Development And Validation Of A Novel Nomogram For Individualized Prediction Of Survival In Cancer Of Unknown Primary
CLINICAL CANCER RESEARCH(2021)
摘要
Purpose: Prognostic uncertainty is a major challenge for cancer of unknown primary (CUP). Current models limit a meaningful patient-provider dialogue. We aimed to establish a nomogram for predicting overall survival (OS) in CUP based on robust dinicopathologic prognostic factors.Experimental Design: We evaluated 521 patients with CUP at MD Anderson Cancer Center (MDACC; Houston, TX; 2012-2016). Baseline variables were analyzed using Cox regression and nomogram developed using significant predictors. Predictive accuracy and discriminatory performance were assessed by calibration curves, concordance probability estimate (CPE +/- SE), and concordance statistic (C-index). The model was subjected to bootstrapping and multi-institutional external validations using two independent CUP cohorts: V1 [MDACC (2017), N = 103] and V2 (BC Cancer, Vancouver, Canada and Sarah Cannon Cancer Center/Tennessee Oncology, Nashville, TN; N = 302).Results: Baseline characteristics of entire cohort (N = 926) included: median age (63 years), women (51%), Eastern Cooperative Oncology Group performance status (ECOG PS) 0-1 (64%), adenocarcinomas (52%), >= sites of metastases (30%), and median follow-up duration and OS of 40.1 and 14.7 months, respectively. Five independent prognostic factors were identified: gender, ECOG PS, histology, number of metastatic sites, and neutrophil-lymphocyte ratio. The resulting model predicted OS with CPE of 0.69 [SE: +/- 0.01; C-index: 0.71 (95% confidence interval: 0.68-0.74)] outperforming Culine/Seve prognostic models (CPE: 0.59 +/- 0.01). CPE for external validation cohorts Vi and V2 were 0.67 (1 0.02) and 0.70 (+/- 0.01), respectively. Calibration curves for 1-year OS showed strong agreement between nomogram prediction and actual observations in all cohorts.Conclusions: Our user-friendly CUP nomogram integrating commonly available baseline factors provides robust personalized prognostication which can aid clinical decision making and selection/stratification for clinical trials.
更多查看译文
AI 理解论文
溯源树
样例
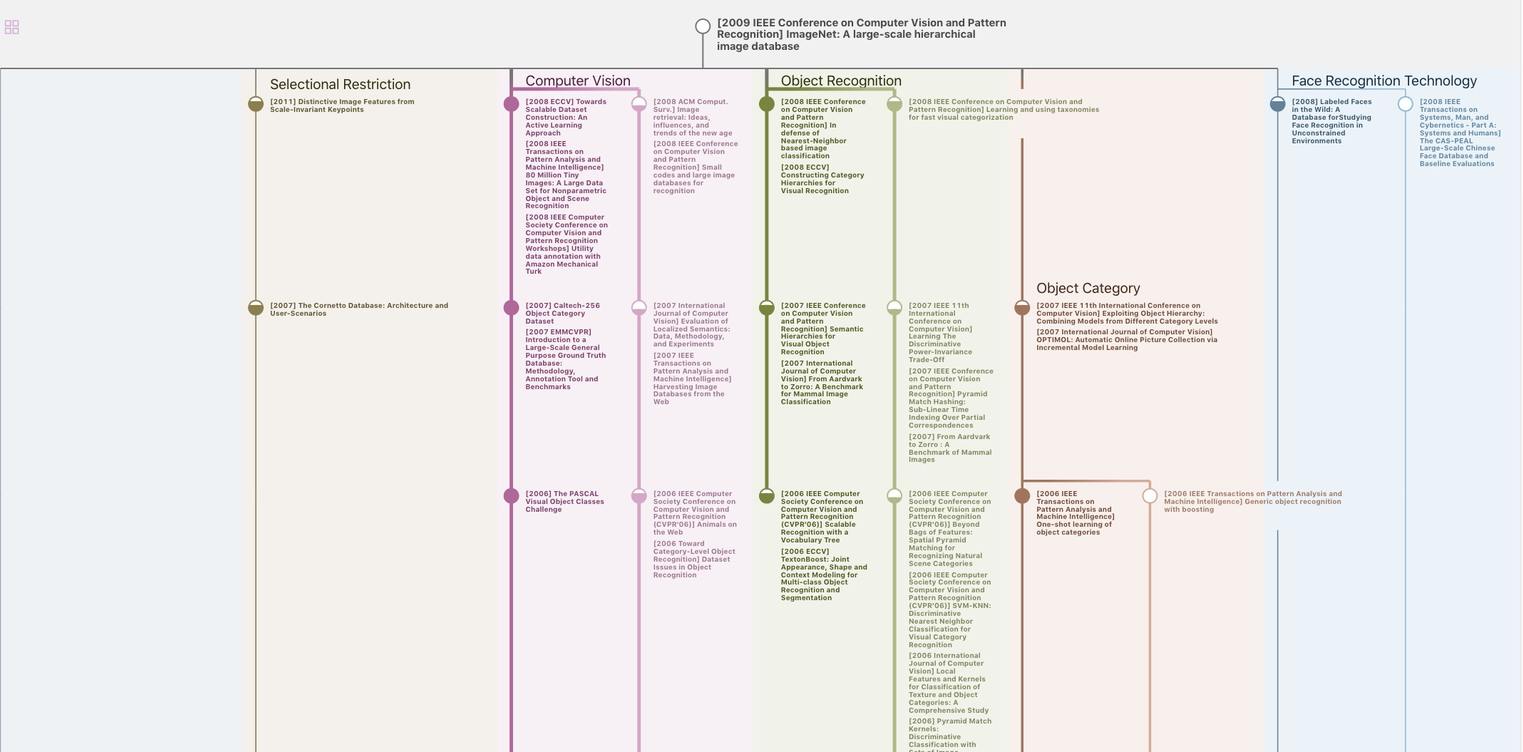
生成溯源树,研究论文发展脉络
Chat Paper
正在生成论文摘要