VolPy: Automated and scalable analysis pipelines for voltage imaging datasets
PLoS computational biology(2021)
摘要
Author summary Roughly 290 million electrical action potentials occur every second in the human brain, facilitating the propagation of signals among cells in the nervous system and driving most of our daily operations. New methods in brain imaging are emerging that have the speed and resolution to capture events in the brain at the pace at which neurons typically communicate. These methods measure voltage in neurons by using light, and therefore can access very detailed brain signaling patterns. However, the adoption of these methods by a larger community, and not a restricted set of experts, is limited by the lack of computational tools, thereby greatly hindering progress in this field. In this paper, we present VolPy, a software framework that greatly facilitates the preprocessing of this new type of imaging datasets. This pipeline incorporates efficient and optimized algorithms that can identify neurons and extract their activity with great accuracy. The presented software will make this new imaging modality accessible to a wide audience. Voltage imaging enables monitoring neural activity at sub-millisecond and sub-cellular scale, unlocking the study of subthreshold activity, synchrony, and network dynamics with unprecedented spatio-temporal resolution. However, high data rates (>800MB/s) and low signal-to-noise ratios create bottlenecks for analyzing such datasets. Here we present VolPy, an automated and scalable pipeline to pre-process voltage imaging datasets. VolPy features motion correction, memory mapping, automated segmentation, denoising and spike extraction, all built on a highly parallelizable, modular, and extensible framework optimized for memory and speed. To aid automated segmentation, we introduce a corpus of 24 manually annotated datasets from different preparations, brain areas and voltage indicators. We benchmark VolPy against ground truth segmentation, simulations and electrophysiology recordings, and we compare its performance with existing algorithms in detecting spikes. Our results indicate that VolPy's performance in spike extraction and scalability are state-of-the-art.
更多查看译文
AI 理解论文
溯源树
样例
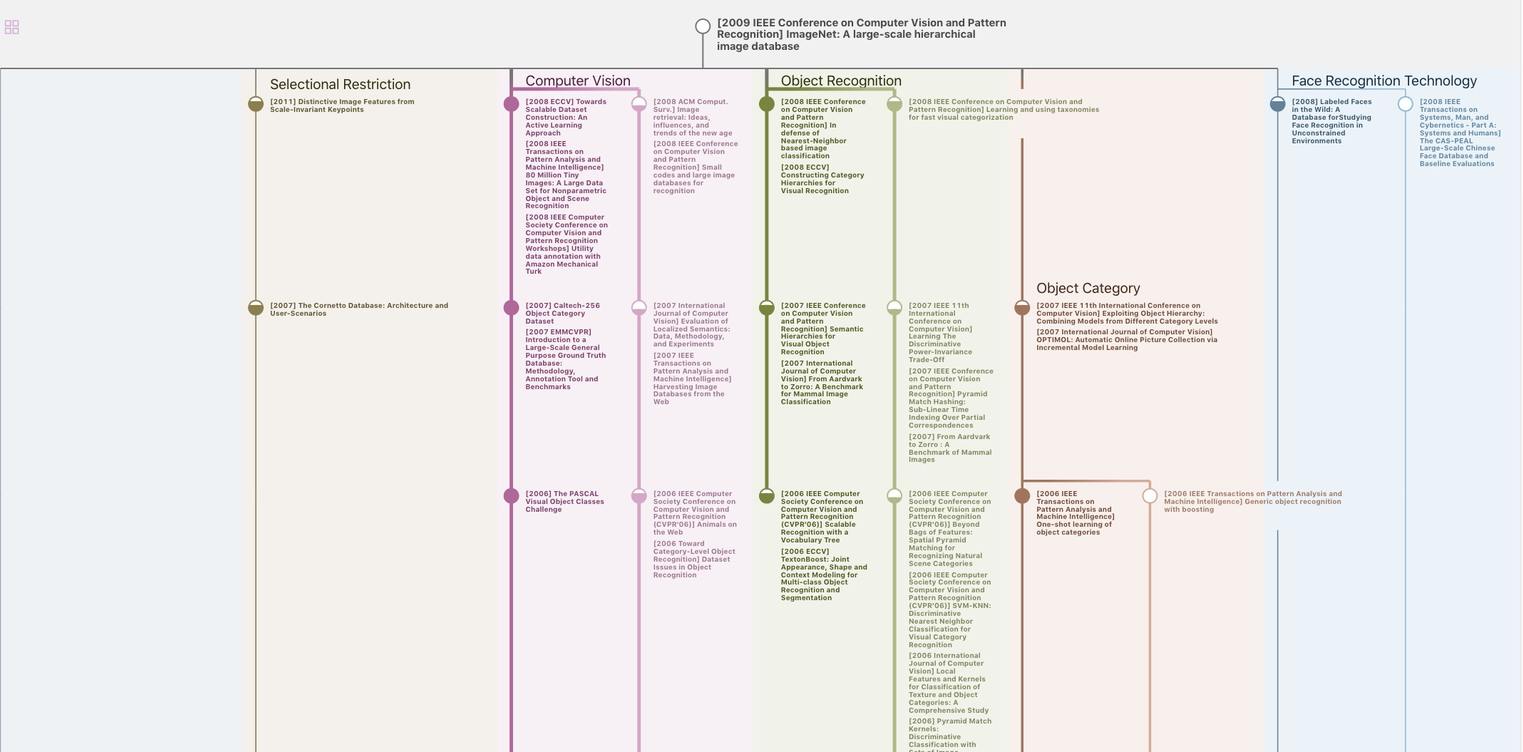
生成溯源树,研究论文发展脉络
Chat Paper
正在生成论文摘要