A random persistence diagram generator
Statistics and Computing(2022)
摘要
Topological data analysis (TDA) studies the shape patterns of data. Persistent homology is a widely used method in TDA that summarizes homological features of data at multiple scales and stores them in persistence diagrams (PDs). In this paper, we propose a random persistence diagram generator (RPDG) method that generates a sequence of random PDs from the ones produced by the data. RPDG is underpinned by a model based on pairwise interacting point processes and a reversible jump Markov chain Monte Carlo (RJ-MCMC) algorithm. A first example, which is based on a synthetic dataset, demonstrates the efficacy of RPDG and provides a comparison with another method for sampling PDs. A second example demonstrates the utility of RPDG to solve a materials science problem given a real dataset of small sample size.
更多查看译文
关键词
Interacting point processes, Topological data analysis, Reversible jump Markov chain Monte Carlo, Materials microstructure analysis
AI 理解论文
溯源树
样例
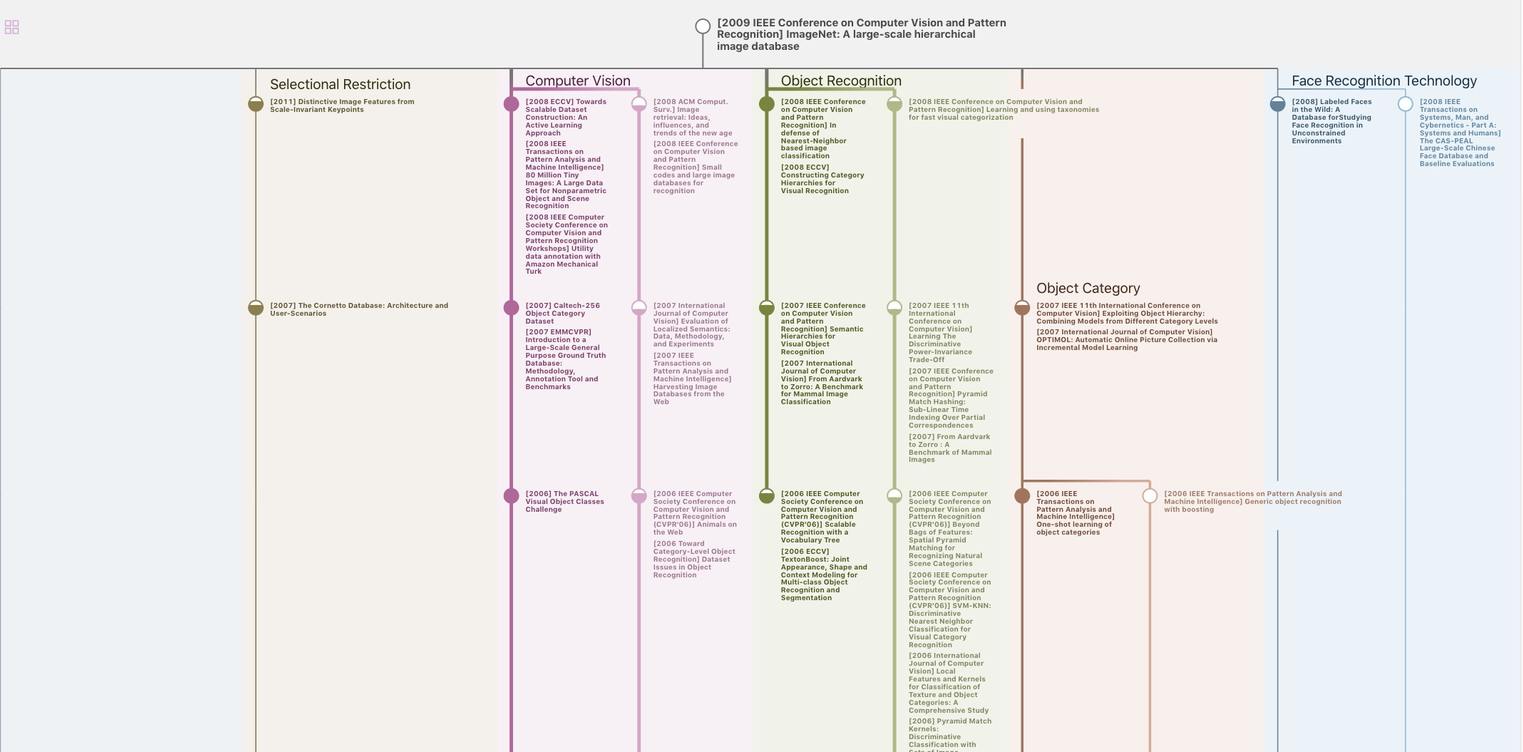
生成溯源树,研究论文发展脉络
Chat Paper
正在生成论文摘要