Inverse probability of censoring weighting for visual predictive checks of time-to-event models with time-varying covariates
PHARMACEUTICAL STATISTICS(2021)
摘要
When constructing models to summarize clinical data to be used for simulations, it is good practice to evaluate the models for their capacity to reproduce the data. This can be done by means of Visual Predictive Checks (VPC), which consist of several reproductions of the original study by simulation from the model under evaluation, calculating estimates of interest for each simulated study and comparing the distribution of those estimates with the estimate from the original study. This procedure is a generic method that is straightforward to apply, in general. Here we consider the application of the method to time-to-event data and consider the special case when a time-varying covariate is not known or cannot be approximated after event time. In this case, simulations cannot be conducted beyond the end of the follow-up time (event or censoring time) in the original study. Thus, the simulations must be censored at the end of the follow-up time. Since this censoring is not random, the standard KM estimates from the simulated studies and the resulting VPC will be biased. We propose to use inverse probability of censoring weighting (IPoC) method to correct the KM estimator for the simulated studies and obtain unbiased VPCs. For analyzing the Cantos study, the IPoC weighting as described here proved valuable and enabled the generation of VPCs to qualify PKPD models for simulations. Here, we use a generated data set, which allows illustration of the different situations and evaluation against the known truth.
更多查看译文
关键词
inverse probability weighting, model diagnostics, time‐, to‐, event models, time‐, varying covariates, visual predictive check
AI 理解论文
溯源树
样例
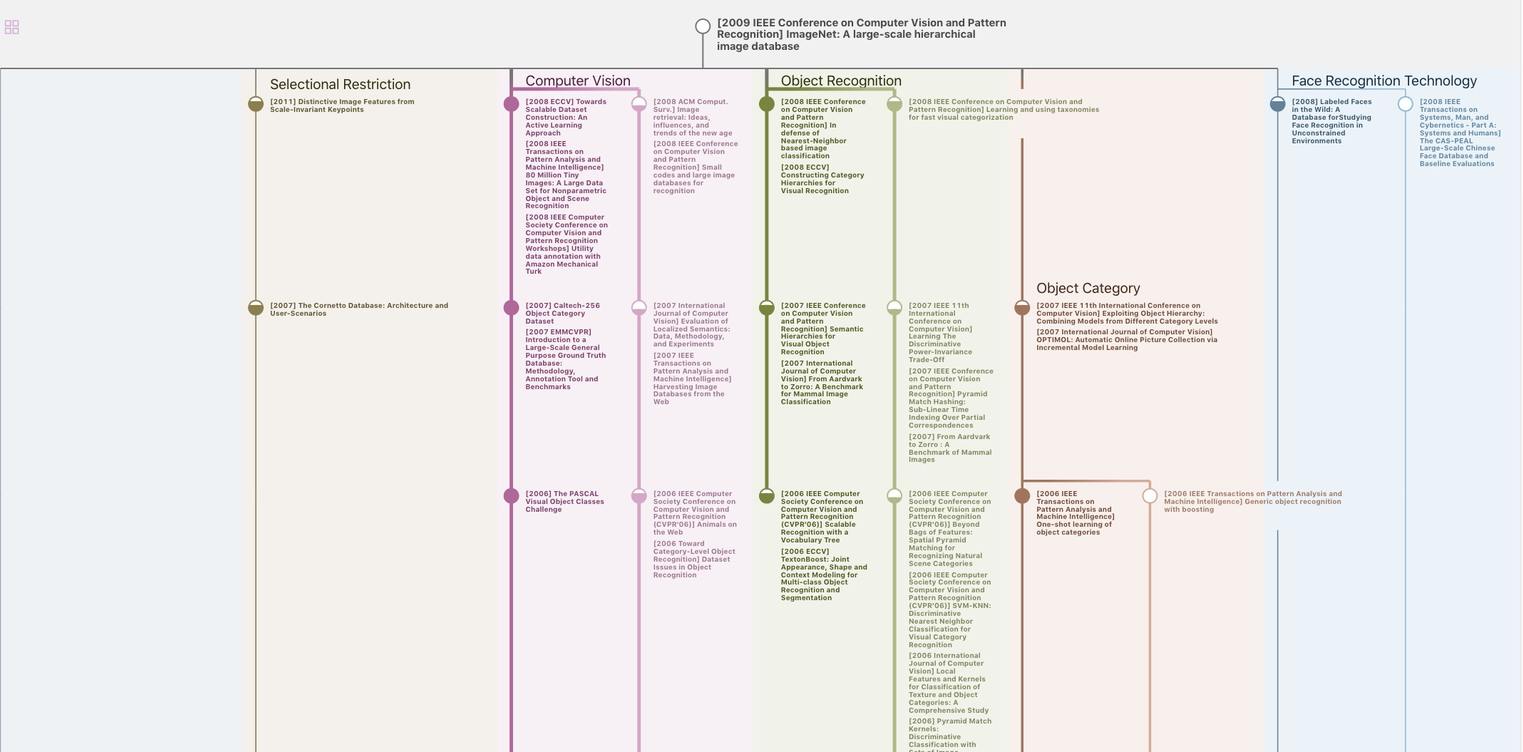
生成溯源树,研究论文发展脉络
Chat Paper
正在生成论文摘要