Chaotic Compressive Spectrum Sensing Based On Chebyshev Map For Cognitive Radio Networks
SYMMETRY-BASEL(2021)
摘要
Recently, the chaotic compressive sensing paradigm has been widely used in many areas, due to its ability to reduce data acquisition time with high security. For cognitive radio networks (CRNs), this mechanism aims at detecting the spectrum holes based on few measurements taken from the original sparse signal. To ensure a high performance of the acquisition and recovery process, the choice of a suitable sensing matrix and the appropriate recovery algorithm should be done carefully. In this paper, a new chaotic compressive spectrum sensing (CSS) solution is proposed for cooperative CRNs based on the Chebyshev sensing matrix and the Bayesian recovery via Laplace prior. The chaotic sensing matrix is used first to acquire and compress the high-dimensional signal, which can be an interesting topic to be published in symmetry journal, especially in the data-compression subsection. Moreover, this type of matrix provides reliable and secure spectrum detection as opposed to random sensing matrix, since any small change in the initial parameters generates a different sensing matrix. For the recovery process, unlike the convex and greedy algorithms, Bayesian models are fast, require less measurement, and deal with uncertainty. Numerical simulations prove that the proposed combination is highly efficient, since the Bayesian algorithm with the Chebyshev sensing matrix provides superior performances, with compressive measurements. Technically, this number can be reduced to 20% of the length and still provides a substantial performance.
更多查看译文
关键词
compressive sensing, chaotic sensing matrices, Chebyshev map, Bayesian models, spectrum sensing, cognitive radio networks, cooperative networks
AI 理解论文
溯源树
样例
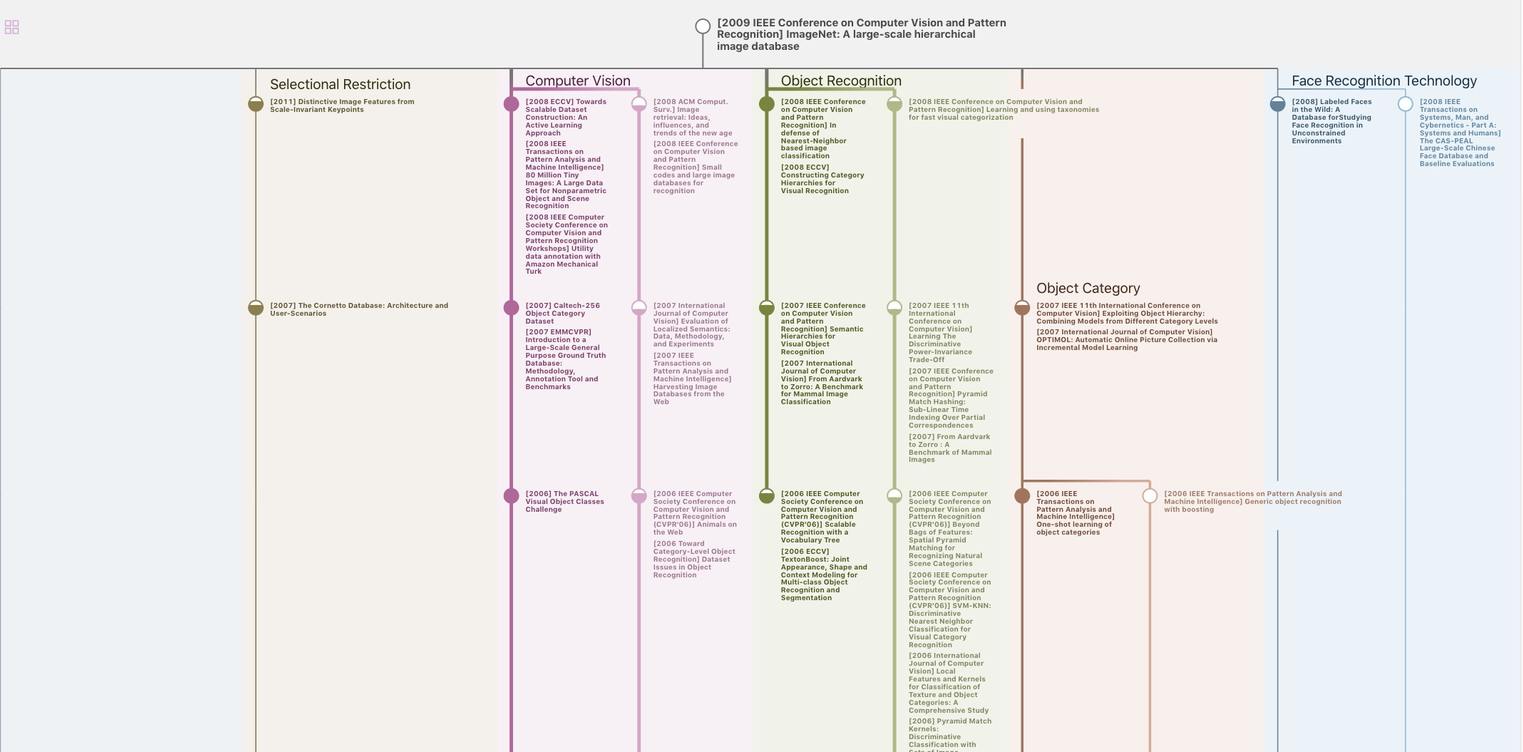
生成溯源树,研究论文发展脉络
Chat Paper
正在生成论文摘要