Real-time reconstruction of intense, ultrafast laser pulses using deep learning
arxiv(2021)
摘要
Ultrafast lasers ($< 500$ fs) have enabled laser-matter interactions at intensities exceeding $10^{18} \rm{Wcm}^{-2}$ with only millijoules of laser energy. However, as pulse durations become shorter, larger spectral bandwidths are required. Increasing the bandwidth causes the temporal structure to be increasingly sensitive to spectral phase, yet measuring the spectral phase of a laser pulse is nontrivial. While direct measurements of the spectral phase cannot be done using square-integrable detectors, phase information can be reconstructed by measuring the spectral response of a nonlinear optical effect. We introduce a new deep learning approach using the generalized nonlinear Schr\"{o}dinger equation and self-phase modulation, a $\chi_3$ nonlinearity occurring from material propagation. By training a neural network on numerical simulations of pulses propagating in a known material, the features of spectral change can be use to reconstruct the spectral phase. The technique is also sensitive to the local fluence of the pulse, enabling the full temporal intensity profile to be calculated. We demonstrate our method on a simulated large bandwidth pulse undergoing moderate material dispersion, and an experimentally produced broadband spectrum with substantial material dispersion. Error rates are low, even when modest amounts of noise introduced. With a single plate of glass and an optical spectrometer, single shot phase and fluence measurements are possible in real-time on intense ultrafast laser systems.
更多查看译文
AI 理解论文
溯源树
样例
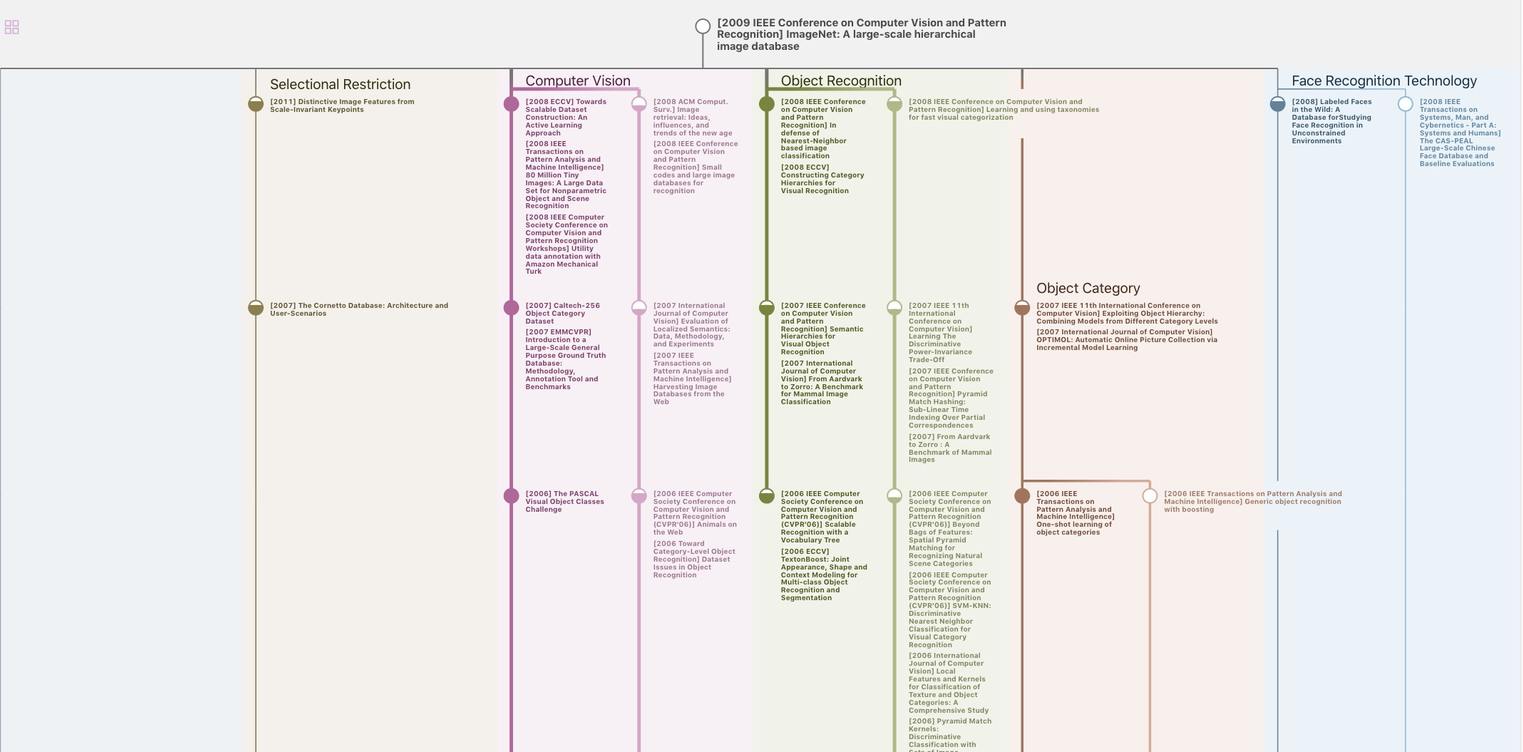
生成溯源树,研究论文发展脉络
Chat Paper
正在生成论文摘要