A Feature Selection Algorithm Performance Metric For Comparative Analysis
ALGORITHMS(2021)
摘要
This study presents a novel performance metric for feature selection algorithms that is unbiased and can be used for comparative analysis across feature selection problems. The baseline fitness improvement (BFI) measure quantifies the potential value gained by applying feature selection. The BFI measure can be used to compare the performance of feature selection algorithms across datasets by measuring the change in classifier performance as a result of feature selection, with respect to the baseline where all features are included. Empirical results are presented to show that there is performance complementarity for a suite of feature selection algorithms on a variety of real world datasets. The BFI measure is a normalised performance metric that can be used to correlate problem characteristics with feature selection algorithm performance, across multiple datasets. This ability paves the way towards describing the performance space of the per-instance algorithm selection problem for feature selection algorithms.
更多查看译文
关键词
feature selection, baseline fitness improvement, performance analysis
AI 理解论文
溯源树
样例
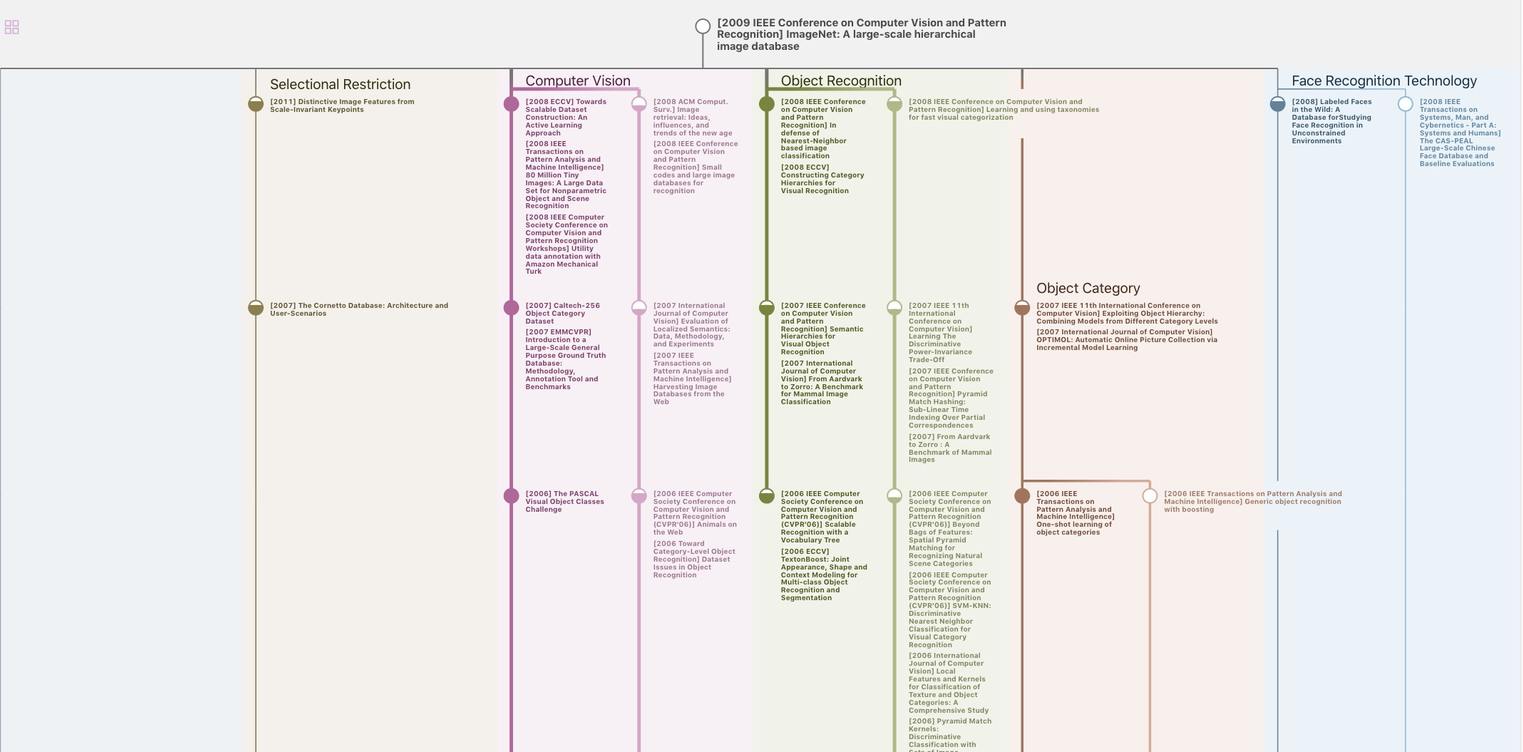
生成溯源树,研究论文发展脉络
Chat Paper
正在生成论文摘要