Enabling Probabilistic Differential Privacy Protection for Location Recommendations
IEEE Transactions on Services Computing(2021)
摘要
The sequential pattern in the human movement is one of the most important aspects for location recommendations in geosocial networks. Existing location recommenders have to access users' raw check-in data to mine their sequential patterns that raises serious location privacy breaches. In this paper, we propose a new Privacy-preserving LOcation REcommendation framework (PLORE) to address this privacy challenge. First, we employ the nnth-order additive Markov chain to exploit users' sequential patterns for location recommendations. Further, we contrive the probabilistic differential privacy mechanism to reach a good trade-off between high recommendation accuracy and strict location privacy protection. Finally, we conduct extensive experiments to evaluate the performance of PLORE using three large-scale real-world data sets. Extensive experimental results show that PLORE provides efficient and highly accurate location recommendations, and guarantees strict privacy protection for user check-in data in geosocial networks.
更多查看译文
关键词
Location recommendations,sequential patterns,additive Markov chain,probabilistic differential privacy,noise injection
AI 理解论文
溯源树
样例
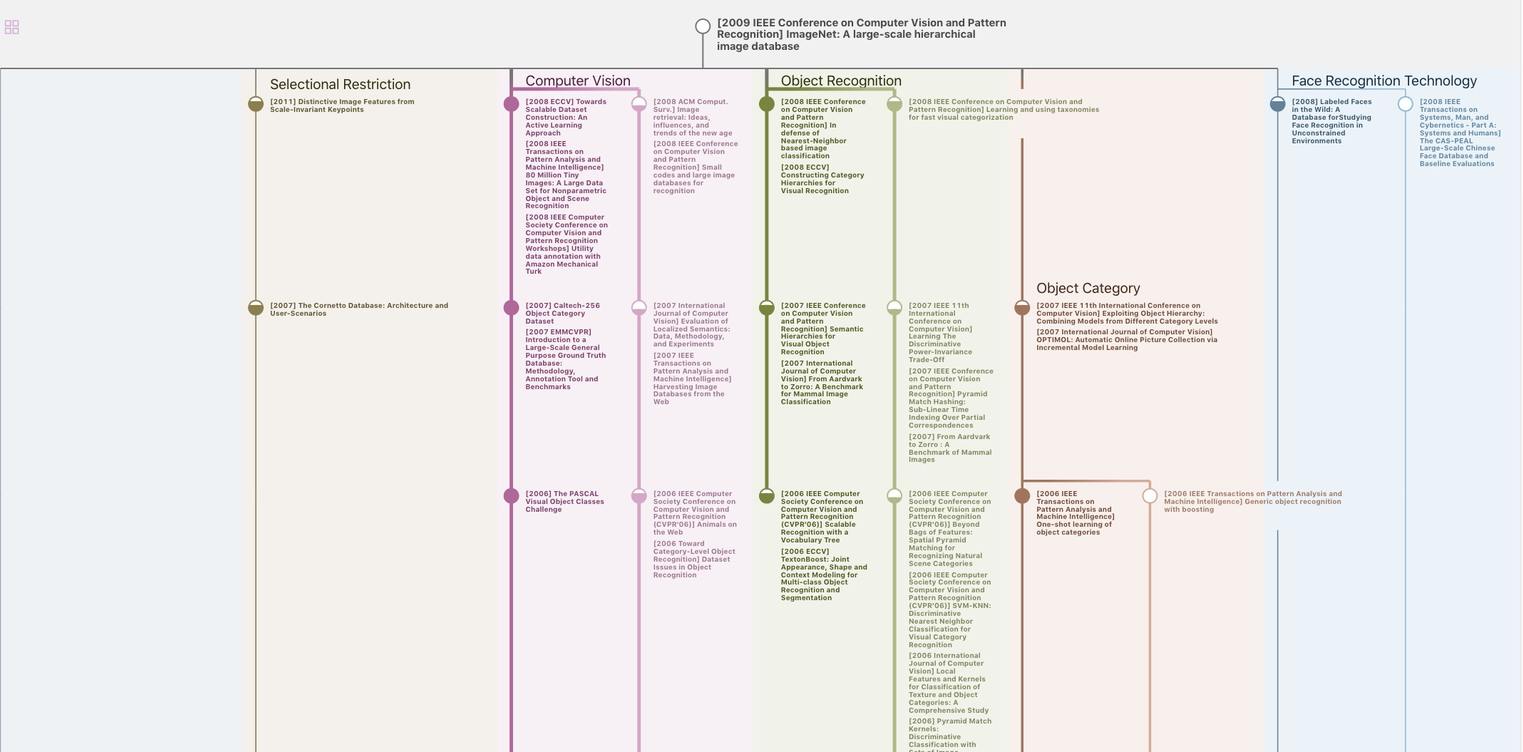
生成溯源树,研究论文发展脉络
Chat Paper
正在生成论文摘要