Mixed Effect Modeling and Variable Selection for Quantile Regression
arxiv(2021)
摘要
It is known that the estimating equations for quantile regression (QR) can be solved using an EM algorithm in which the M-step is computed via weighted least squares, with weights computed at the E-step as the expectation of independent generalized inverse-Gaussian variables. This fact is exploited here to extend QR to allow for random effects in the linear predictor. Convergence of the algorithm in this setting is established by showing that it is a generalized alternating minimization (GAM) procedure. Another modification of the EM algorithm also allows us to adapt a recently proposed method for variable selection in mean regression models to the QR setting. Simulations show the resulting method significantly outperforms variable selection in QR models using the lasso penalty. Applications to real data include a frailty QR analysis of hospital stays, and variable selection for age at onset of lung cancer and for riboflavin production rate using high-dimensional gene expression arrays for prediction.
更多查看译文
关键词
Expectation-maximization (EM) algorithm,Generalized alternating minimization (GAM) algorithm,high-dimensional estimation,mixture model,mixed effects regression,model diagnostics,variable selection
AI 理解论文
溯源树
样例
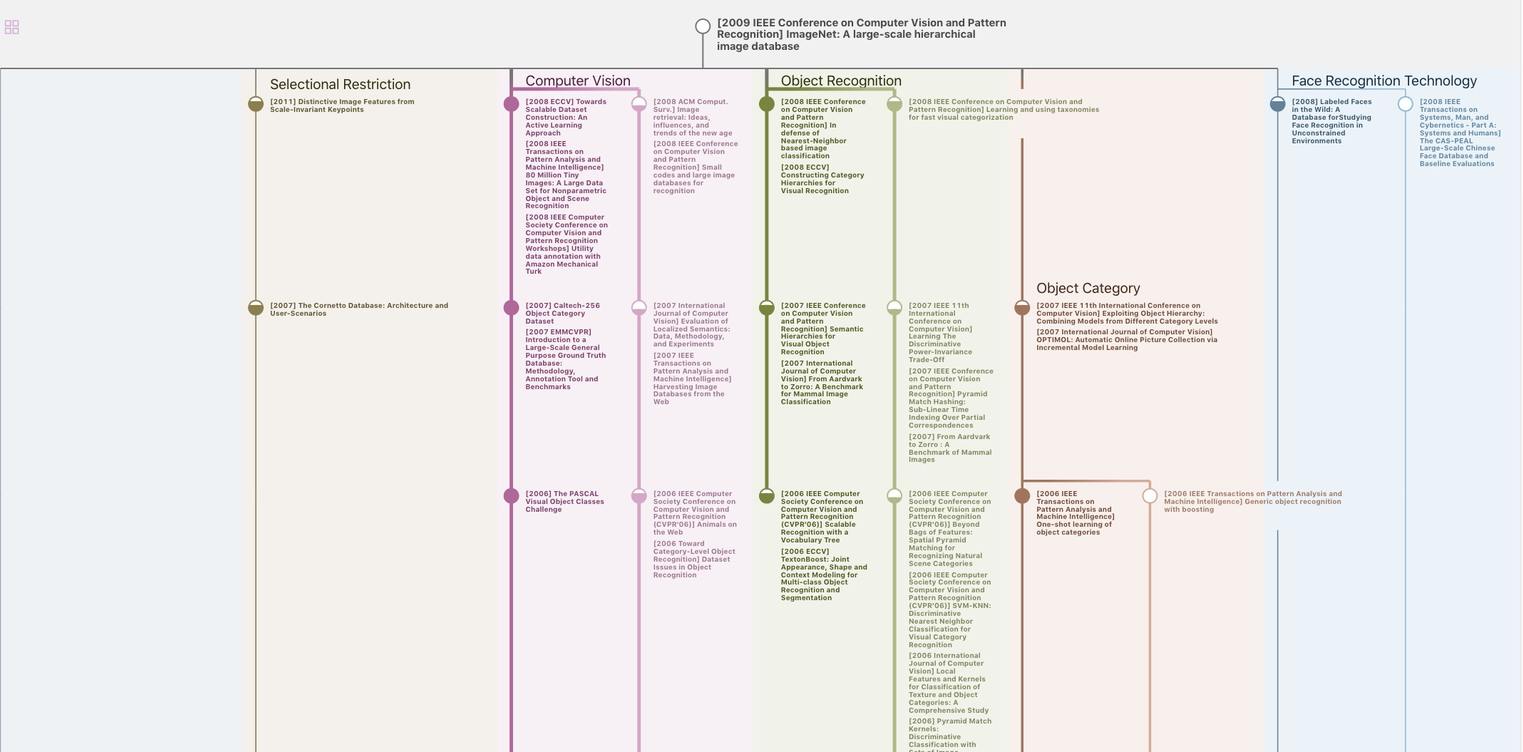
生成溯源树,研究论文发展脉络
Chat Paper
正在生成论文摘要