Adaptive Robust Model Predictive Control with Matched and Unmatched Uncertainty.
ACC(2022)
摘要
We propose a learning-based robust predictive control algorithm that can handle large uncertainty in the dynamics for a class of discrete-time systems that are nominally linear with an additive nonlinear dynamics component. Such systems commonly model the nonlinear effects of an unknown environment on a nominal system. Motivated by an inability of existing learning-based predictive control algorithms to achieve safety guarantees in the presence of uncertainties of large magnitude in this setting, we achieve significant performance improvements by optimizing over a novel class of nonlinear feedback policies inspired by certainty equivalent "estimate-and-cancel" control laws pioneered in classical adaptive control. In contrast with previous work in robust adaptive MPC, this allows us to take advantage of the structure in the a priori unknown dynamics that are learned online through function approximation. Our approach also extends typical nonlinear adaptive control methods to systems with state and input constraints even when an additive uncertain function cannot directly be canceled from the dynamics. Moreover, our approach allows us to apply contemporary statistical estimation techniques to certify the safety of the system through persistent constraint satisfaction with high probability. We show that our method allows us to consider larger unknown terms in the dynamics than existing methods through simulated examples.
更多查看译文
关键词
uncertainty,predictive,control
AI 理解论文
溯源树
样例
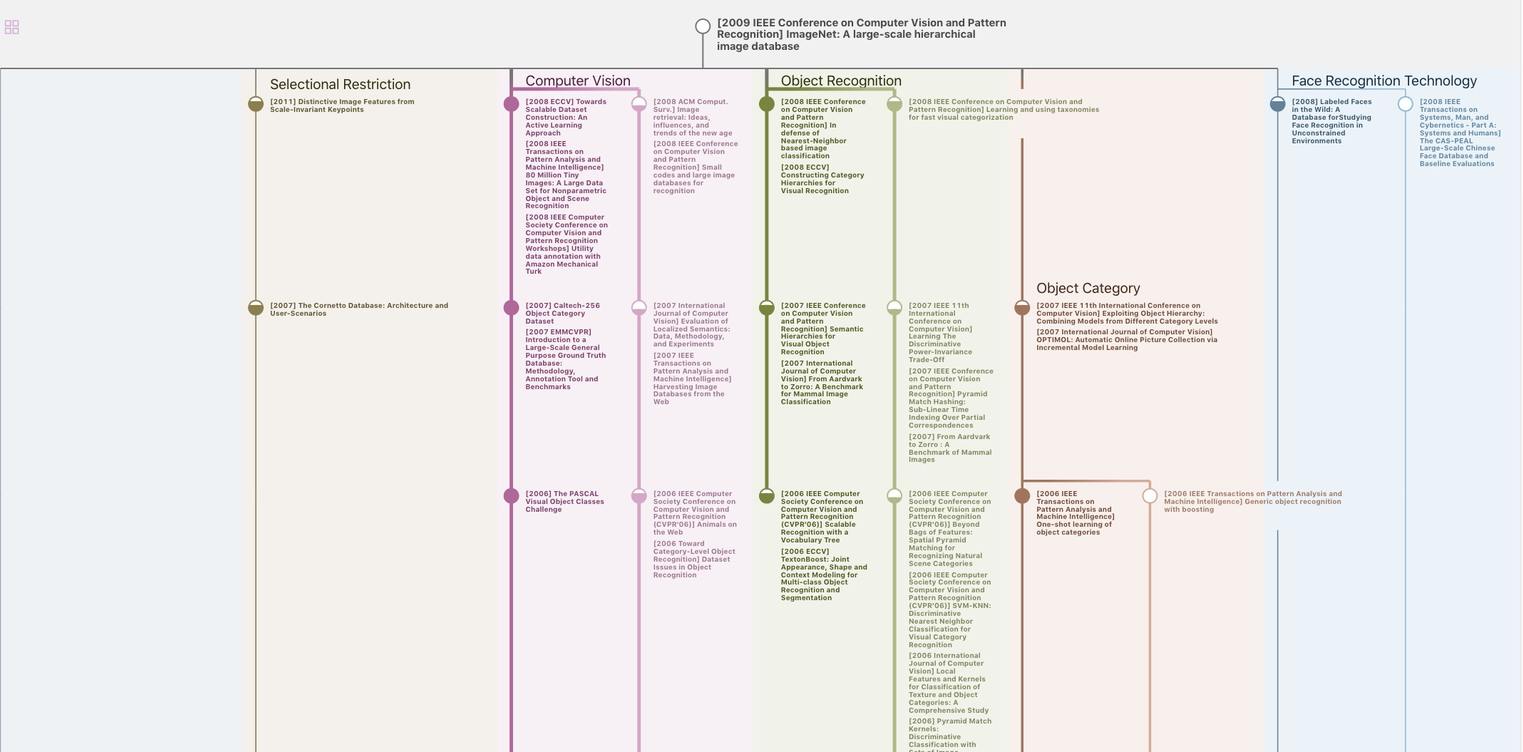
生成溯源树,研究论文发展脉络
Chat Paper
正在生成论文摘要