ZeRO-infinity: breaking the GPU memory wall for extreme scale deep learning
SC(2021)
摘要
ABSTRACTIn the last three years, the largest dense deep learning models have grown over 1000x to reach hundreds of billions of parameters, while the GPU memory has only grown by 5x (16 GB to 80 GB). Therefore, the growth in model scale has been supported primarily though system innovations that allow large models to fit in the aggregate GPU memory of multiple GPUs. However, we are getting close to the GPU memory wall. It requires 800 NVIDIA V100 GPUs just to fit a trillion parameter model for training, and such clusters are simply out of reach for most data scientists. In addition, training models at that scale requires complex combinations of parallelism techniques that puts a big burden on the data scientists to refactor their model. In this paper we present ZeRO-Infinity, a novel heterogeneous system technology that leverages GPU, CPU, and NVMe memory to allow for unprecedented model scale on limited resources without requiring model code refactoring. At the same time it achieves excellent training throughput and scalability, unencumbered by the limited CPU or NVMe bandwidth. ZeRO-Infinity can fit models with tens and even hundreds of trillions of parameters for training on current generation GPU clusters. It can be used to fine-tune trillion parameter models on a single NVIDIA DGX-2 node, making large models more accessible. In terms of training throughput and scalability, it sustains over 25 petaflops on 512 NVIDIA V100 GPUs (40% of peak), while also demonstrating super linear scalability. An open source implementation of ZeRO-Infinity is available through DeepSpeed 1.
更多查看译文
关键词
extreme scale deep learning,gpu memory wall,deep learning,zero-infinity
AI 理解论文
溯源树
样例
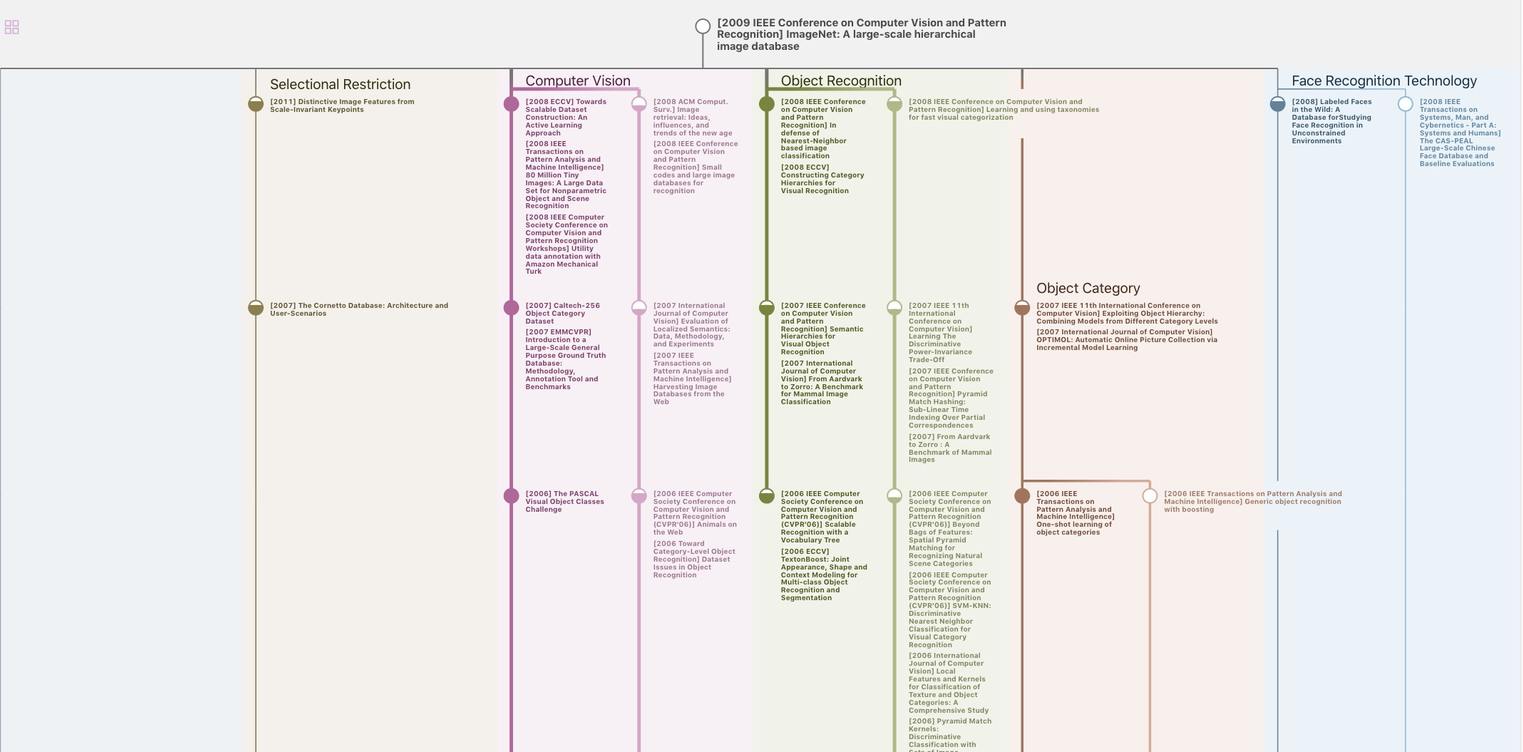
生成溯源树,研究论文发展脉络
Chat Paper
正在生成论文摘要