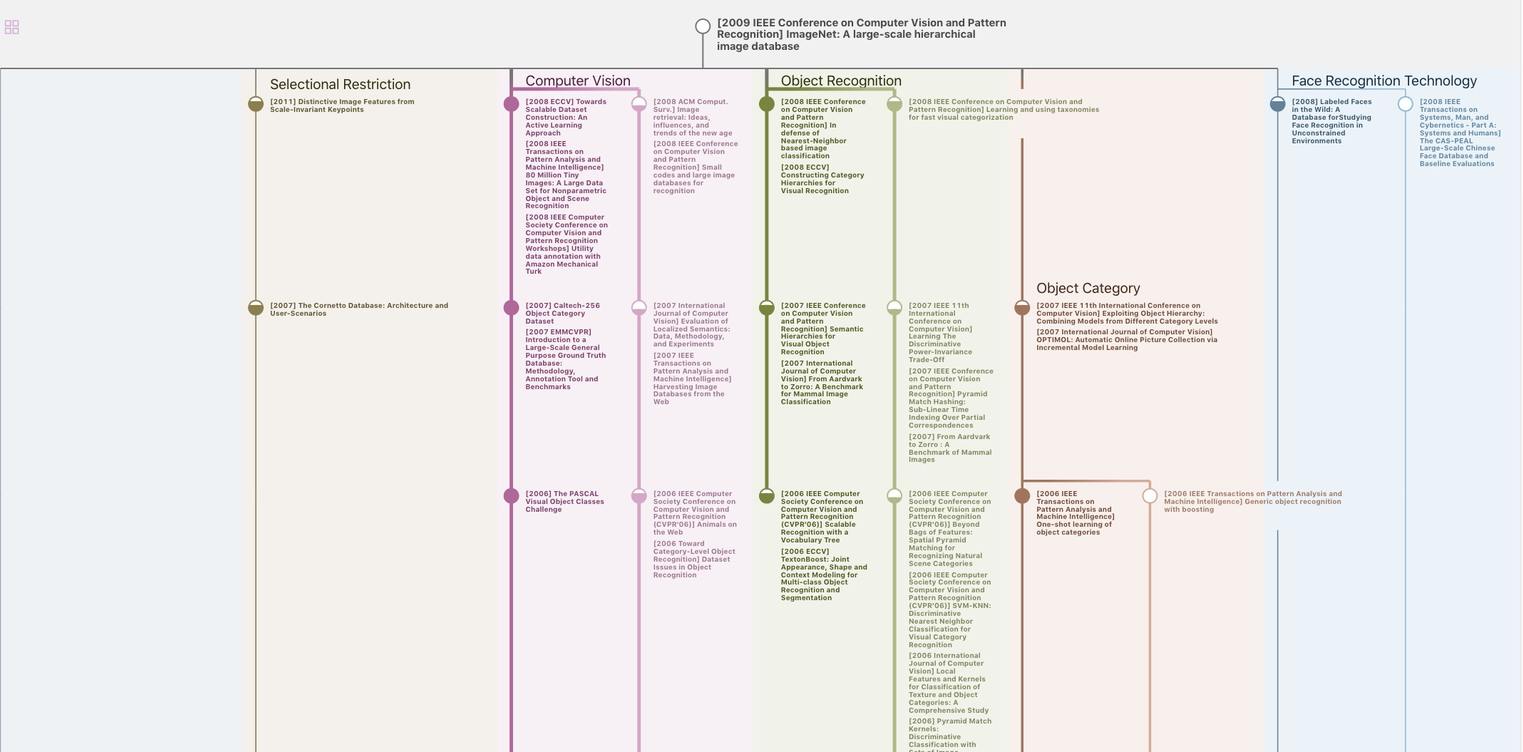
生成溯源树,研究论文发展脉络
Chat Paper
正在生成论文摘要