Noise-Aware Saliency Prediction for Videos with Incomplete Gaze Data
arxiv(2021)
摘要
Deep-learning-based algorithms have led to impressive results in visual-saliency prediction, but the impact of noise in training gaze data has been largely overlooked. This issue is especially relevant for videos, where the gaze data tends to be incomplete, and thus noisier, compared to images. Therefore, we propose a noise-aware training (NAT) paradigm for visual-saliency prediction that quantifies the uncertainty arising from gaze data incompleteness and inaccuracy, and accounts for it in training. We demonstrate the advantage of NAT independently of the adopted model architecture, loss function, or training dataset. Given its robustness to the noise in incomplete training datasets, NAT ushers in the possibility of designing gaze datasets with fewer human subjects. We also introduce the first dataset that offers a video-game context for video-saliency research, with rich temporal semantics, and multiple gaze attractors per frame.
更多查看译文
关键词
video,prediction,noise-aware
AI 理解论文
溯源树
样例
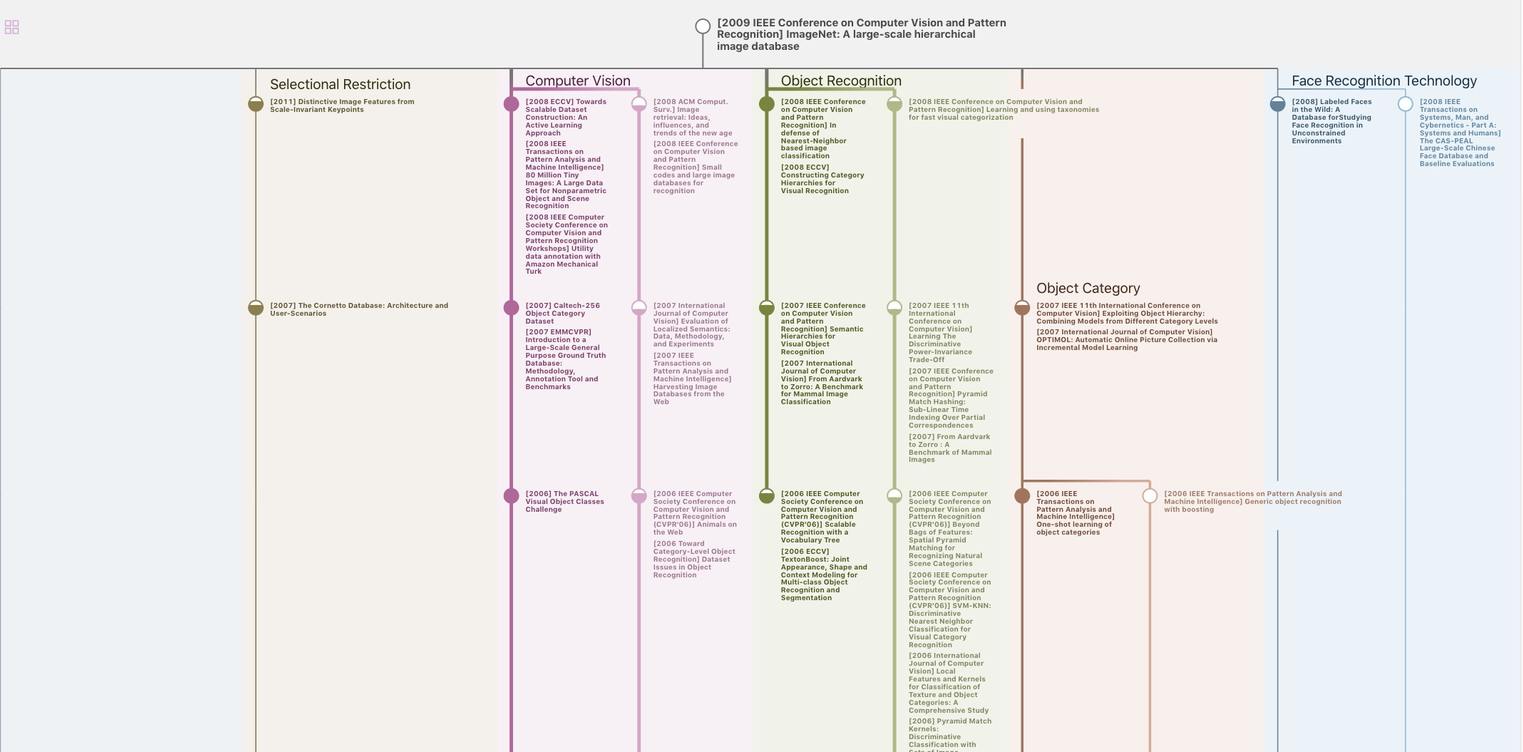
生成溯源树,研究论文发展脉络
Chat Paper
正在生成论文摘要