An information-based metric for observing strategy optimization, demonstrated in the context of photometric redshifts with applications to cosmology
arxiv(2021)
摘要
The observing strategy of a galaxy survey influences the degree to which its resulting data can be used to accomplish any science goal. LSST is thus seeking metrics of observing strategies for multiple science cases in order to optimally choose a cadence. Photometric redshifts are essential for many extragalactic science applications of LSST's data, including but not limited to cosmology, but there are few metrics available, and they are not straightforwardly integrated with metrics of other cadence-dependent quantities that may influence any given use case. We propose a metric for observing strategy optimization based on the potentially recoverable mutual information about redshift from a photometric sample under the constraints of a realistic observing strategy. We demonstrate a tractable estimation of a variational lower bound of this mutual information implemented in a public code using conditional normalizing flows. By comparing the recoverable redshift information across observing strategies, we can distinguish between those that preclude robust redshift constraints and those whose data will preserve more redshift information, to be generically utilized in a downstream analysis. We recommend the use of this versatile metric to observing strategy optimization for redshift-dependent extragalactic use cases, including but not limited to cosmology, as well as any other science applications for which photometry may be modeled from true parameter values beyond redshift.
更多查看译文
关键词
photometric redshifts,cosmology,strategy optimization,information-based
AI 理解论文
溯源树
样例
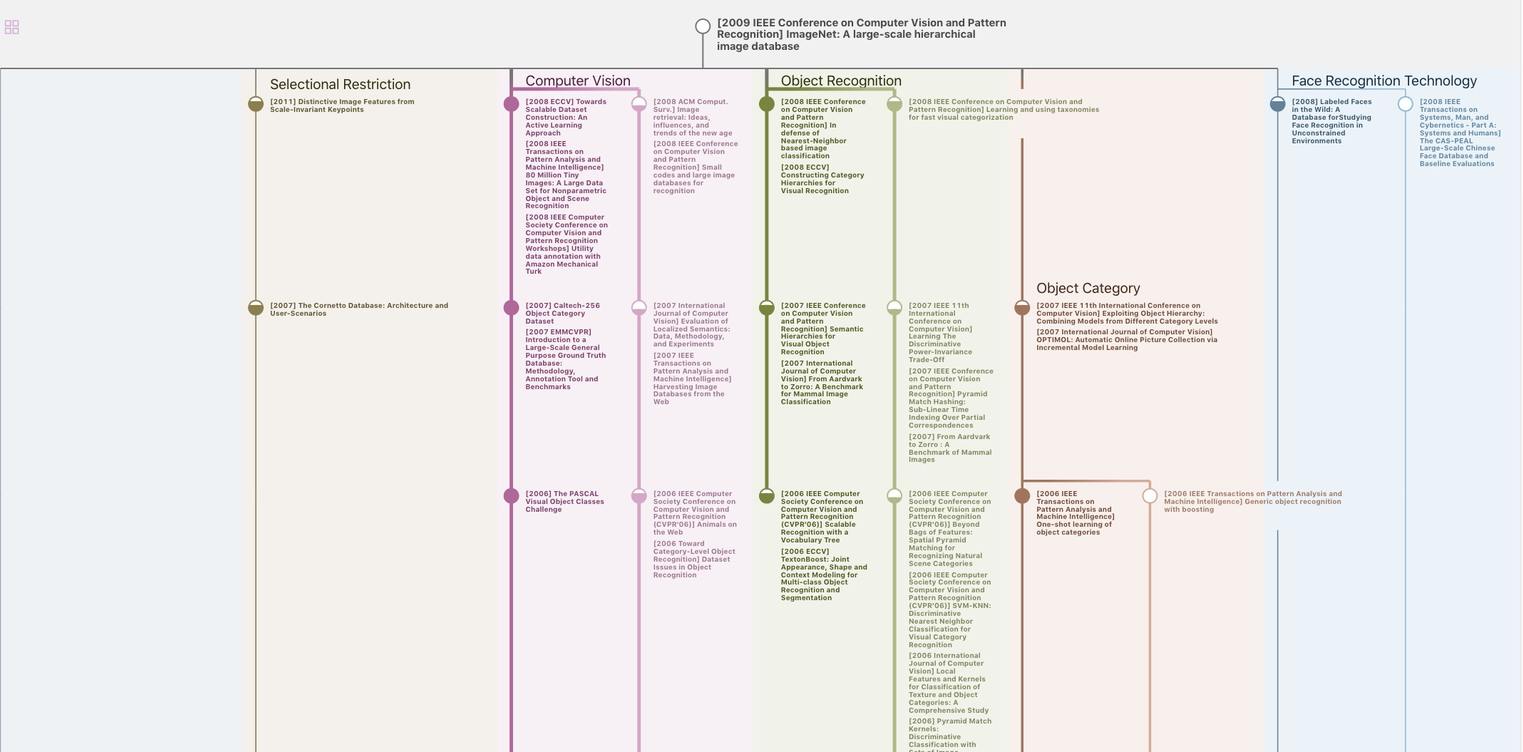
生成溯源树,研究论文发展脉络
Chat Paper
正在生成论文摘要