Learning to design drug-like molecules in three-dimensional space using deep generative models
arxiv(2021)
摘要
Recently, deep generative models for molecular graphs are gaining more and more attention in the field of de novo drug design. A variety of models have been developed to generate topological structures of drug-like molecules, but explorations in generating three-dimensional structures are still limited. Existing methods have either focused on low molecular weight compounds without considering drug-likeness or generate 3D structures indirectly using atom density maps. In this work, we introduce Ligand Neural Network (L-Net), a novel graph generative model for designing drug-like molecules with high-quality 3D structures. L-Net directly outputs the topological and 3D structure of molecules (including hydrogen atoms), without the need for additional atom placement or bond order inference algorithm. The architecture of L-Net is specifically optimized for drug-like molecules, and a set of metrics is assembled to comprehensively evaluate its performance. The results show that L-Net is capable of generating chemically correct, conformationally valid, and highly druglike molecules. Finally, to demonstrate its potential in structure-based molecular design, we combine L-Net with MCTS and test its ability to generate potential inhibitors targeting ABL1 kinase.
更多查看译文
关键词
deep generative models,molecules,drug-like,three-dimensional
AI 理解论文
溯源树
样例
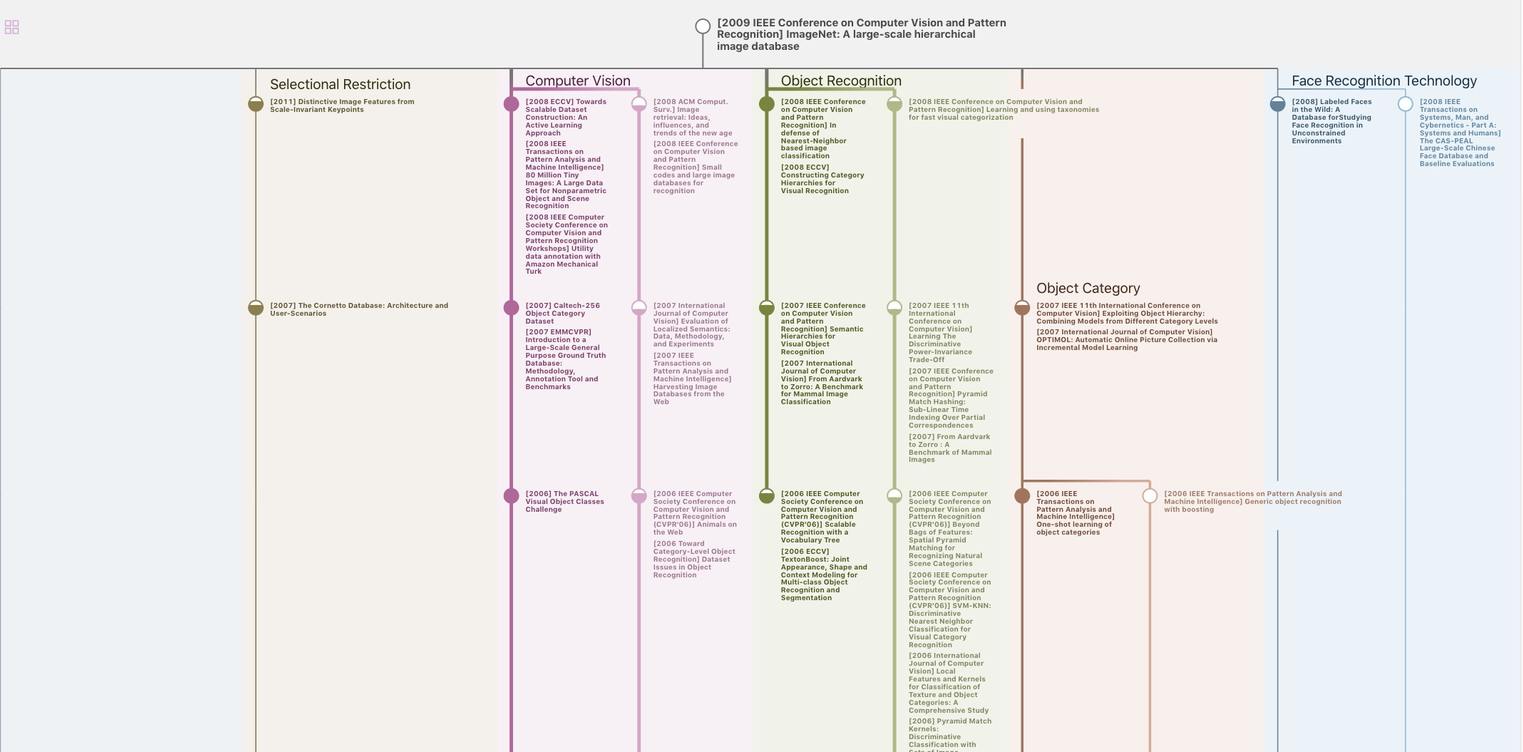
生成溯源树,研究论文发展脉络
Chat Paper
正在生成论文摘要